A Self-enhanced Automatic Traceability Link Recovery via Structure Knowledge Mining for Small-scale Labeled Data
2021 IEEE 45TH ANNUAL COMPUTERS, SOFTWARE, AND APPLICATIONS CONFERENCE (COMPSAC 2021)(2021)
摘要
Traceability links between requirements and source code are beneficial to the maintenance and evolution activities. Compared with the proposed unsupervised solutions, supervised solutions are more effective to generate trace links automatically and gaining more attention. However, supervised solutions often need to spend a lot of effort on labeling data. To overcome this limitation, we propose a self-enhanced automatic traceability link recovery approach based on structure knowledge mining for small-scale labeled data, named K2Trace, which not only enhances the semantic representations of artifacts by mining context information but also self-enhances the size of training set by exploring transitive relationships. Evaluation results show that K2Trace can outperform the state-of-the-art baseline approach. K2Trace proves the usefulness of mining knowledge from the structure information of software artifacts, as well as provides a new way to substantially reduce the amount of training data needed for training efficient classification models, which may pave the way for generating accurate trace links.
更多查看译文
关键词
traceability link recovery, context information, context embedding, transitive relationship, inference rule
AI 理解论文
溯源树
样例
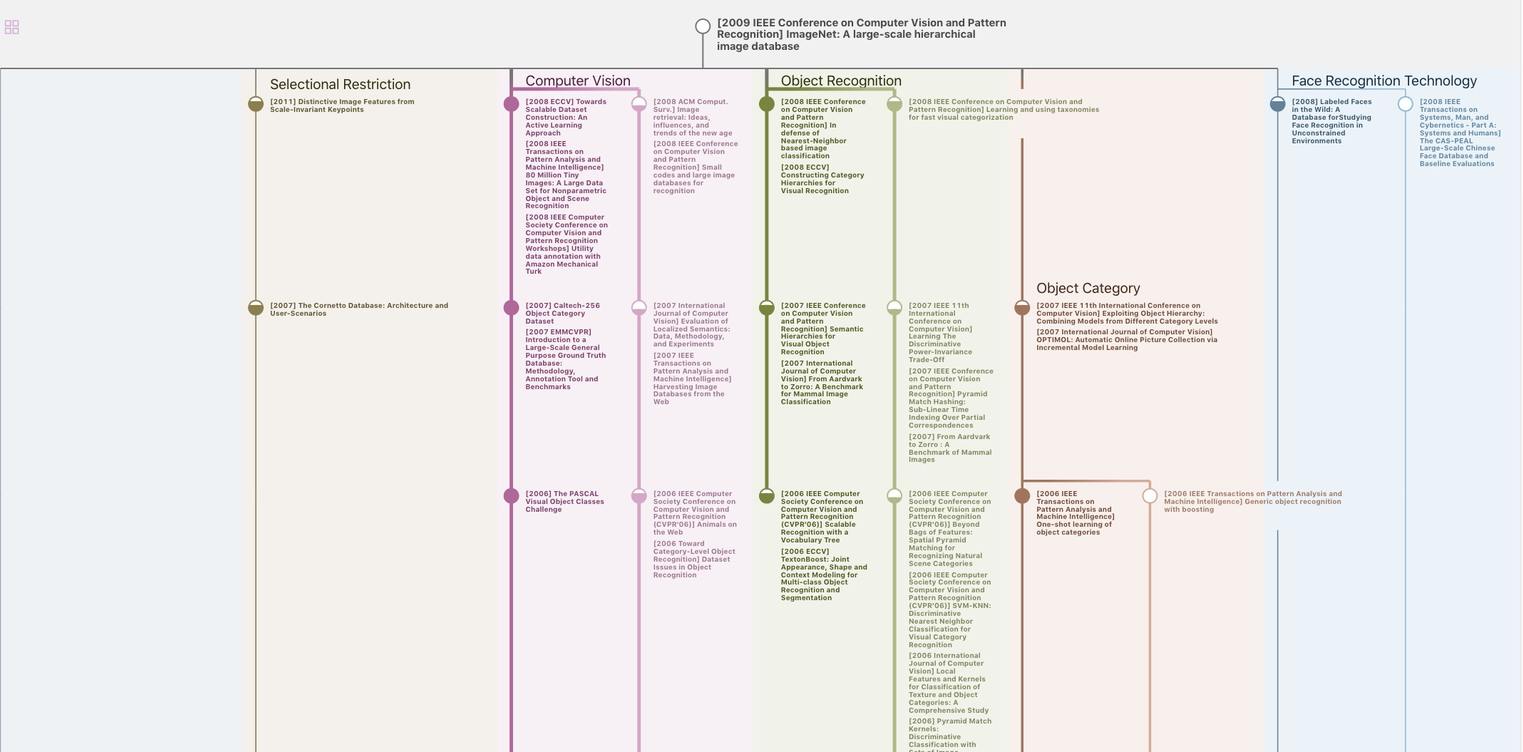
生成溯源树,研究论文发展脉络
Chat Paper
正在生成论文摘要