Deep Convolutional Neural Network Transfer Learning Optimization Based on Visual Interpretation
RCAR(2021)
摘要
In image classification tasks, the training of deep convolutional neural networks generally requires a large amount of data, and due to the constraints of environment, resources and time, it is of great practical importance to use fewer training samples to obtain a higher recognition rate in the shortest possible time. A deep convolutional neural network transfer learning optimization method based on visual interpretation is proposed for a specific image classification task. Firstly, we use class activation mapping visualization as a visual interpretation, output the class activation heat map of the validation set images, and analyze the reasons for misrecognition of the images. Secondly, we introduce “feedback” by pre-recognizing and visualizing the optimized dataset with the model trained on the original dataset, selecting the images that have a greater impact on improving the recognition rate, and maximizing the impact of the optimized images on the original model. Finally, the model is retrained on the optimized training set. The experimental results show that this method can effectively improve the recognition rate of the transfer learning model for image classification.
更多查看译文
关键词
visual interpretation,image classification tasks,fewer training samples,class activation mapping visualization,class activation heat map,optimized dataset,optimized images,optimized training set,transfer learning model,deep convolutional neural network transfer learning optimization,higher recognition rate,validation set images,images misrecognition
AI 理解论文
溯源树
样例
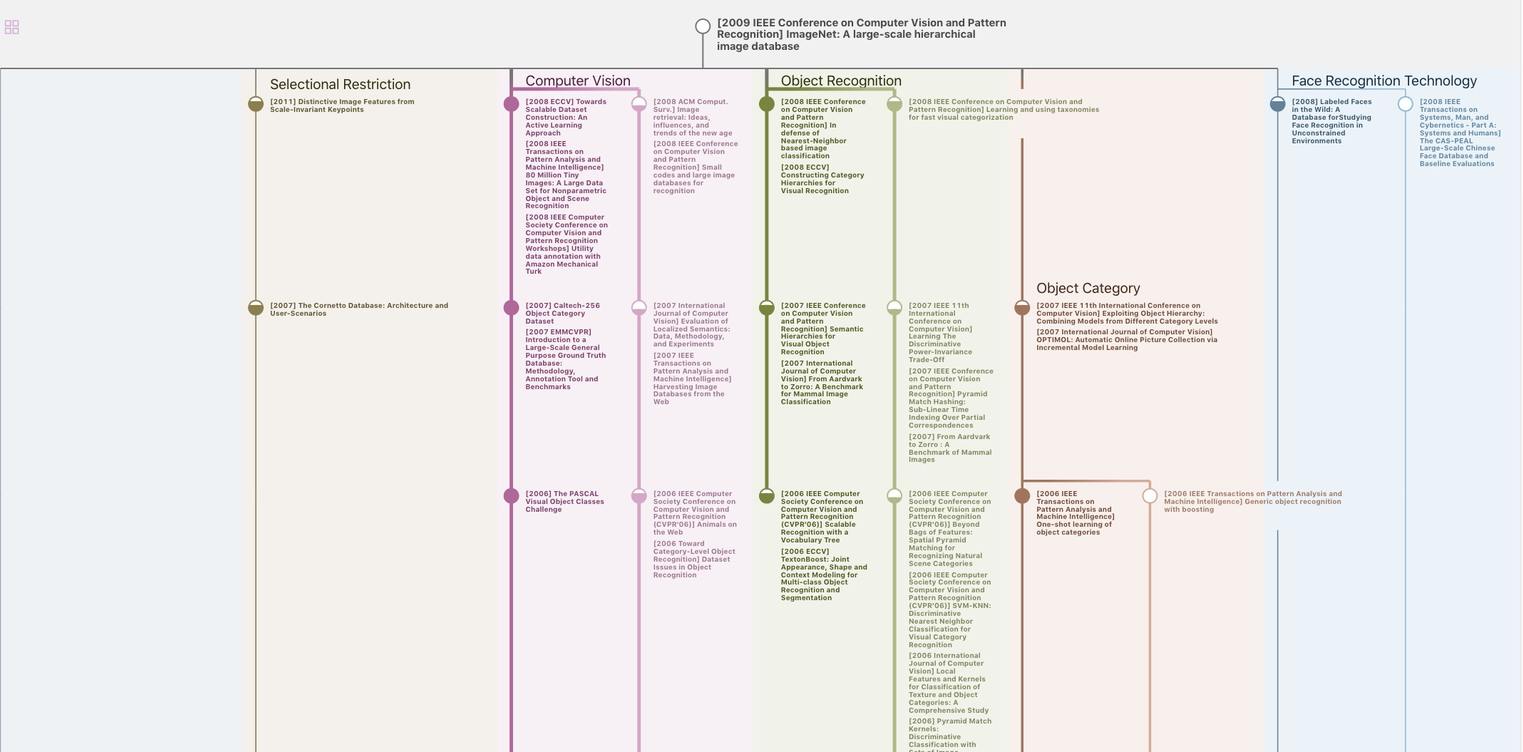
生成溯源树,研究论文发展脉络
Chat Paper
正在生成论文摘要