A Depthwise Separable Convolution Based 6D Pose Estimation Network by Efficient 2D-3D Feature Fusion.
RCAR(2021)
摘要
Precise 6D pose estimation of the target object is an essential prerequisite for robots to understand the real world. Previous 6D pose estimation methods based on 3D data usually have problems such as long model training time, imperfect feature extraction, redundant network model parameters, and complicated follow-up processing steps. This paper proposes a 2D-3D feature fusion module that could enhance feature extraction for the 6D pose estimation network. Furthermore, we compress the size of model parameters by adopting depthwise separable convolution to accelerate training speed and to reduce memory consumption. The experiment results on LineMOD dataset show the effectiveness of our method. Our method achieves on par or better performance than the state-of-art methods for 6D pose estimation and reduces model training time and the number of model parameters simultaneously.
更多查看译文
关键词
depthwise separable convolution,6D pose estimation network,Efficient 2D-3D Feature Fusion,precise 6D pose estimation,estimation methods,long model training time,imperfect feature extraction,redundant network model parameters,2D-3D feature fusion module
AI 理解论文
溯源树
样例
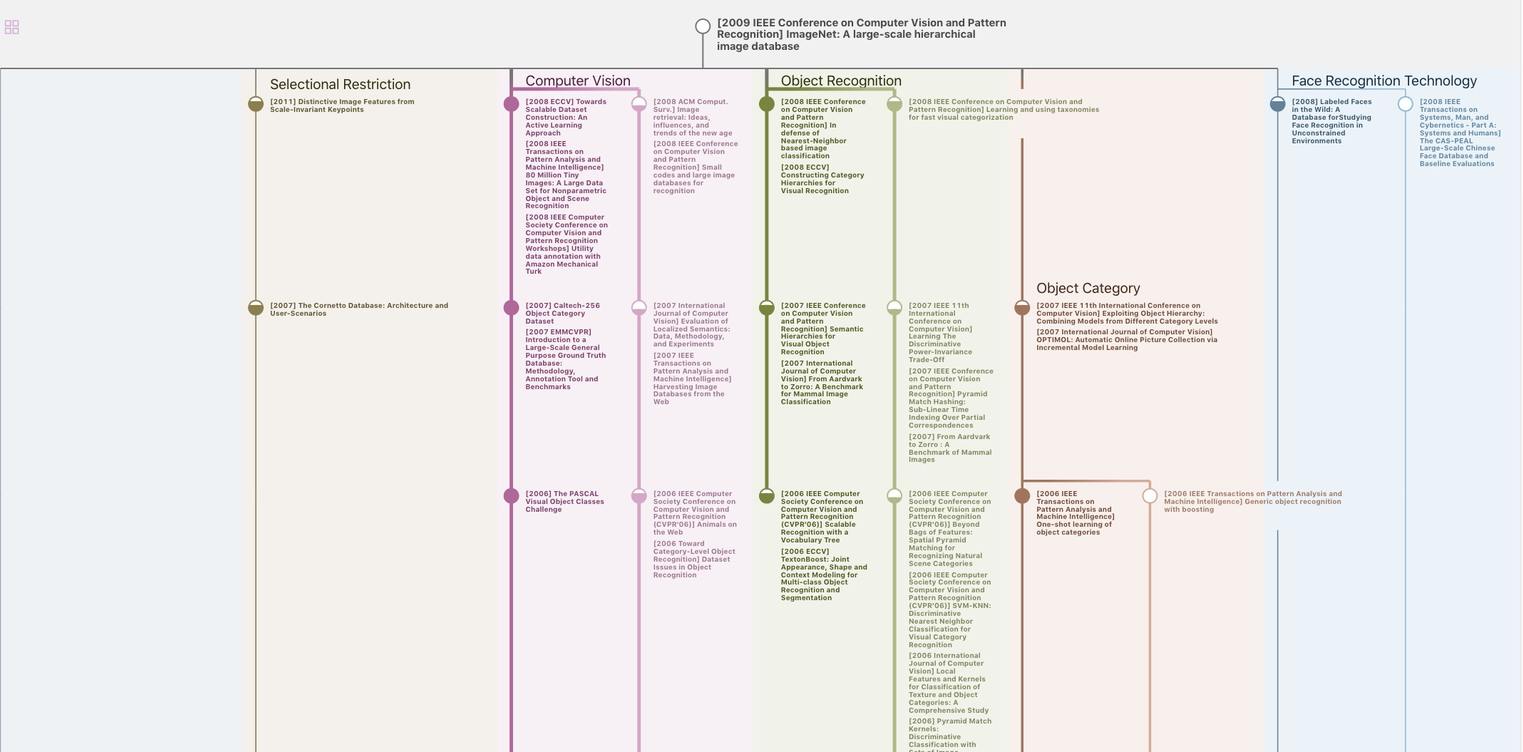
生成溯源树,研究论文发展脉络
Chat Paper
正在生成论文摘要