Learning Unsupervised Parameter-Specific Affine Transformation for Medical Images Registration
MEDICAL IMAGE COMPUTING AND COMPUTER ASSISTED INTERVENTION - MICCAI 2021, PT IV(2021)
摘要
Affine registration has recently been formulated using deep learning frameworks to establish spatial correspondences between different images. In this work, we propose a new unsupervised model that investigates two new strategies to tackle fundamental problems related to affine registration. More specifically, the new model 1) has the advantage to explicitly learn specific geometric transformation parameters (e.g. translations, rotation, scaling and shearing); and 2) can effectively understand the context between the images via cross-stitch units allowing feature exchange. The proposed model is evaluated on two two-dimensional X-ray datasets and a three-dimensional CT dataset. Our experimental results show that our model not only outperforms state-of-art approaches and also can predict specific transformation parameters.
更多查看译文
关键词
medical images registration,medical images,transformation,parameter-specific
AI 理解论文
溯源树
样例
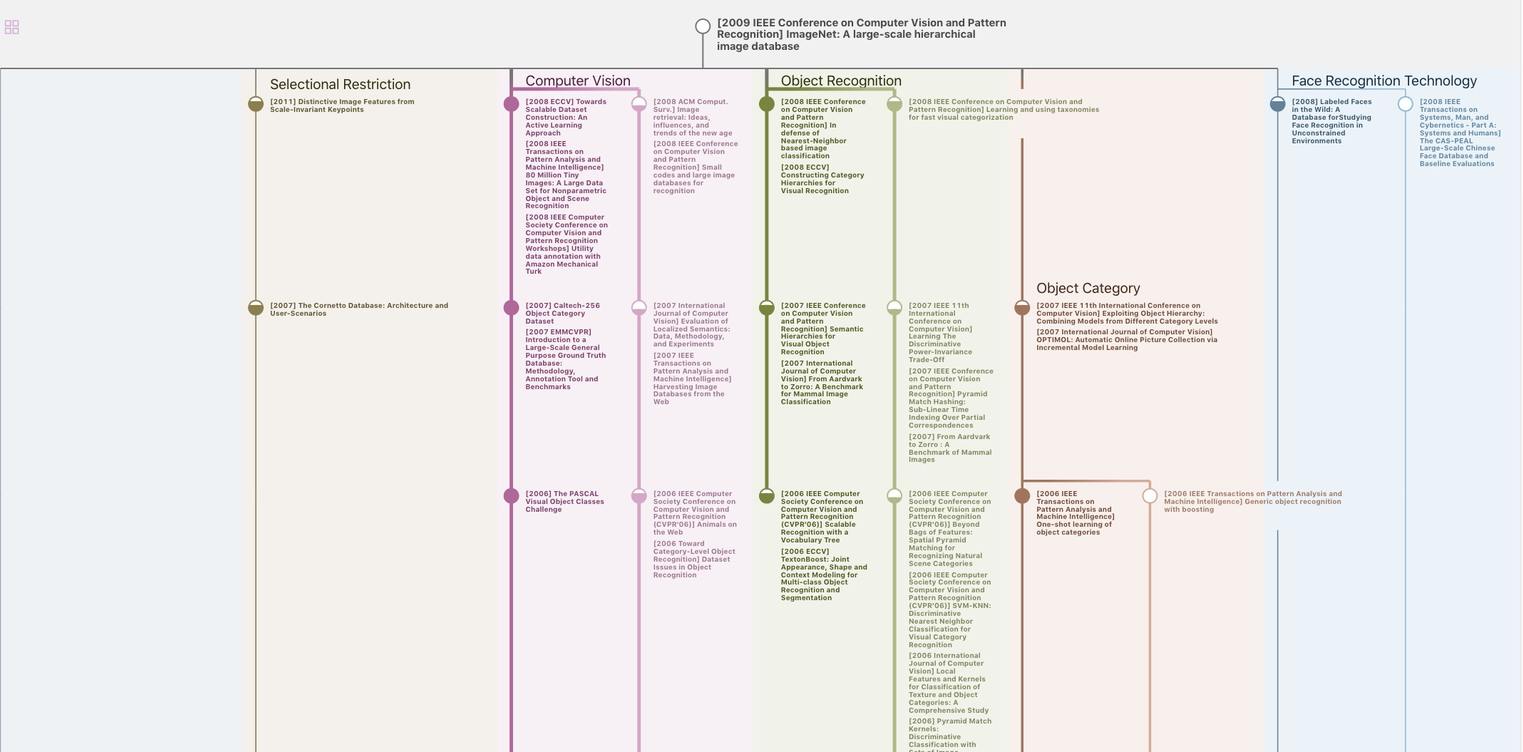
生成溯源树,研究论文发展脉络
Chat Paper
正在生成论文摘要