On the Quality of Compositional Prediction for Prospective Analytics on Graphs
DATABASE AND EXPERT SYSTEMS APPLICATIONS - DEXA 2021 WORKSHOPS(2021)
摘要
Recently, micro-learning has been successfully applied to various scenarios, such as graph optimization (e.g. power grid management). In these approaches, ad-hoc models of local data are built instead of one large model on the overall data set. Micro-learning is typically useful for incremental, what-if/prospective scenarios, where one has to perform step-by-step decisions based on local properties. A common feature of these applications is that the predicted properties (such as speed of a bus line) are compositions of smaller parts (e.g. the speed on each bus inter-stations along the line). But little is known about the quality of such predictions when generalized at a larger scale. In this paper we propose a generic technique that embeds machine-learning for graph-based compositional prediction, that allows 1) the prediction of the behaviour of composite objects, based on the predictions of their sub-parts and appropriate composition rules, and 2) the production of rich prospective analytics scenarios, where new objects never observed before can be predicted based on their simpler parts. We show that the quality of such predictions compete with macro-learning ones, while enabling prospective scenarios. We assess our work on a real size, operational bus network data set.
更多查看译文
关键词
Compositional, Machine learning, Graph, Prospective, Link weight prediction
AI 理解论文
溯源树
样例
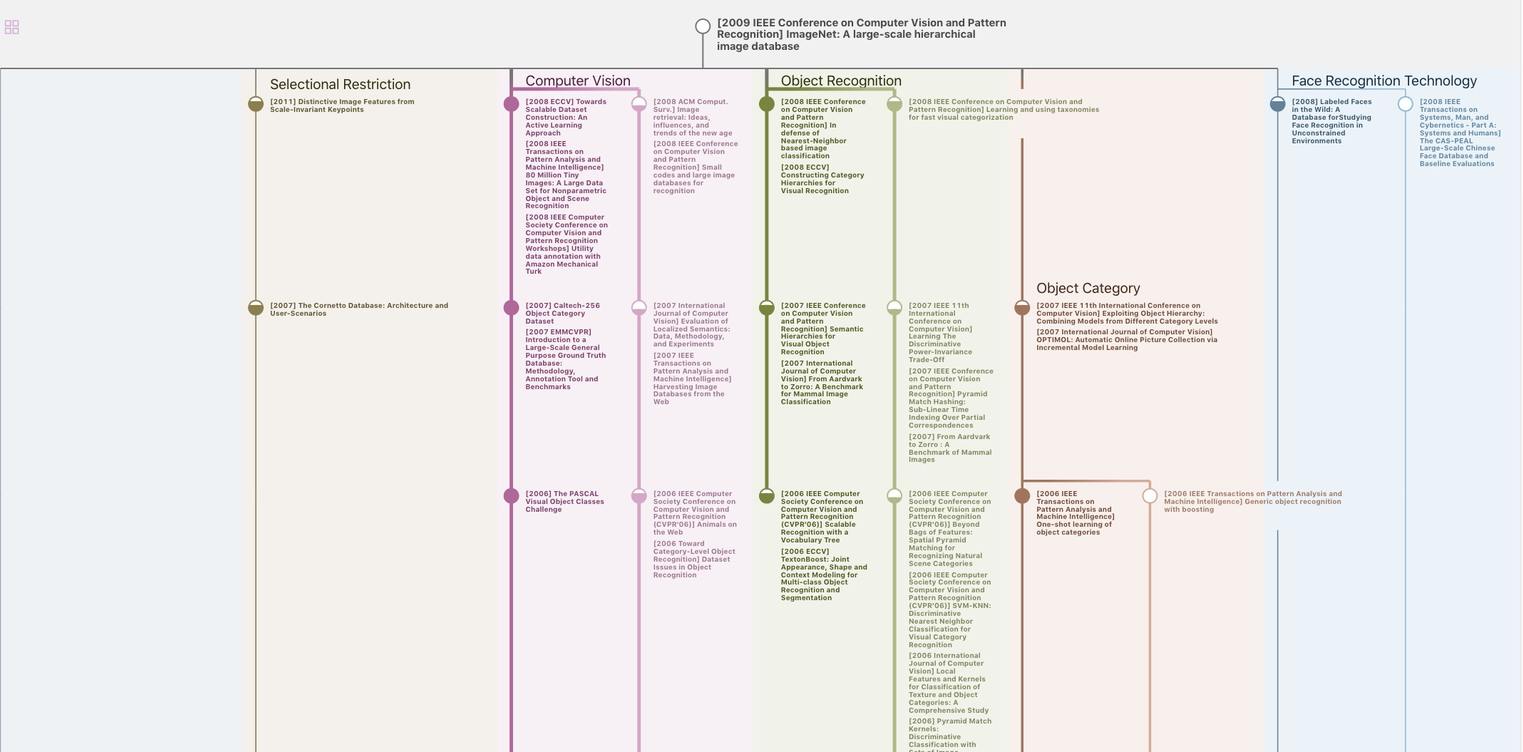
生成溯源树,研究论文发展脉络
Chat Paper
正在生成论文摘要