MSMANet: A multi-scale mesh aggregation network for brain tumor segmentation
APPLIED SOFT COMPUTING(2021)
摘要
The fine segmentation of brain tumor, which is instrumental in brain tumor diagnosis, treatment planning and prognosis, is becoming a research hotspot in medical images processing. However, manual segmentation is labor-intensive, time-consuming and easily affected by subjective and objective factors. Automatic segmentation methods based on convolutional neural networks have attracted significant interest in recent years. In this paper, a novel multi-scale mesh aggregation network (MSMANet) for brain tumor segmentation is proposed. Firstly, an improved Inception module is introduced to replace the standard convolution in the encoder to extract and aggregate effective information from different receptive fields. Secondly, a novel mesh aggregation strategy is proposed to gradually refine the shallow features and further alleviate the semantic gap. This strategy maximizes the aggregation of multi-level features at different scales and realizes the complementary advantages among features. Finally, the ability of network identification and convergence is improved by employing attention mechanism and deep supervision. Experiments were implemented on BraTS2018 to evaluate the proposed network. The dice similarity coefficient (DSC) of MSMANet in enhancing tumor, whole tumor and tumor core are 0.758, 0.890 and 0.811, respectively. Experimental results indicate that satisfactory performance is achieved compared with the state-of-the-art methods. (C) 2021 Elsevier B.V. All rights reserved.
更多查看译文
关键词
Mesh aggregation, Multi-scale, Attention mechanism, Deep supervision, Brain tumor segmentation
AI 理解论文
溯源树
样例
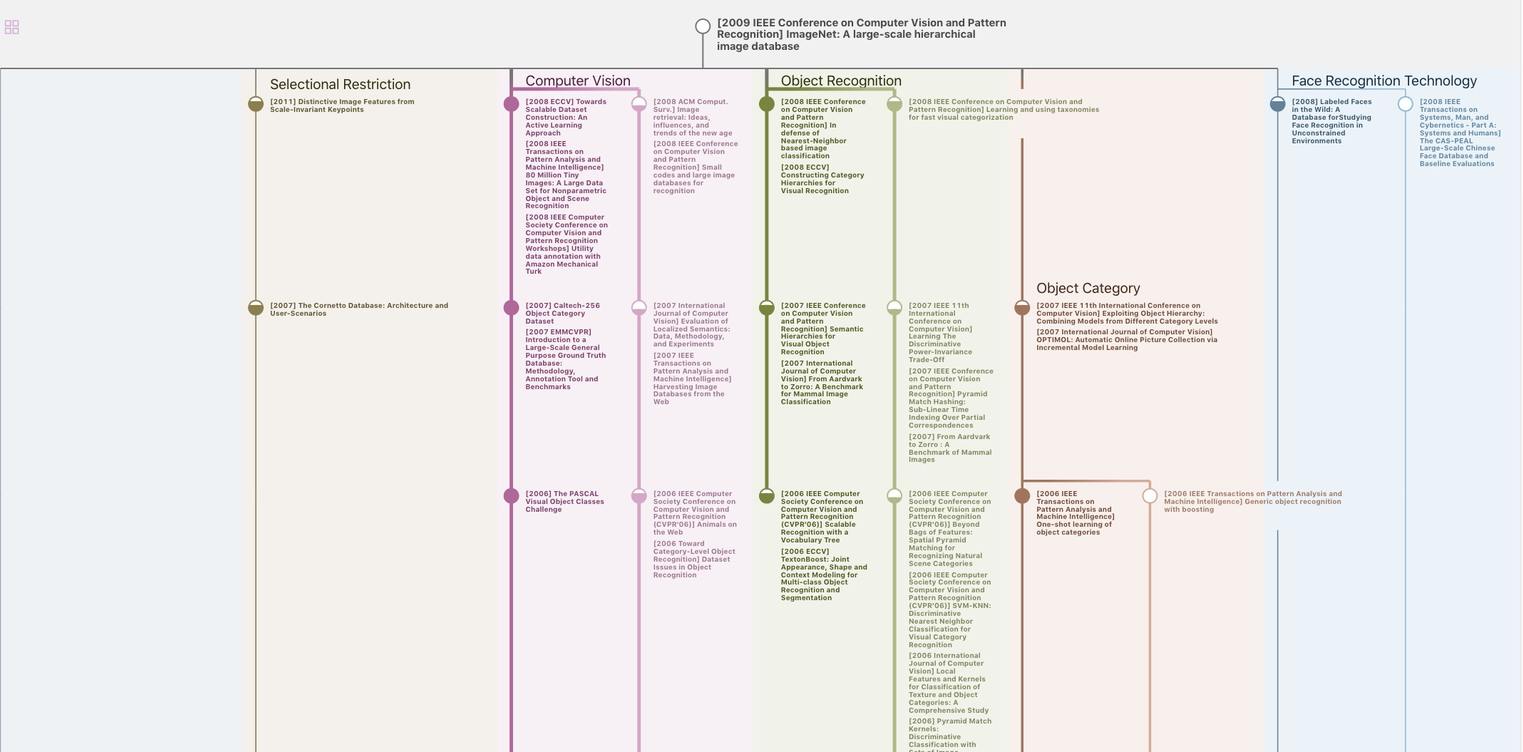
生成溯源树,研究论文发展脉络
Chat Paper
正在生成论文摘要