A deep neural network model for fashion collocation recommendation using side information in e-commerce
APPLIED SOFT COMPUTING(2021)
摘要
A fashion collocation recommendation model is a useful tool to enhance customer experiences and increase cross-selling in e-commerce. Prior research efforts focus on using titles and images to build the model. However, accessible side information in e-commerce, such as textual descriptions, purchase data and category information of items, generally bear valuable information regarding this task. This study seeks to develop a fashion collocation recommendation model that leverages accessible side information in e-commerce. First, a knowledge graph is built to model the purchase data and category information of items and an approach is subsequently proposed to learn the knowledge embeddings in the knowledge graph. To cope with the challenge of Chinese titles, a model is developed to encode the Chinese titles into vectors. Finally, a fashion collocation recommendation model combines the knowledge embeddings of the knowledge graph and the encoded titles to compute the probability of fashion collocation between items. The experiments are conducted on two datasets. Compared with baseline models, our model obtains nearly 6% and 1% AUC improvement in TIANCHI and POG dataset, respectively. The experimental results demonstrate that our efforts to leverage side information to improve fashion collocation recommendations are feasible and effective. (C) 2021 Elsevier B.V. All rights reserved.
更多查看译文
关键词
Collocation recommendation, Knowledge graph, Deep learning
AI 理解论文
溯源树
样例
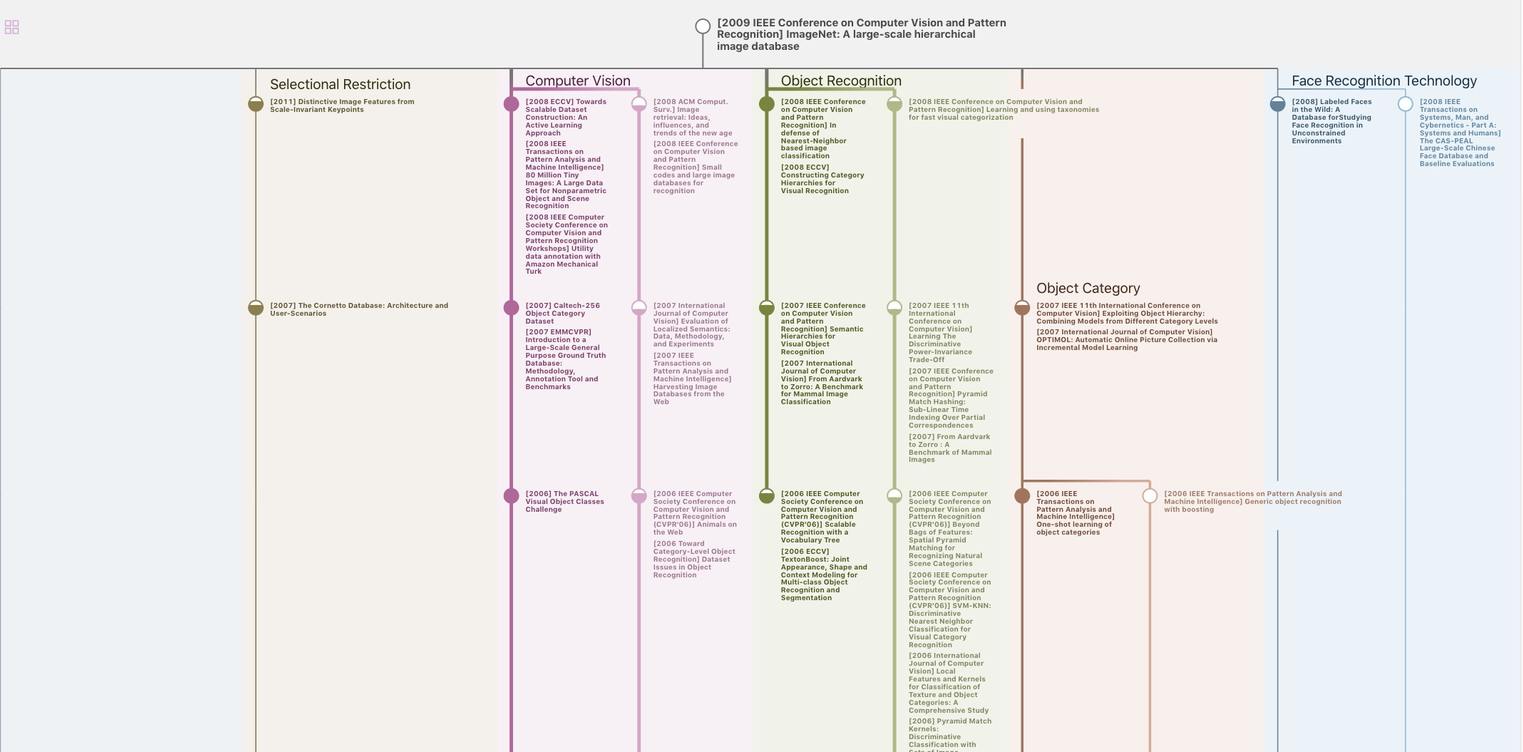
生成溯源树,研究论文发展脉络
Chat Paper
正在生成论文摘要