Graph convolutional networks for enhanced resolution 3D Electrical Capacitance Tomography image reconstruction
APPLIED SOFT COMPUTING(2021)
摘要
Three dimensional Electrical Capacitance Tomography (3D ECT) is an inexpensive tool for diagnosing non-conductive components of industrial processes. Although relatively mature, it still requires much work to improve its inverse nature of imaging capability. In particular, high resolution 3D ECT image reconstruction is very time-consuming and computationally heavy, and the best-known 3D ECT image reconstruction techniques have already reached their limits. Thus, there is a strong need to change a direction towards modern computational intelligence solutions. Therefore, this work proposes using graph convolutional networks (GCN) to raise the 3D ECT image quality. Mainly, it takes advantage of GCN's ability to effectively use specific geometrical relationships hidden in the finite modeling unstructured grids commonly used to build 3D ECT images. These relationships are first encoded by a graph representing an ECT volumetric finite element grid. A GCN is next trained in a graph-to-graph framework with pairs of graphs representing high-quality nonlinear image reconstruction results as input and a simulated phantom as output. As a result, a trained GCN model fed with lower resolution 3D ECT image enhances its quality and spatial resolution. Tomographic image quality and resolution enhancement was evaluated using normalized mean square error and Pearson correlation coefficient, which improved by 35.5% and 3.74%, respectively. (C) 2021 Elsevier B.V. All rights reserved.
更多查看译文
关键词
3D capacitance tomography, Non-invasive imaging, Image reconstruction, Graph convolutional networks, Geometric deep learning
AI 理解论文
溯源树
样例
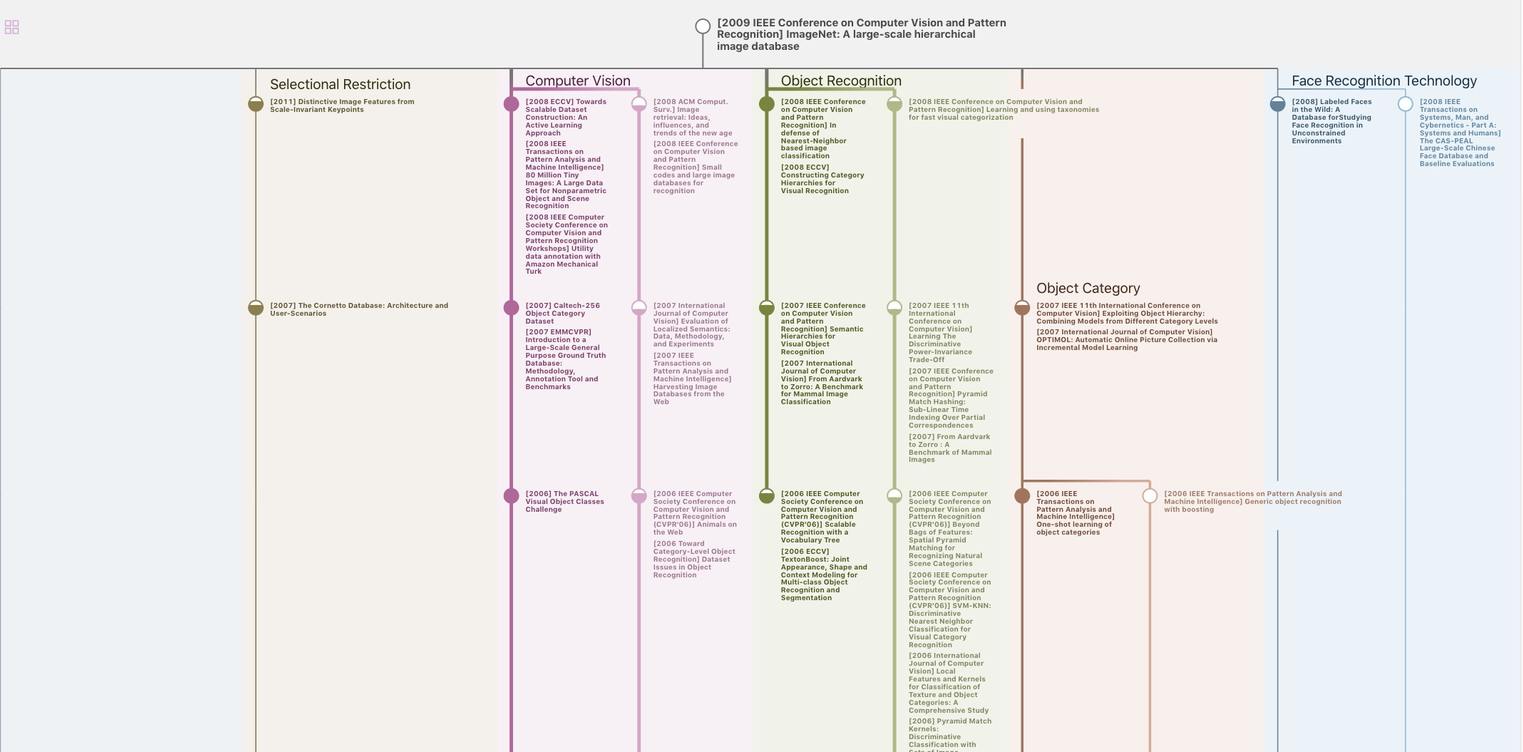
生成溯源树,研究论文发展脉络
Chat Paper
正在生成论文摘要