scDEA: differential expression analysis in single-cell RNA-sequencing data via ensemble learning.
BRIEFINGS IN BIOINFORMATICS(2022)
摘要
The identification of differentially expressed genes between different cell groups is a crucial step in analyzing single-cell RNA-sequencing (scRNA-seq) data. Even though various differential expression analysis methods for scRNA-seq data have been proposed based on different model assumptions and strategies recently, the differentially expressed genes identified by them are quite different from each other, and the performances of them depend on the underlying data structures. In this paper, we propose a new ensemble learning-based differential expression analysis method, scDEA, to produce a more stable and accurate result. scDEA integrates the P-values obtained from 12 individual differential expression analysis methods for each gene using a P-value combination method. Comprehensive experiments show that scDEA outperforms the state-of-the-art individual methods with different experimental settings and evaluation metrics. We expect that scDEA will serve a wide range of users, including biologists, bioinformaticians and data scientists, who need to detect differentially expressed genes in scRNA-seq data.
更多查看译文
关键词
scRNA-seq, differential expression analysis, ensemble learning
AI 理解论文
溯源树
样例
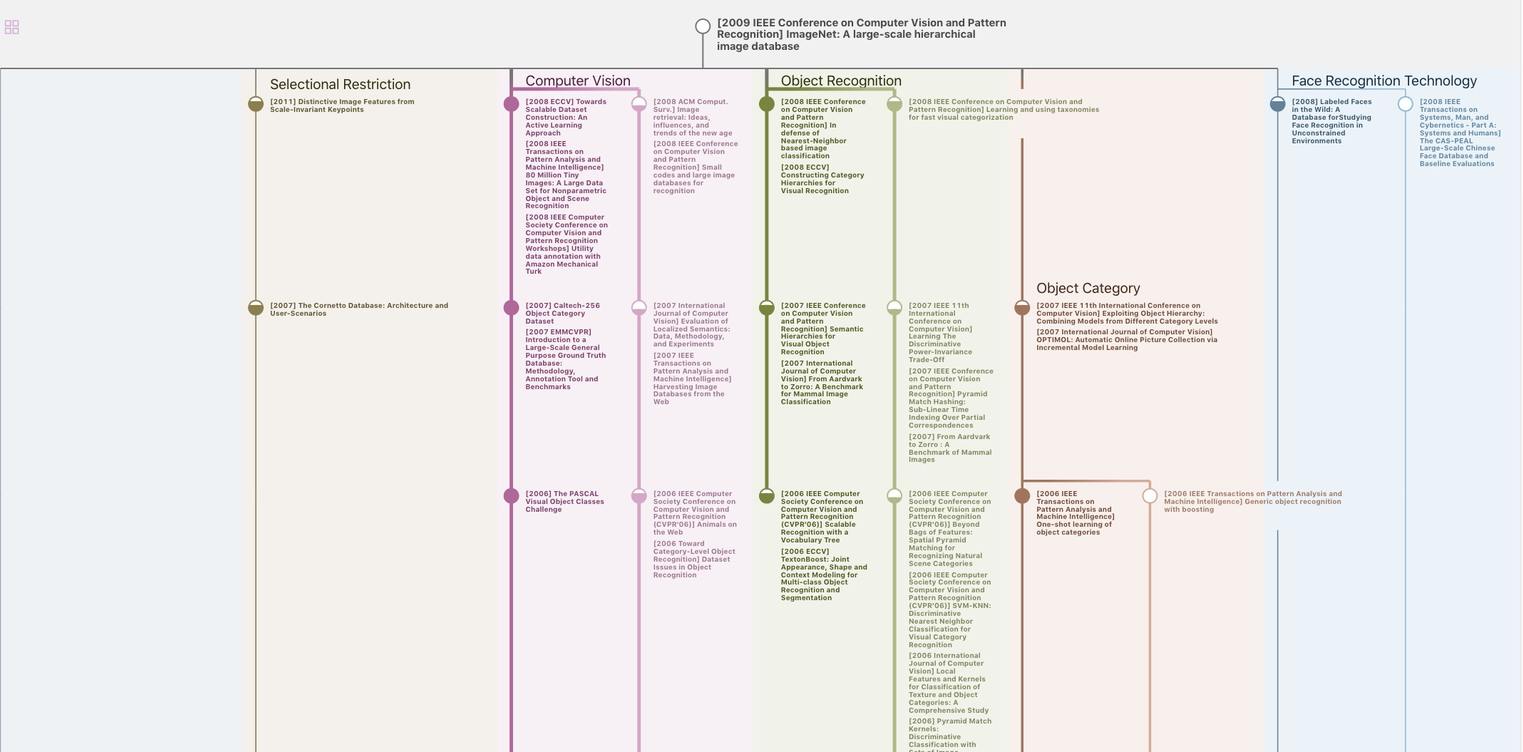
生成溯源树,研究论文发展脉络
Chat Paper
正在生成论文摘要