Predicting Task-Driven Attention Via Integrating Bottom-Up Stimulus And Top-Down Guidance
IEEE TRANSACTIONS ON IMAGE PROCESSING(2021)
摘要
Task-free attention has gained intensive interest in the computer vision community while relatively few works focus on task-driven attention (TDAttention). Thus this paper handles the problem of TDAttention prediction in daily scenarios where a human is doing a task. Motivated by the cognition mechanism that human attention allocation is jointly controlled by the top-down guidance and bottom-up stimulus, this paper proposes a cognitively-explanatory deep neural network model to predict TDAttention. Given an image sequence, bottom-up features, such as human pose and motion, are firstly extracted. At the same time, the coarse-grained task information and fine-grained task information are embedded as a top-down feature. The bottom-up features are then fused with the top-down feature to guide the model to predict TDAttention. Two public datasets are re-annotated to make them qualified for TDAttention prediction, and our model is widely compared with other models on the two datasets. In addition, some ablation studies are conducted to evaluate the individual modules in our model. Experiment results demonstrate the effectiveness of our model.
更多查看译文
关键词
Task analysis, Predictive models, Feature extraction, Image color analysis, Visualization, Computer architecture, Psychology, Human attention, task-driven
AI 理解论文
溯源树
样例
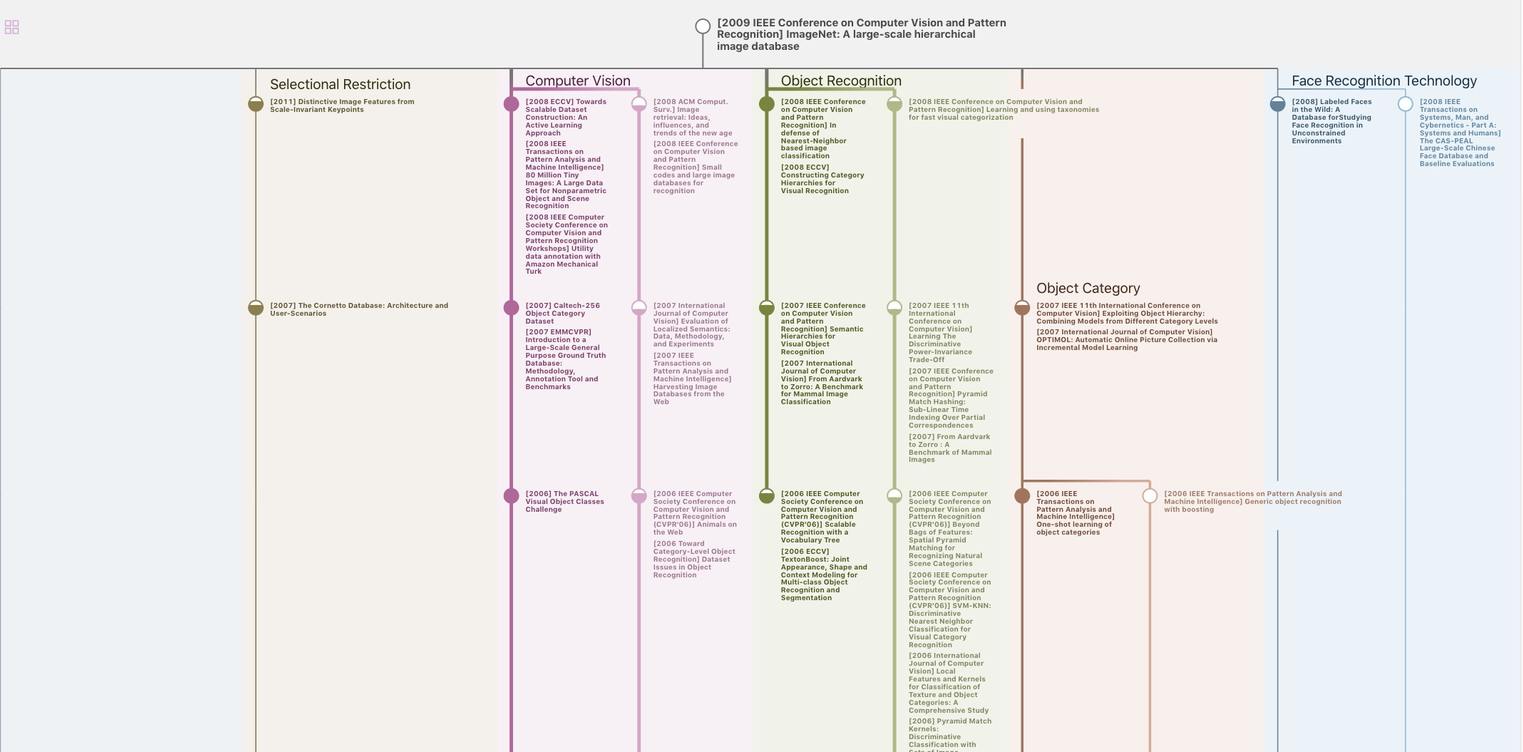
生成溯源树,研究论文发展脉络
Chat Paper
正在生成论文摘要