Physics-incorporated convolutional recurrent neural networks for source identification and forecasting of dynamical systems
Neural Networks(2021)
摘要
Spatio-temporal dynamics of physical processes are generally modeled using partial differential equations (PDEs). Though the core dynamics follows some principles of physics, real-world physical processes are often driven by unknown external sources. In such cases, developing a purely analytical model becomes very difficult and data-driven modeling can be of assistance. In this paper, we present a hybrid framework combining physics-based numerical models with deep learning for source identification and forecasting of spatio-temporal dynamical systems with unobservable time-varying external sources. We formulate our model PhICNet as a convolutional recurrent neural network (RNN) which is end-to-end trainable for spatio-temporal evolution prediction of dynamical systems and learns the source behavior as an internal state of the RNN. Experimental results show that the proposed model can forecast the dynamics for a relatively long time and identify the sources as well.
更多查看译文
关键词
Dynamical systems,Partial differential equation,Recurrent neural networks,Physics-incorporated neural networks
AI 理解论文
溯源树
样例
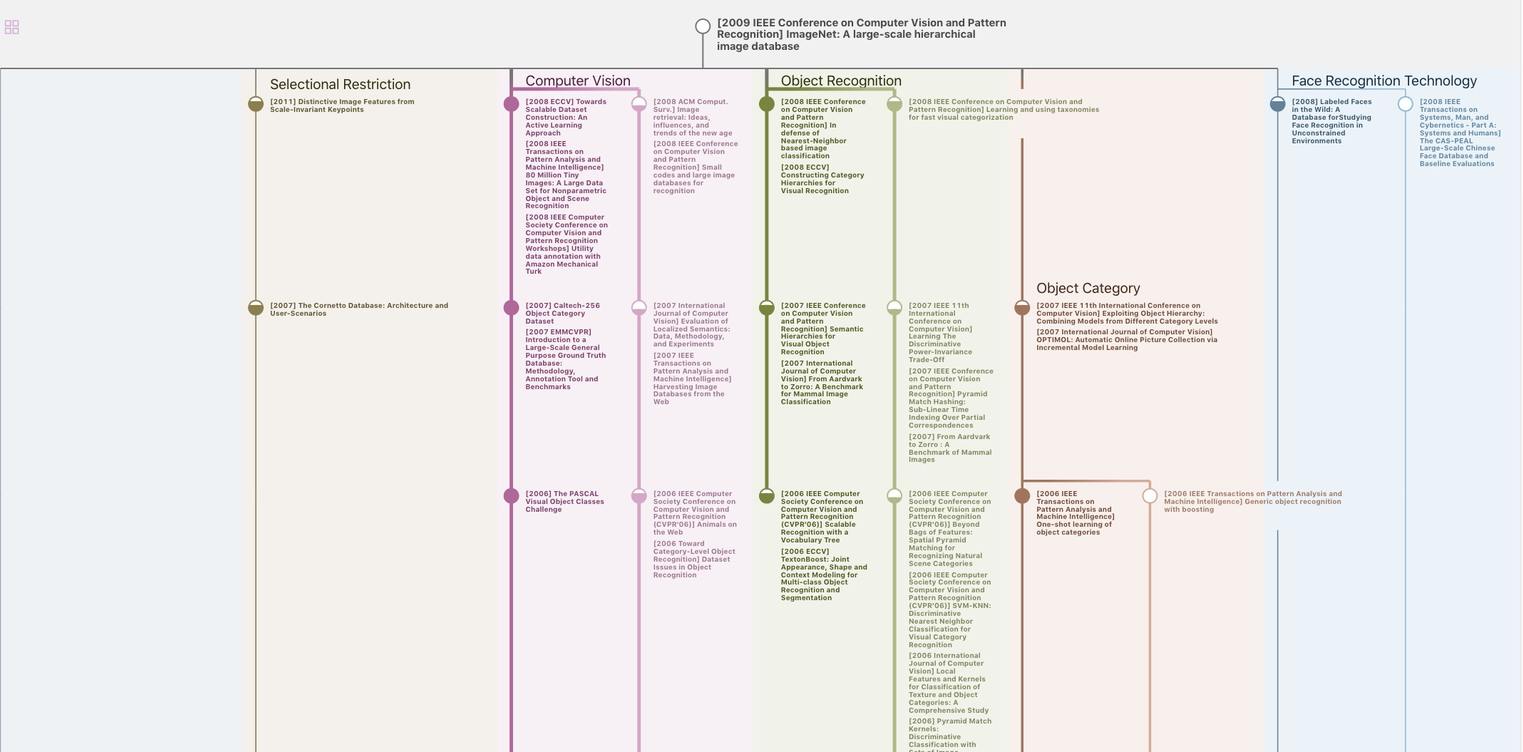
生成溯源树,研究论文发展脉络
Chat Paper
正在生成论文摘要