Online Object Model Reconstruction and Reuse for Lifelong Improvement of Robot Manipulation
IEEE International Conference on Robotics and Automation(2022)
摘要
This work proposes a robotic pipeline for picking and constrained placement of objects without geometric shape priors. Compared to recent efforts developed for similar tasks, where every object was assumed to be novel, the proposed system recognizes previously manipulated objects and per-forms online model reconstruction and reuse. Over a lifelong manipulation process, the system keeps learning features of objects it has interacted with and updates their reconstructed models. Whenever an instance of a previously manipulated object reappears, the system aims to first recognize it and then register its previously reconstructed model given the current observation. This step greatly reduces object shape uncertainty allowing the system to even reason for parts of objects, which are currently not observable. This also results in better manipulation efficiency as it reduces the need for active perception of the target object during manipulation. To get a reusable reconstructed model, the proposed pipeline adopts: i) TSDF for object representation, and ii) a variant of the standard particle filter algorithm for pose estimation and tracking of the partial object model. Furthermore, an effective way to construct and maintain a dataset of manipulated objects is presented. A sequence of real-world manipulation experiments is performed. They show how future manipulation tasks become more effective and efficient by reusing reconstructed models of previously manipulated objects, which were generated during their prior manipulation, instead of treating objects as novel every time.
更多查看译文
关键词
online object model reconstruction,robot,lifelong improvement,reuse
AI 理解论文
溯源树
样例
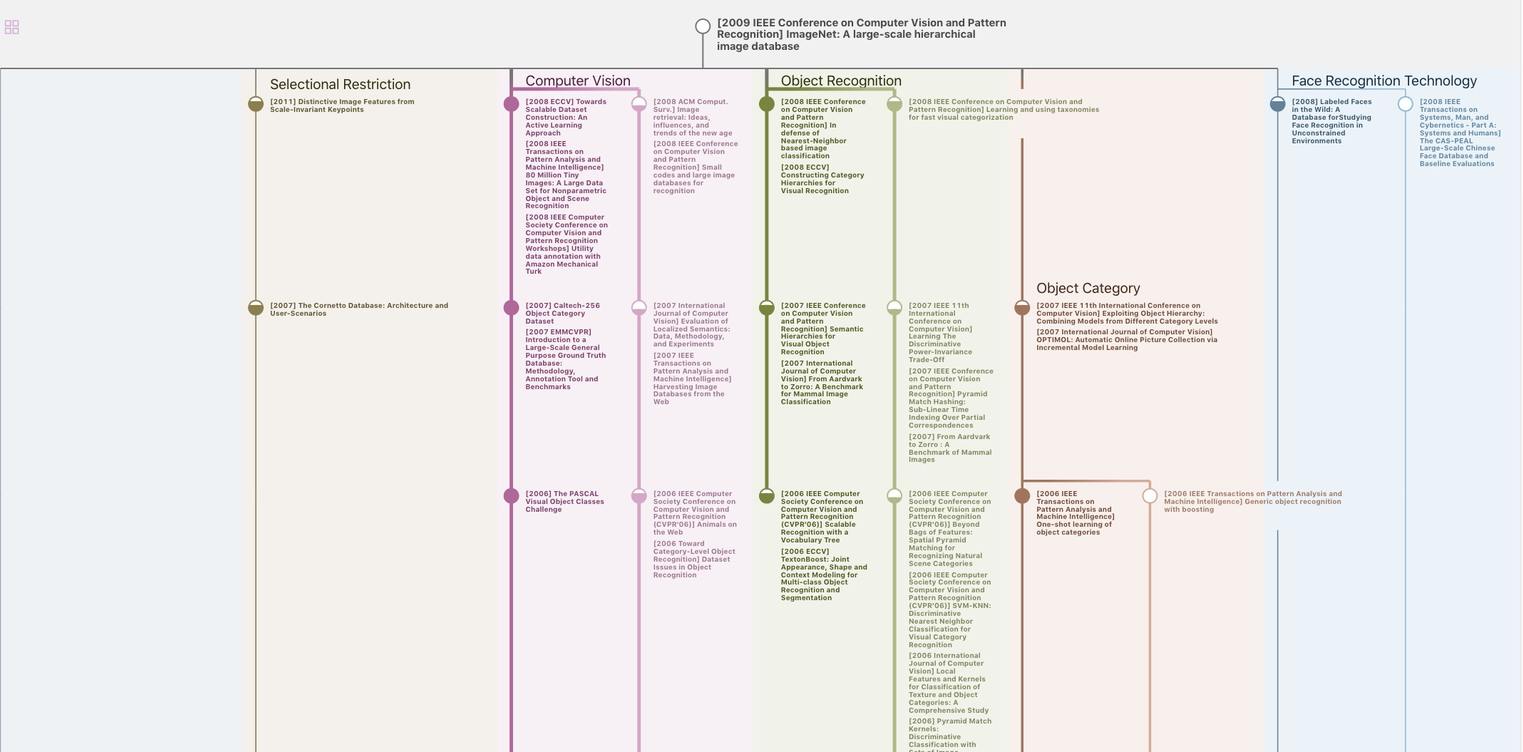
生成溯源树,研究论文发展脉络
Chat Paper
正在生成论文摘要