FlowVocoder: A small Footprint Neural Vocoder based Normalizing Flow for Speech Synthesis
Conference of the International Speech Communication Association (INTERSPEECH)(2022)
摘要
Recently, non-autoregressive neural vocoders have provided remarkable performance in generating high-fidelity speech and have been able to produce synthetic speech in real-time. However, non-autoregressive neural vocoders such as WaveGlow are far behind autoregressive neural vocoders like WaveFlow in terms of modeling audio signals due to their limitation in expressiveness. In addition, though NanoFlow is a state-of-the-art autoregressive neural vocoder that has immensely small parameters, its performance is marginally lower than WaveFlow. Therefore, in this paper, we propose a new type of autoregressive neural vocoder called FlowVocoder, which has a small memory footprint and is able to generate high-fidelity audio in real-time. Our proposed model improves the expressiveness of flow blocks by operating a mixture of Cumulative Distribution Function(CDF) for bipartite transformation. Hence, the proposed model is capable of modeling waveform signals as well as WaveFlow, while its memory footprint is much smaller thanWaveFlow. As shown in experiments, FlowVocoder achieves competitive results with baseline methods in terms of both subjective and objective evaluation, also, it is more suitable for real-time text-to-speech applications.
更多查看译文
关键词
small footprint neural flowvocoder,normalizing flowvocoder,synthesis
AI 理解论文
溯源树
样例
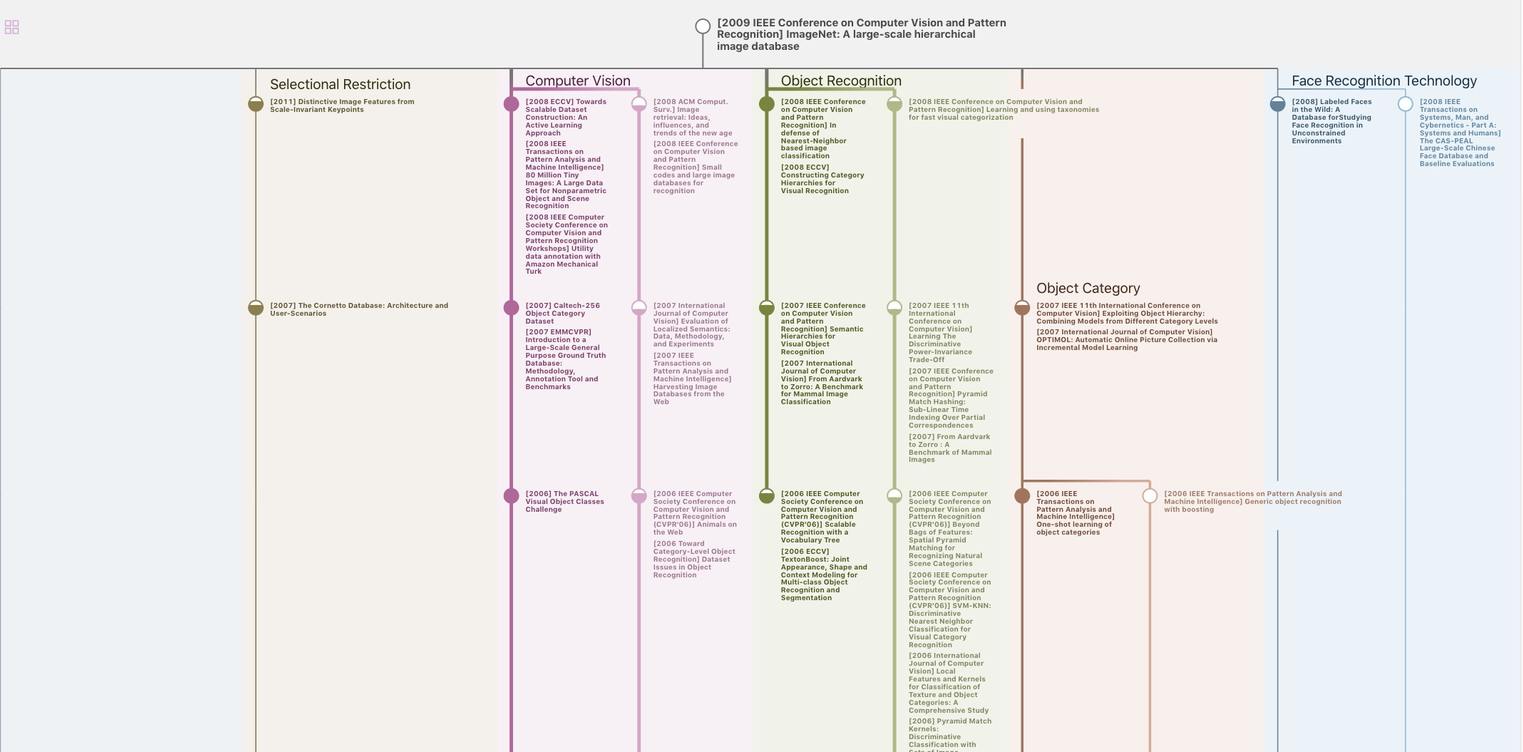
生成溯源树,研究论文发展脉络
Chat Paper
正在生成论文摘要