Multimodality in meta-learning: A comprehensive survey
Knowledge-Based Systems(2022)
摘要
Meta-learning has gained wide popularity as a training framework that is more data-efficient than traditional machine learning methods. However, its generalization ability in complex task distributions, such as multimodal tasks, has not been thoroughly studied. Recently, some studies on multimodality-based meta-learning have emerged. This survey provides a comprehensive overview of the multimodality-based meta-learning landscape in terms of the methodologies and applications. We first formalize the definition of meta-learning in multimodality, along with the research challenges in this growing field, such as how to enrich the input in few-shot learning (FSL) or zero-shot learning (ZSL) in multimodal scenarios and how to generalize the models to new tasks. We then propose a new taxonomy to discuss typical meta-learning algorithms in multimodal tasks systematically. We investigate the contributions of related papers and summarize them by our taxonomy. Finally, we propose potential research directions for this promising field.
更多查看译文
关键词
Meta-learning,Multimodal,Deep learning,Few-shot learning,Zero-shot learning
AI 理解论文
溯源树
样例
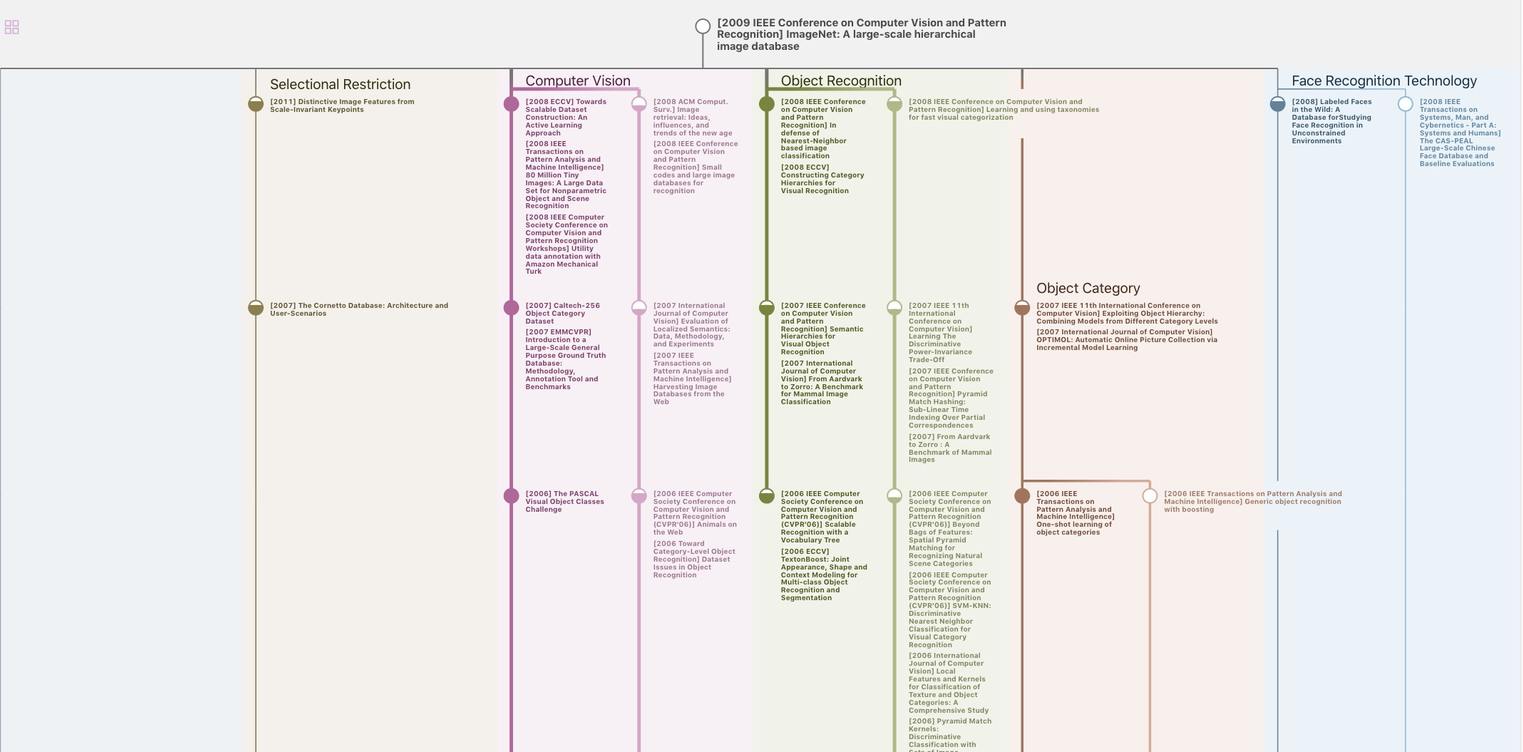
生成溯源树,研究论文发展脉络
Chat Paper
正在生成论文摘要