Graph-Based Spatial-Temporal Convolutional Network for Vehicle Trajectory Prediction in Autonomous Driving
IEEE Transactions on Intelligent Transportation Systems(2022)
摘要
Forecasting the trajectories of neighbor vehicles is a crucial step for decision making and motion planning of autonomous vehicles. This paper proposes a graph-based spatial-temporal convolutional network (GSTCN) to predict future trajectory distributions of all neighbor vehicles using past trajectories. This network tackles spatial interactions using a graph convolutional network (GCN), and captures temporal features with a convolutional neural network (CNN). The spatial-temporal features are encoded and decoded by a gated recurrent unit (GRU) network to generate future trajectory distributions. Besides, we propose a weighted adjacency matrix to describe the intensities of mutual influence between vehicles, and the ablation study demonstrates the effectiveness of our scheme. Our network is evaluated on two real-world freeway trajectory datasets: I-80 and US-101 in the Next Generation Simulation (NGSIM). Comparisons in three aspects, including prediction errors, model sizes, and inference speeds, show that our network can achieve state-of-the-art performance.
更多查看译文
关键词
Vehicle trajectory prediction,graph convolutional network,spatial-temporal dependency,autonomous driving
AI 理解论文
溯源树
样例
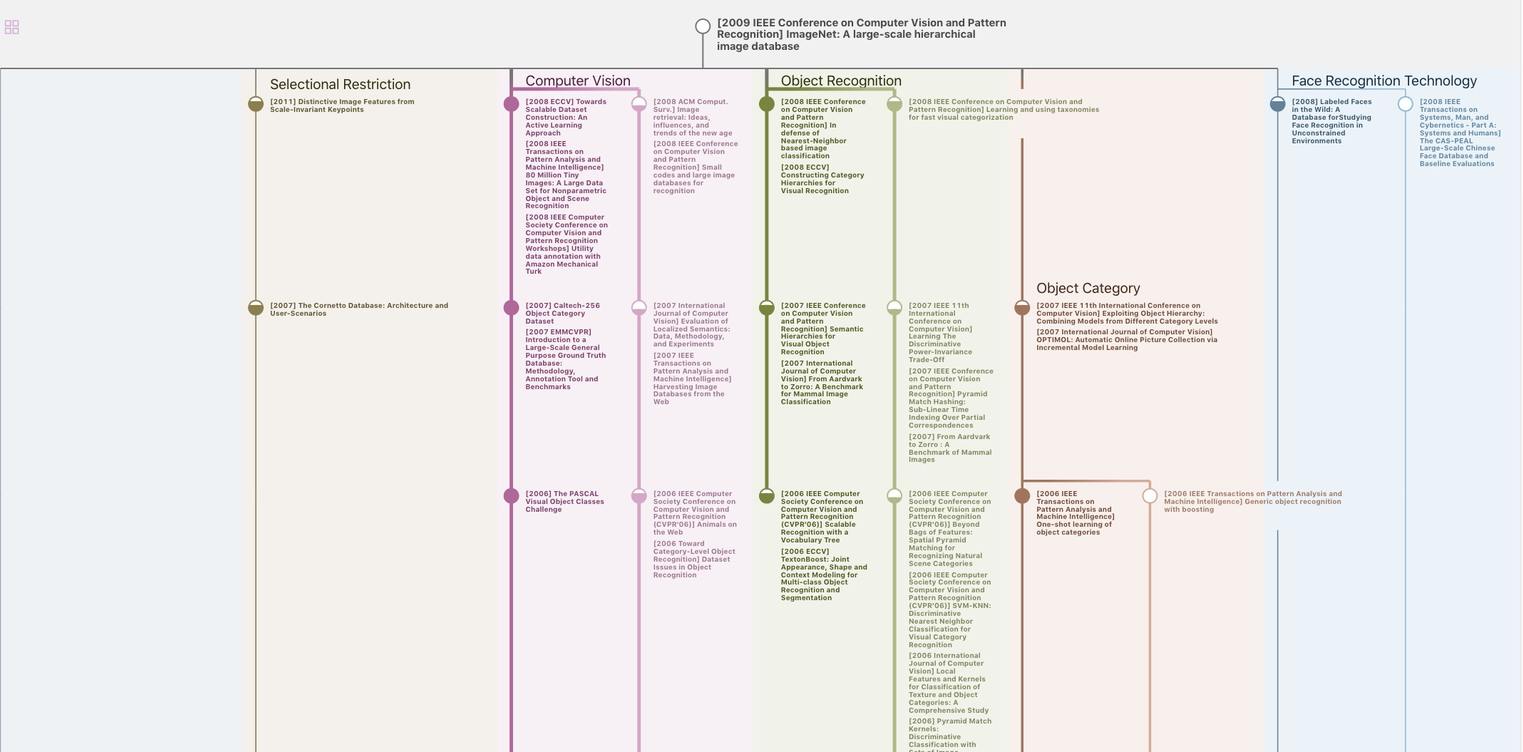
生成溯源树,研究论文发展脉络
Chat Paper
正在生成论文摘要