Uniting Nonempirical and Empirical Density Functional Approximation Strategies Using Constraint-Based Regularization
arxiv(2022)
摘要
In this work, we present a general framework that unites the two primary strategies for constructing density functional approximations (DFAs): nonempirical (NE) constraint satisfaction and empirical (E) data-driven optimization. The proposed method employs B-splines, bell-shaped spline functions with compact support, to construct each inhomogeneity correction factor (ICF). This choice offers several distinct advantages over traditional polynomial expansions by enabling explicit enforcement of linear and nonlinear constraints as well as ICF smoothness using Tikhonov and penalized B-splines (P-splines) regularization. As proof-of-concept, we use the so-called CASE (constrained and smoothed empirical) framework to construct a constraint-satisfying and data-driven global hybrid that exhibits enhanced performance across a diverse set of chemical properties. We argue that the CASE approach can be used to generate DFAs that maintain the physical rigor and transferability of NE-DFAs while leveraging high-quality quantum-mechanical data to remove the arbitrariness of ansatz selection and improve performance.
更多查看译文
关键词
regularization,approximation,density,constraint-based
AI 理解论文
溯源树
样例
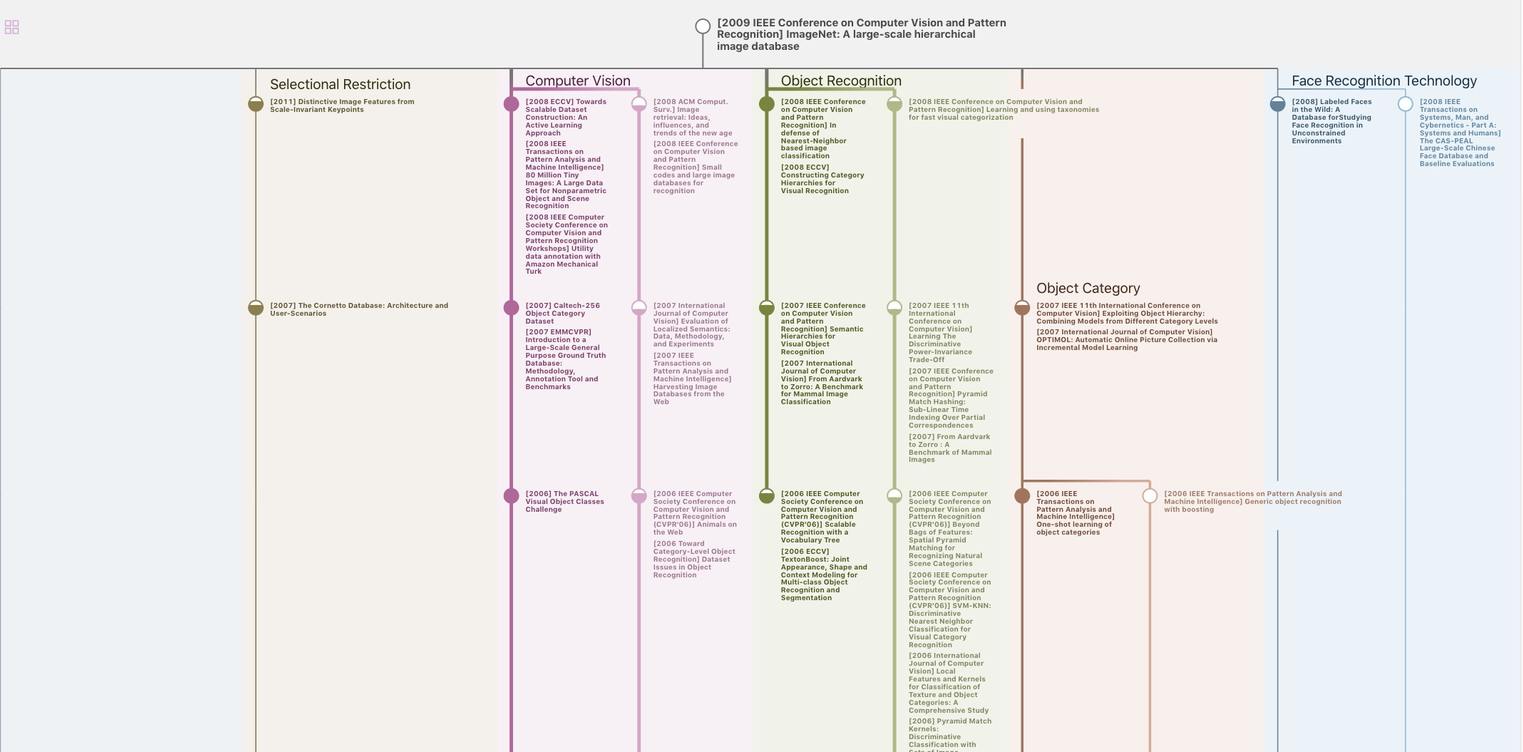
生成溯源树,研究论文发展脉络
Chat Paper
正在生成论文摘要