Accelerated consensus in multi-agent networks via memory of local averages.
CDC(2021)
摘要
Classical mathematical models of information sharing and updating in multi-agent networks use linear operators. In the paradigmatic DeGroot model, agents update their states with linear combinations of their neighbors' current states. In prior work, an accelerated averaging model employing the use of memory has been suggested to accelerate convergence to a consensus state for undirected networks. There, the DeGroot update on the current states is followed by a linear combination with the previous states. We propose a modification where the DeGroot update is applied to the current and previous states and is then followed by a linear combination step. We show that this simple modification applied to undirected networks permits convergence even for periodic networks. Further, it allows for faster convergence than the DeGroot and accelerated averaging models for suitable networks and model parameters.
更多查看译文
关键词
accelerated consensus,multiagent networks,local averages,information sharing,linear operators,paradigmatic DeGroot model,accelerated averaging model,undirected networks,DeGroot update,periodic networks
AI 理解论文
溯源树
样例
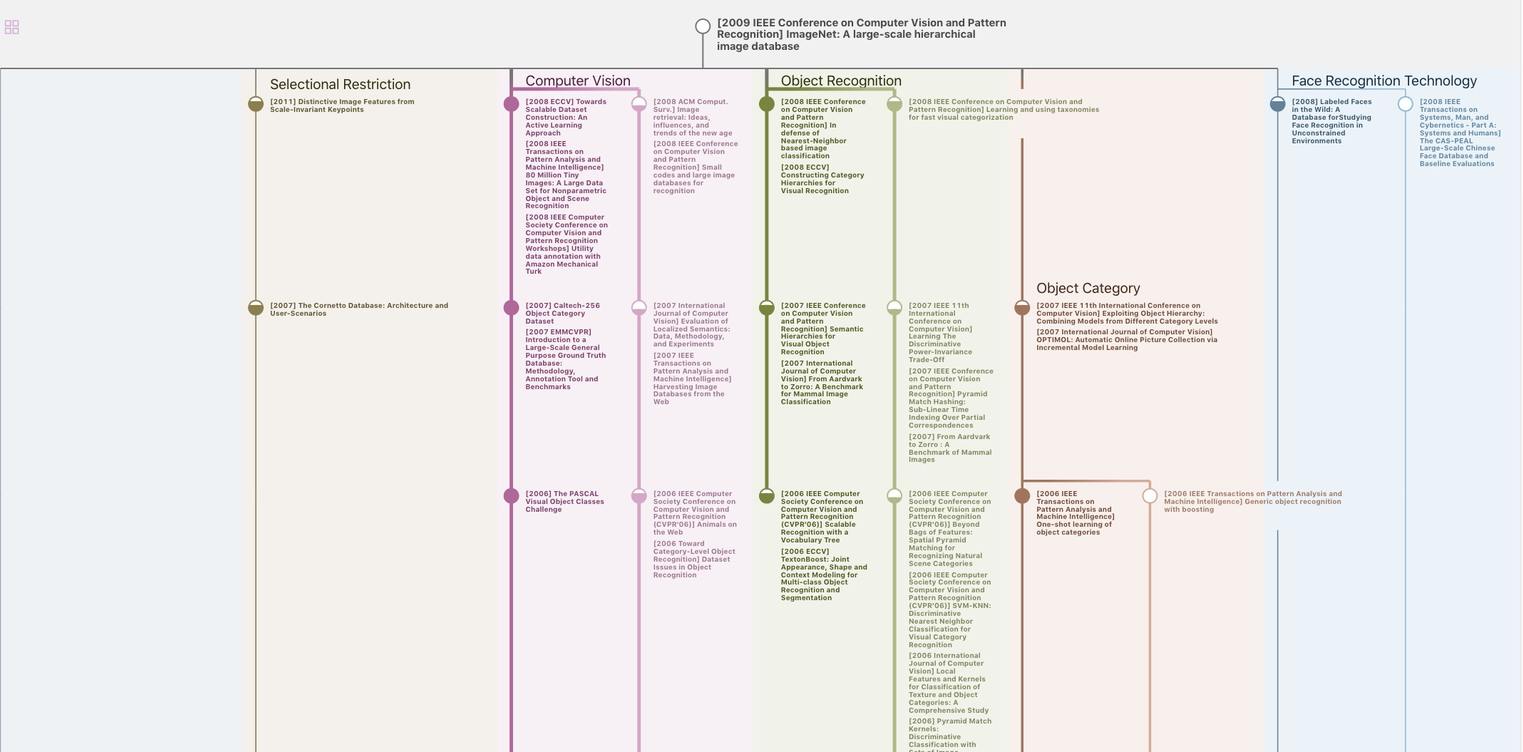
生成溯源树,研究论文发展脉络
Chat Paper
正在生成论文摘要