Topic Model Robustness to Automatic Speech Recognition Errors in Podcast Transcripts
arxiv(2021)
摘要
For a multilingual podcast streaming service, it is critical to be able to deliver relevant content to all users independent of language. Podcast content relevance is conventionally determined using various metadata sources. However, with the increasing quality of speech recognition in many languages, utilizing automatic transcriptions to provide better content recommendations becomes possible. In this work, we explore the robustness of a Latent Dirichlet Allocation topic model when applied to transcripts created by an automatic speech recognition engine. Specifically, we explore how increasing transcription noise influences topics obtained from transcriptions in Danish; a low resource language. First, we observe a baseline of cosine similarity scores between topic embeddings from automatic transcriptions and the descriptions of the podcasts written by the podcast creators. We then observe how the cosine similarities decrease as transcription noise increases and conclude that even when automatic speech recognition transcripts are erroneous, it is still possible to obtain high-quality topic embeddings from the transcriptions.
更多查看译文
关键词
automatic speech recognition errors,podcast
AI 理解论文
溯源树
样例
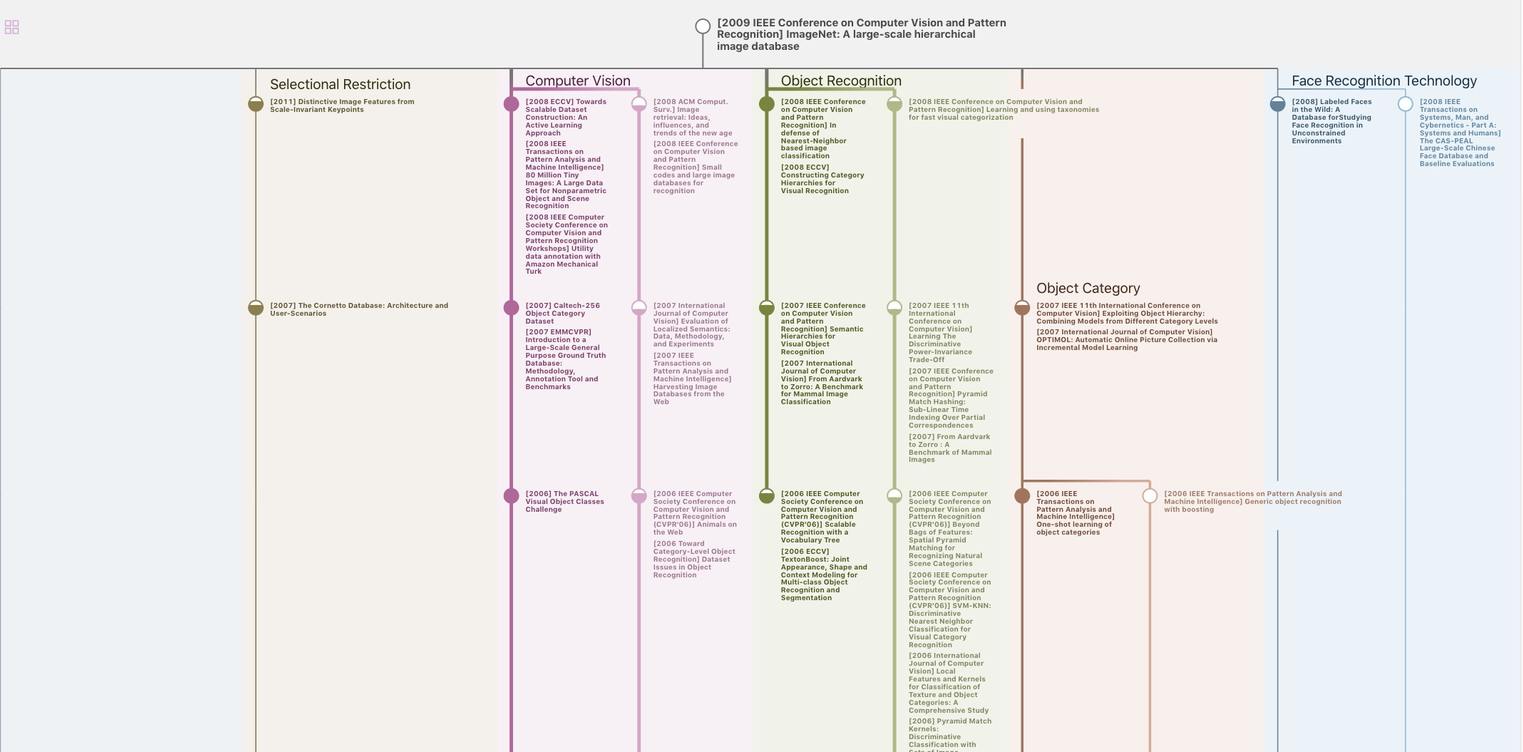
生成溯源树,研究论文发展脉络
Chat Paper
正在生成论文摘要