List-GRAND: A Practical Way to Achieve Maximum Likelihood Decoding
IEEE Transactions on Very Large Scale Integration (VLSI) Systems(2023)
摘要
Guessing random additive noise decoding (GRAND) is a recently proposed universal maximum likelihood (ML) decoder for short-length and high-rate linear block codes. Soft-GRAND (SGRAND) is a prominent soft-input GRAND variant, outperforming the other GRAND variants in decoding performance; nevertheless, SGRAND is not suitable for parallel hardware implementation. Ordered Reliability Bits-GRAND (ORBGRAND) is another soft-input GRAND variant that is suitable for parallel hardware implementation; however, it has lower decoding performance than SGRAND. In this article, we propose List-GRAND (LGRAND), a technique for enhancing the decoding performance of ORBGRAND to match the ML decoding performance of SGRAND. Numerical simulation results show that LGRAND enhances ORBGRAND’s decoding performance by 0.5–0.75 dB for channel codes of various classes at a target frame error rate (FER) of 10−7. For linear block codes of length 127/128 and different code rates, LGRAND’s VLSI implementation can achieve an average information throughput of 47.27–51.36 Gb/s. In comparison to ORBGRAND’s VLSI implementation, the proposed LGRAND hardware has a 4.84% area overhead.
更多查看译文
关键词
Guessing random additive noise decoding (GRAND),maximum likelihood (ML) decoding,ordered reliability bits GRAND (ORBGRAND),soft GRAND (SGRAND),ultra reliable and low-latency communication (URLLC)
AI 理解论文
溯源树
样例
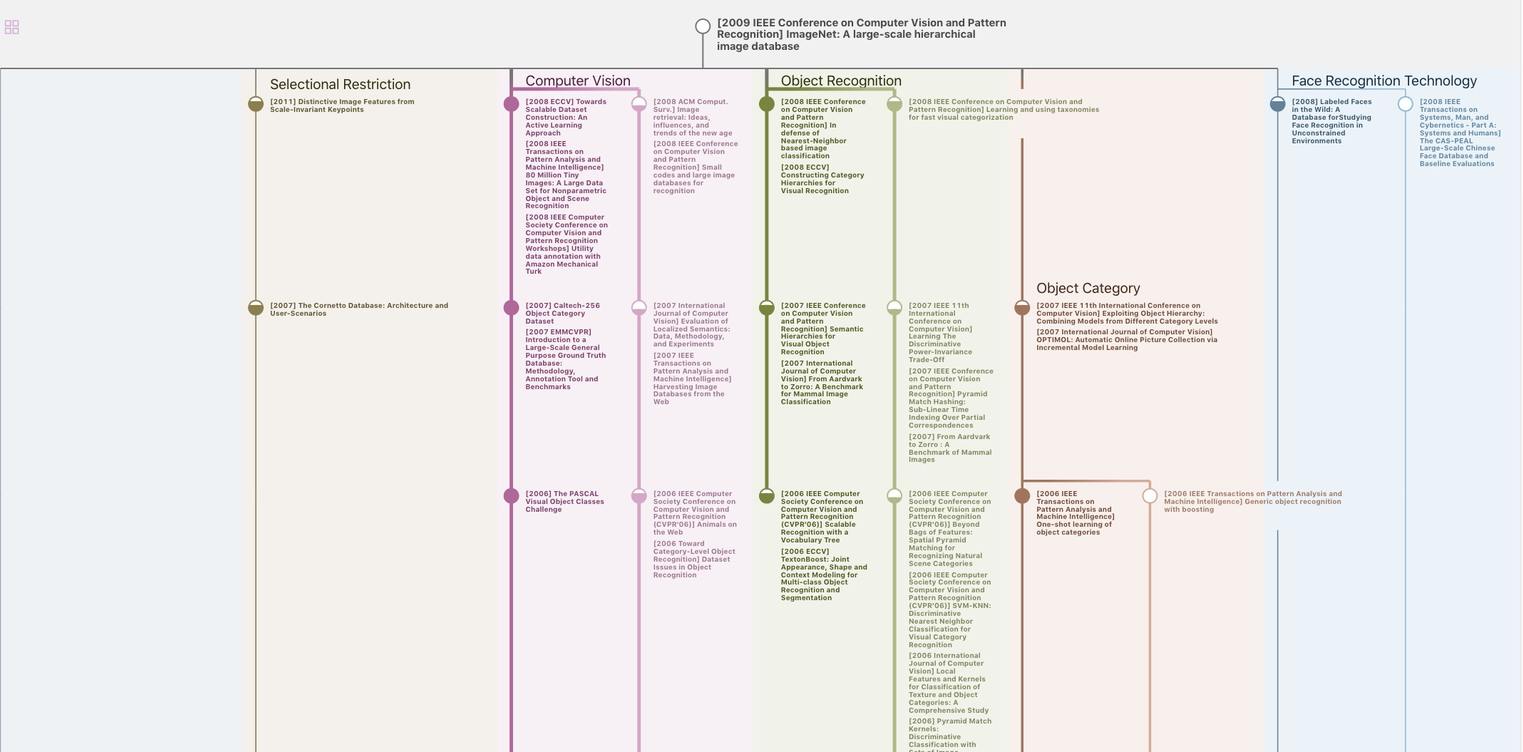
生成溯源树,研究论文发展脉络
Chat Paper
正在生成论文摘要