Adversarial Domain Feature Adaptation for Bronchoscopic Depth Estimation
MEDICAL IMAGE COMPUTING AND COMPUTER ASSISTED INTERVENTION - MICCAI 2021, PT IV(2021)
摘要
Depth estimation from monocular images is an important task in localization and 3D reconstruction pipelines for bronchoscopic navigation. Various supervised and self-supervised deep learning-based approaches have proven themselves on this task for natural images. However, the lack of labeled data and the bronchial tissue's feature-scarce texture make the utilization of these methods ineffective on bronchoscopic scenes. In this work, we propose an alternative domain-adaptive approach. Our novel two-step structure first trains a depth estimation network with labeled synthetic images in a supervised manner; then adopts an unsupervised adversarial domain feature adaptation scheme to improve the performance on real images. The results of our experiments show that the proposed method improves the network's performance on real images by a considerable margin and can be employed in 3D reconstruction pipelines.
更多查看译文
关键词
Bronchoscopy, Depth estimation, Domain adaptation
AI 理解论文
溯源树
样例
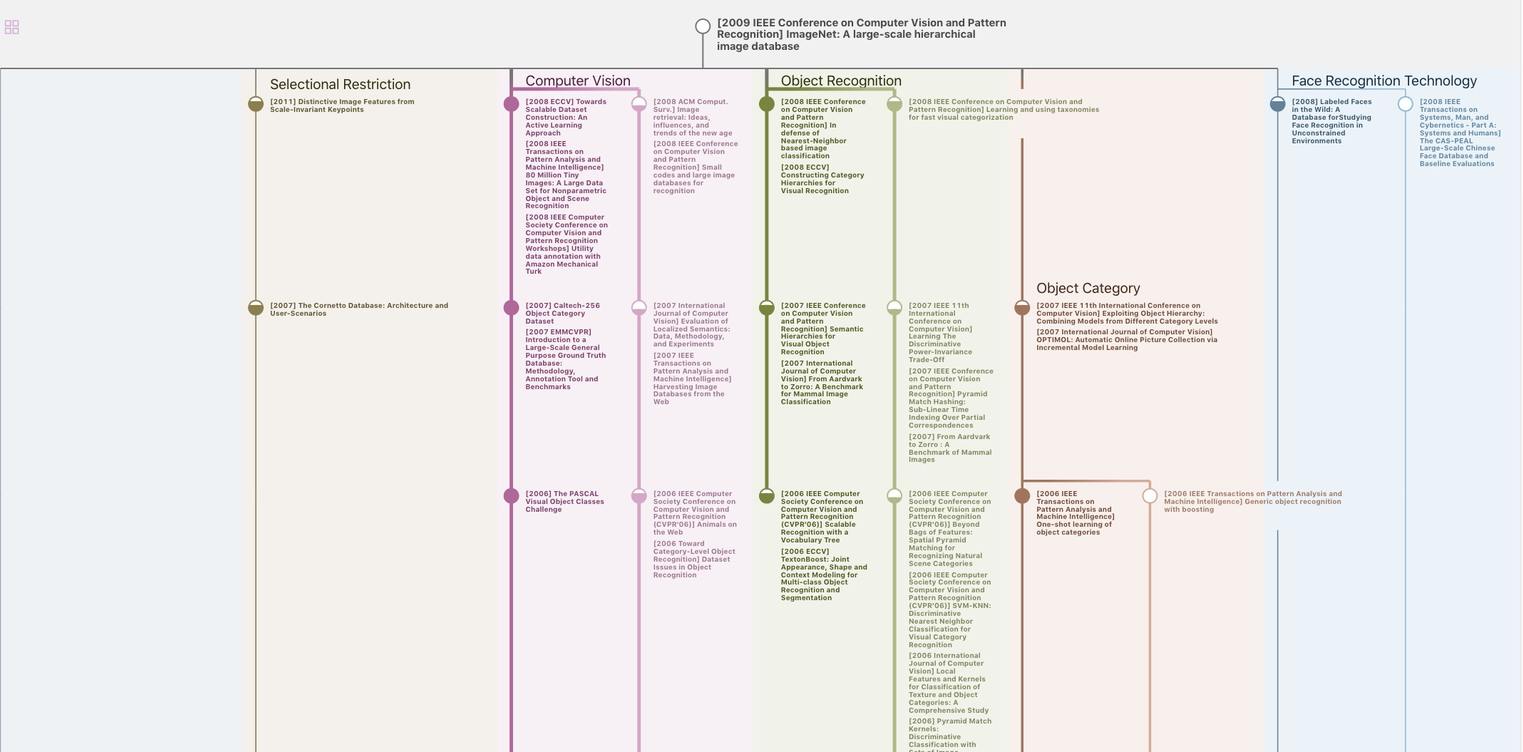
生成溯源树,研究论文发展脉络
Chat Paper
正在生成论文摘要