A Unified Graph-Based Approach to Disinformation Detection Using Contextual and Semantic Relations
International Conference on Web and Social Media (ICWSM)(2022)
摘要
As recent events have demonstrated, disinformation spread through social networks can have dire political, economic and social consequences. Detecting disinformation must inevitably rely on the structure of the network, on users particularities and on event occurrence patterns. We present a graph data structure, which we denote as a meta-graph, that combines underlying users' relational event information, as well as semantic and topical modeling. We detail the construction of an example meta-graph using Twitter data covering the 2016 US election campaign and then compare the detection of disinformation at cascade level, using well-known graph neural network algorithms, to the same algorithms applied on the meta-graph nodes. The comparison shows a consistent 3%-4% improvement in accuracy when using the meta-graph, over all considered algorithms, compared to basic cascade classification, and a further 1% increase when topic modeling and sentiment analysis are considered. We carry out the same experiment on two other datasets, HealthRelease and HealthStory, part of the FakeHealth dataset repository, with consistent results. Finally, we discuss further advantages of our approach, such as the ability to augment the graph structure using external data sources, the ease with which multiple meta-graphs can be combined as well as a comparison of our method to other graph-based disinformation detection frameworks.
更多查看译文
关键词
disinformation detection,graph-based
AI 理解论文
溯源树
样例
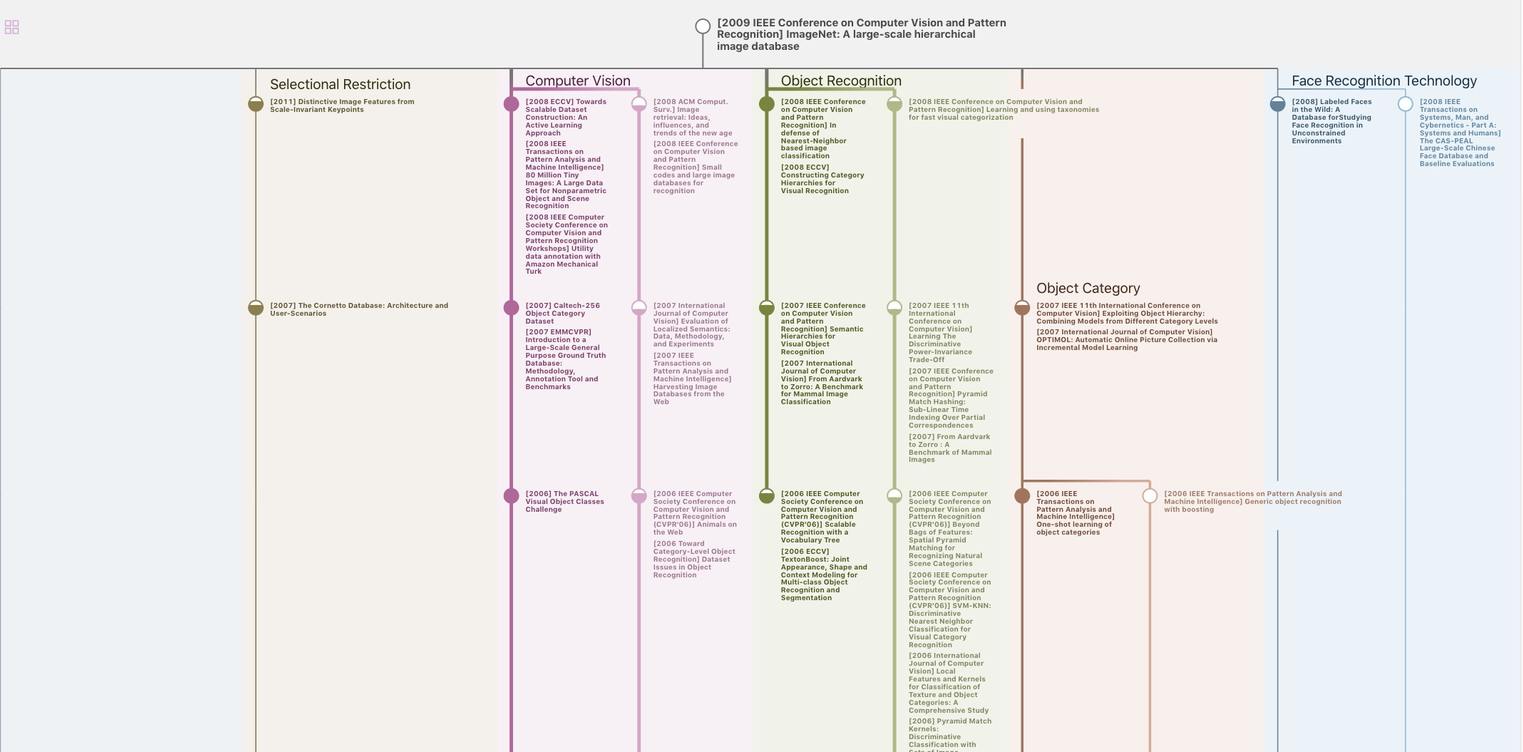
生成溯源树,研究论文发展脉络
Chat Paper
正在生成论文摘要