Combining Contention-Based Spectrum Access and Adaptive Modulation using Deep Reinforcement Learning
arxiv(2023)
摘要
The use of unlicensed spectrum for cellular systems to mitigate spectrum scarcity has led to the development of intelligent adaptive approaches to spectrum access that improve upon traditional carrier sensing and listen-before-talk methods. We study decentralized contention-based medium access for base stations (BSs) of a single Radio Access Technology (RAT) operating on unlicensed shared spectrum. We devise a distributed deep reinforcement learning-based algorithm for both contention and adaptive modulation, modelled on a two state Markov decision process, that attempts to maximize a network-wide downlink throughput objective. Empirically, we find the (proportional fairness) reward accumulated by a policy gradient approach to be significantly higher than even a genie-aided adaptive energy detection threshold. Our approaches are further validated by improved sum and peak throughput. The scalability of our approach to large networks is demonstrated via an improved cumulative reward earned on both indoor and outdoor layouts with a large number of BSs.
更多查看译文
关键词
Adaptive modulation,contention,distributed reinforcement learning,medium access
AI 理解论文
溯源树
样例
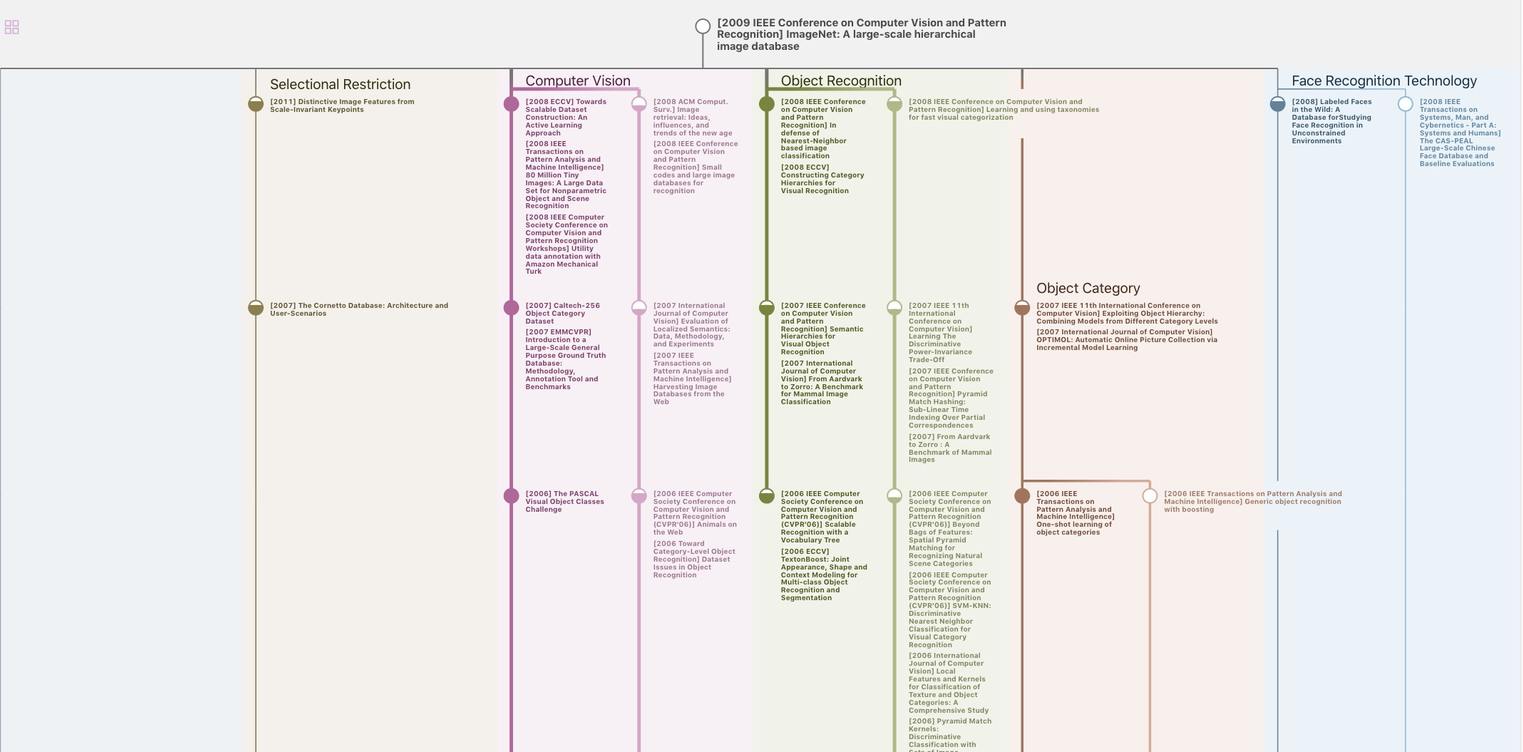
生成溯源树,研究论文发展脉络
Chat Paper
正在生成论文摘要