SPNet: Multi-shell Kernel Convolution for Point Cloud Semantic Segmentation
ADVANCES IN VISUAL COMPUTING (ISVC 2021), PT I(2021)
摘要
Feature encoding is essential for point cloud analysis. In this paper, we propose a novel point convolution operator named Shell Point Convolution (SPConv) for shape encoding and local context learning. Specifically, SPConv splits 3D neighborhood space into shells, aggregates local features on manually designed kernel points, and performs convolution on the shells. Moreover, SPConv incorporates a simple yet effective attention module that enhances local feature aggregation. Based upon SPConv, a deep neural network named SPNet is constructed to process large-scale point clouds. Poisson disk sampling and feature propagation are incorporated in SPNet for better efficiency and accuracy. We provided details of the shell design and conducted extensive experiments on challenging large-scale point cloud datasets. Experimental results show that SPConv is effective in local shape encoding, and our SPNet is able to achieve top-ranking performances in semantic segmentation tasks.
更多查看译文
关键词
Point cloud,Semantic segmentation,Attention,Deep learning
AI 理解论文
溯源树
样例
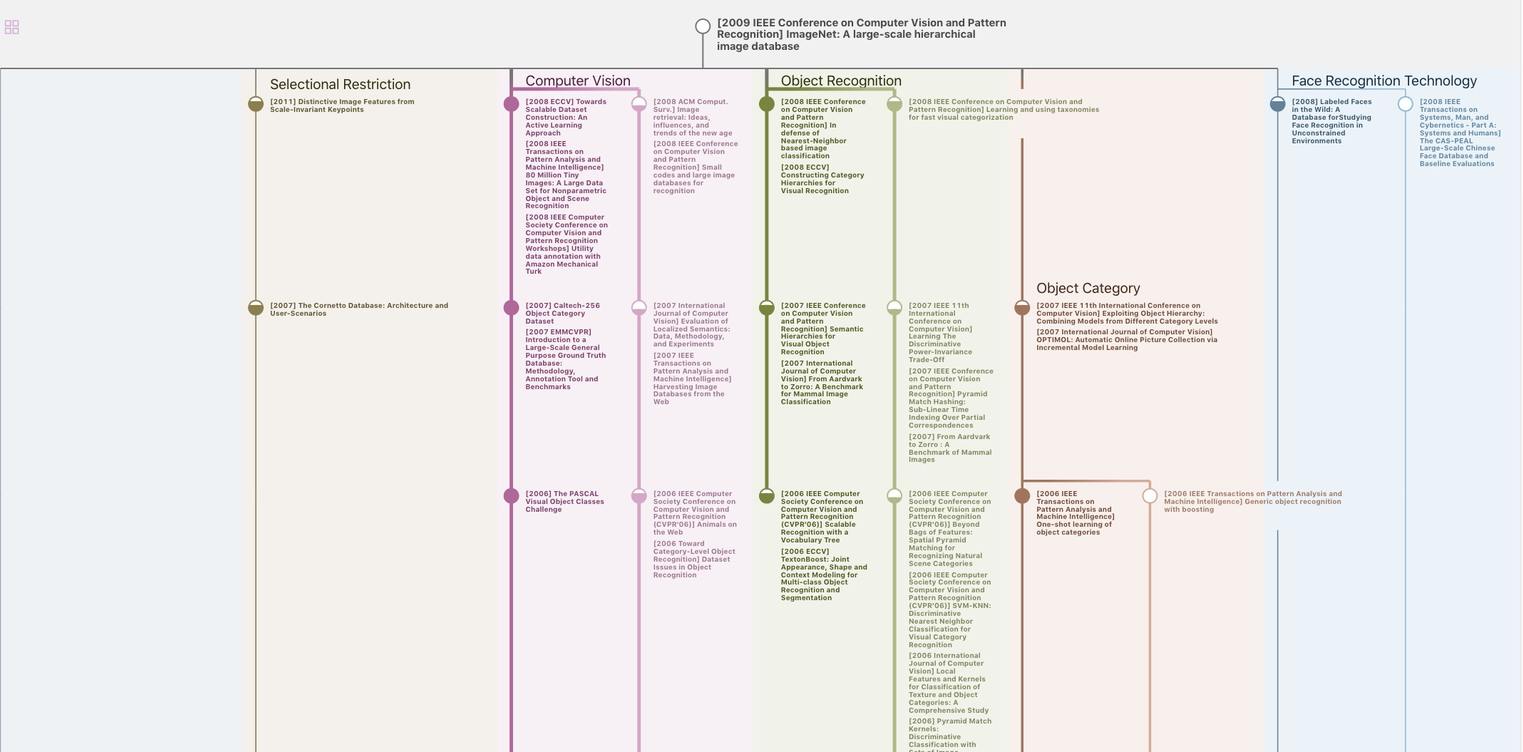
生成溯源树,研究论文发展脉络
Chat Paper
正在生成论文摘要