Enabling Large-Scale Federated Learning over Wireless Edge Networks
2021 IEEE GLOBAL COMMUNICATIONS CONFERENCE (GLOBECOM)(2021)
摘要
Major bottlenecks of large-scale Federated Learning (FL) networks are the high costs for communication and computation. This is due to the fact that most of current FL frameworks only consider a star network topology where all local trained models are aggregated at a single server (e.g., a cloud server). This causes significant overhead at the server when the number of users are huge and local models' sizes are large. This paper proposes a novel edge network architecture which decentralizes the model aggregation process at the server, thereby significantly reducing the aggregation latency of the whole network. In this architecture, we propose a highly-effective in-network computation protocol consisting of two components. First, an in-network aggregation process is designed so that the majority of aggregation computations can be offloaded from cloud server to edge nodes. Second, a joint routing and resource allocation optimization problem is formulated to minimize the aggregation latency for the whole system at every learning round. The problem turns out to be NP-hard, and thus we propose a polynomial time routing algorithm which can achieve near optimal performance with a theoretical bound. Numerical results show that our proposed framework can dramatically reduce the network latency, up to 4.6 times. Furthermore, this framework can significantly decrease cloud's traffic and computing overhead by a factor of K/M, where K is the number of users and M is the number of edge nodes, in comparison with conventional baselines.
更多查看译文
关键词
Mobile Edge Computing, Federated Learning, In-network Computation
AI 理解论文
溯源树
样例
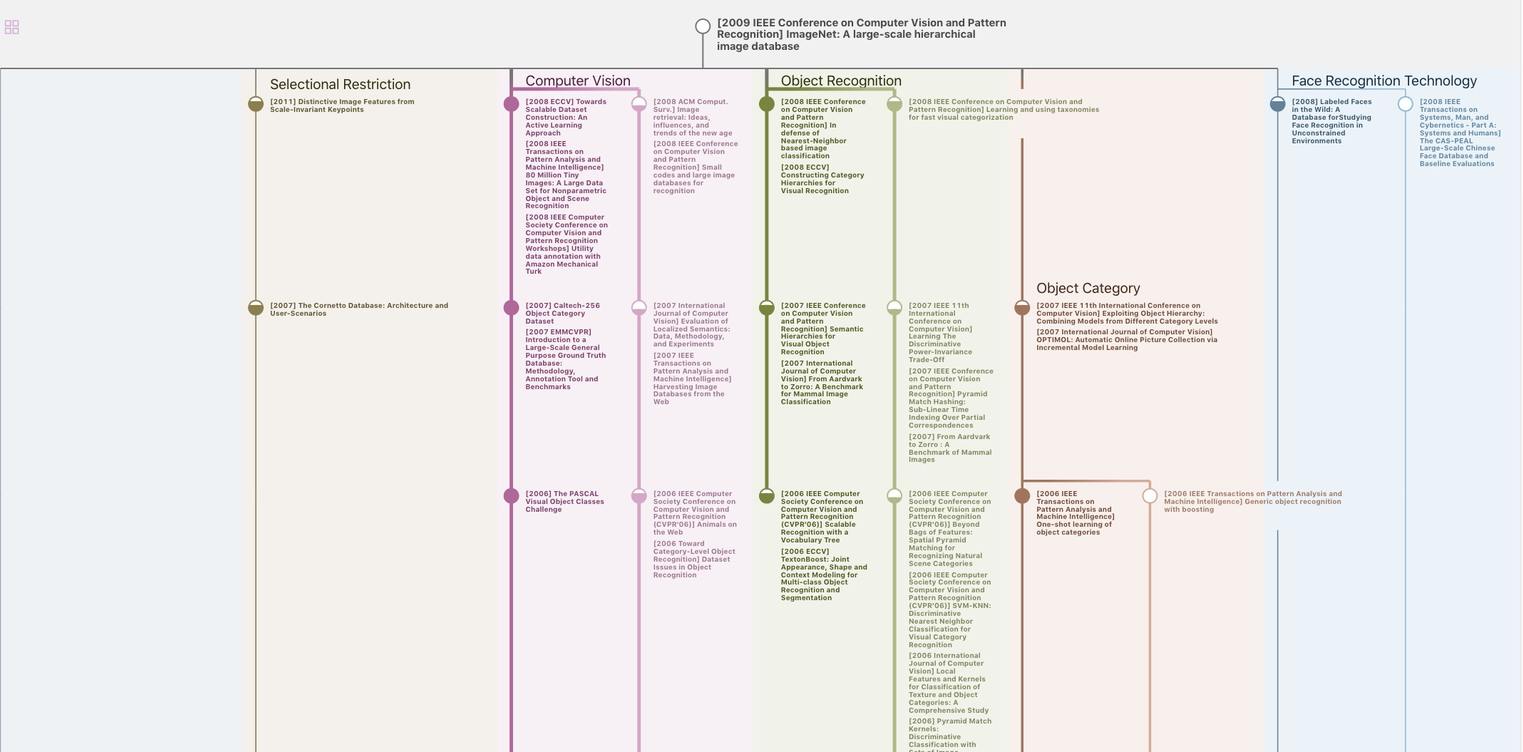
生成溯源树,研究论文发展脉络
Chat Paper
正在生成论文摘要