Robust marginalization of baryonic effects for cosmological inference at the field level
arxiv(2021)
摘要
We train neural networks to perform likelihood-free inference from $(25\,h^{-1}{\rm Mpc})^2$ 2D maps containing the total mass surface density from thousands of hydrodynamic simulations of the CAMELS project. We show that the networks can extract information beyond one-point functions and power spectra from all resolved scales ($\gtrsim 100\,h^{-1}{\rm kpc}$) while performing a robust marginalization over baryonic physics at the field level: the model can infer the value of $\Omega_{\rm m} (\pm 4\%)$ and $\sigma_8 (\pm 2.5\%)$ from simulations completely different to the ones used to train it.
更多查看译文
关键词
cosmological inference,baryonic effects,robust marginalization
AI 理解论文
溯源树
样例
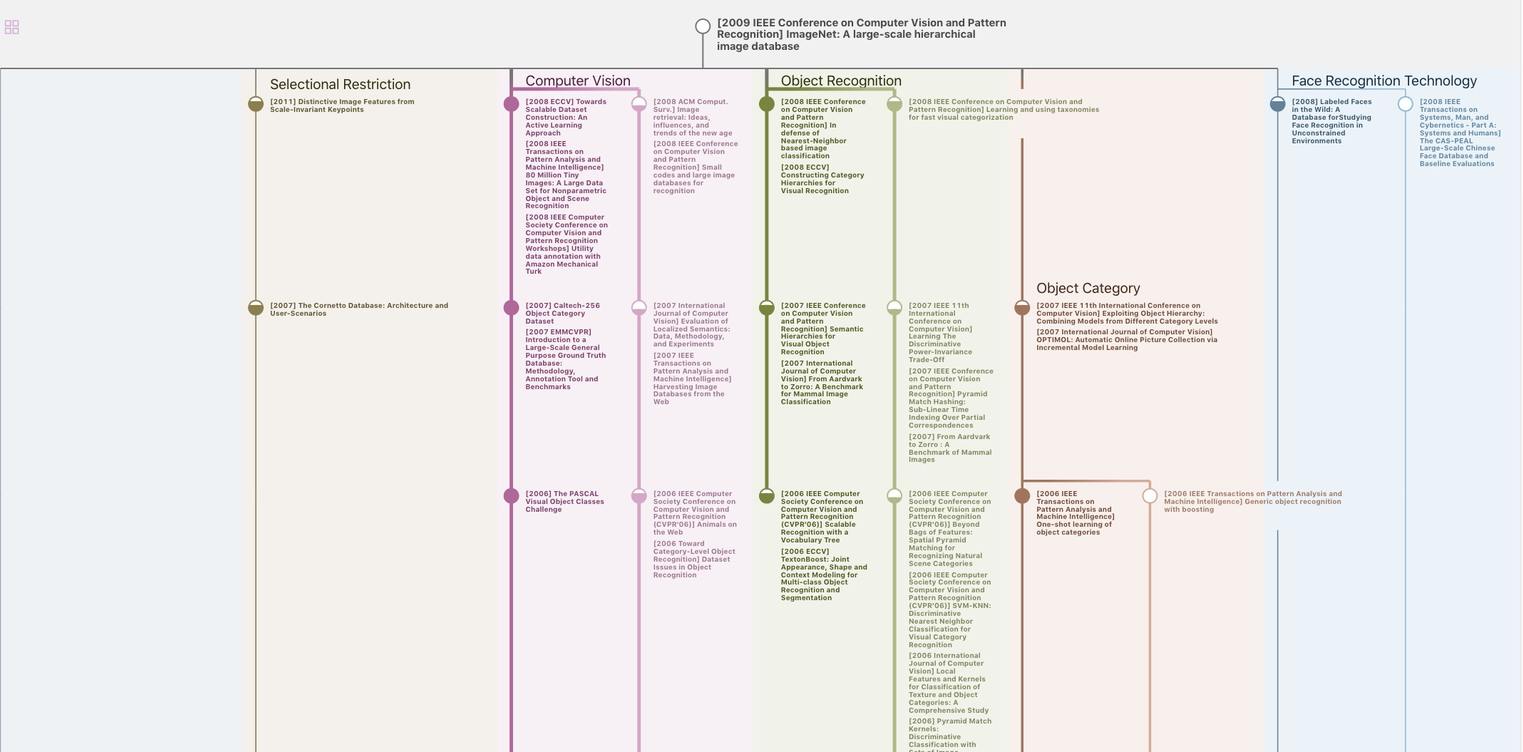
生成溯源树,研究论文发展脉络
Chat Paper
正在生成论文摘要