Negative Interactions for Improved Collaborative Filtering: Don’t go Deeper, go Higher
ACM Conference On Recommender Systems(2021)
摘要
ABSTRACT The recommendation-accuracy of collaborative filtering approaches is typically improved when taking into account higher-order interactions [5, 6, 9, 10, 11, 16, 18, 24, 25, 28, 31, 34, 36, 41, 42, 44]. While deep nonlinear models are theoretically able to learn higher-order interactions, their capabilities were, however, found to be quite limited in practice [5]. Moreover, the use of low-dimensional embeddings in deep networks may severely limit their expressiveness [8]. This motivated us in this paper to explore a simple extension of linear full-rank models that allow for higher-order interactions as additional explicit input-features. Interestingly, we observed that this model-class obtained by far the best ranking accuracies on the largest data set in our experiments, while it was still competitive with various state-of-the-art deep-learning models on the smaller data sets. Moreover, our approach can also be interpreted as a simple yet effective improvement of the (linear) HOSLIM [11] model: by simply removing the constraint that the learned higher-order interactions have to be non-negative, we observed that the accuracy-gains due to higher-order interactions more than doubled in our experiments. The reason for this large improvement was that large positive higher-order interactions (as used in HOSLIM [11]) are relatively infrequent compared to the number of large negative higher-order interactions in the three well-known data-sets used in our experiments. We further characterize the circumstances where the higher-order interactions provide the most significant improvements.
更多查看译文
关键词
collaborative filtering, recommender systems, linear models, higher order interactions
AI 理解论文
溯源树
样例
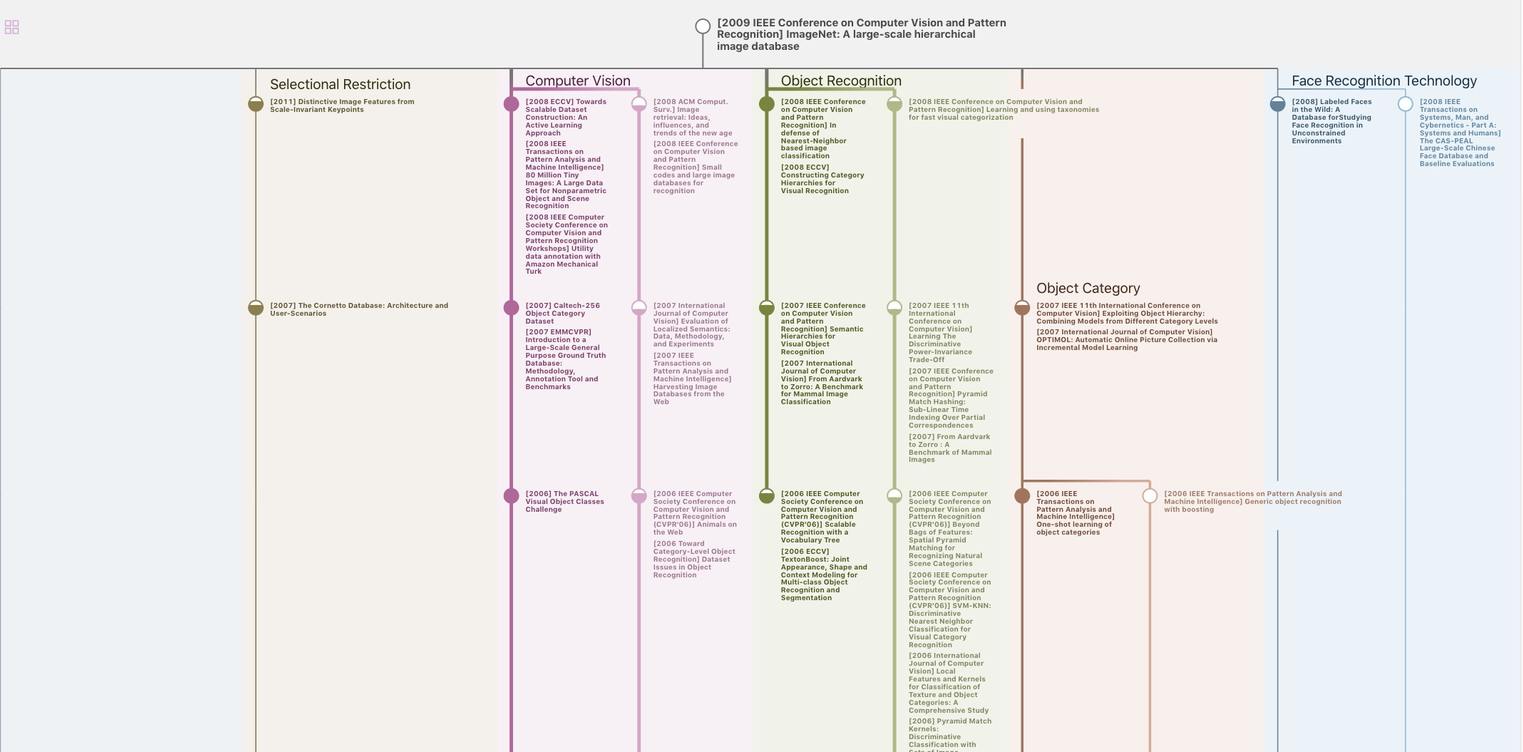
生成溯源树,研究论文发展脉络
Chat Paper
正在生成论文摘要