A Unified Framework For Damaged Image Fusion And Completion Based On Low-Rank And Sparse Decomposition
SIGNAL PROCESSING-IMAGE COMMUNICATION(2021)
摘要
Image fusion can integrate the complementary information of multiple images. However, when the images to be fused are damaged, the existing fusion methods cannot recover the lost information. Matrix completion, on the other hand, can be used to recover the missing information of the image. Therefore, the step-by-step operation of image fusion and completion can fuse the damaged images, but it will cause artifact propagation. In view of this, we develop a unified framework for image fusion and completion. Within this framework, we first assume that the image is superimposed by low-rank and sparse components. To obtain the separation of different components to fuse and restore them separately, we propose a low-rank and sparse dictionary learning model. Specifically, we impose low-rank and sparse constraints on low-rank dictionary and sparse component respectively to improve the discrimination of learned dictionaries and introduce the condition constraints of low-rank and sparse components to promote the separation of different components. Furthermore, we integrate the low-rank characteristic of the image into the decomposition model. Based on this design, the lost information can be recovered with the decomposition of the image without using any additional algorithm. Finally, the maximum l(1)-norm fusion scheme is adopted to merge the coding coefficients of different components. The proposed method can achieve image fusion and completion simultaneously in the unified framework. Experimental results show that this method can well preserve the brightness and details of images, and is superior to the compared methods according to the performance evaluation.
更多查看译文
关键词
Image fusion, Image completion, Image decomposition, Low-rank and sparse representation, Dictionary learning
AI 理解论文
溯源树
样例
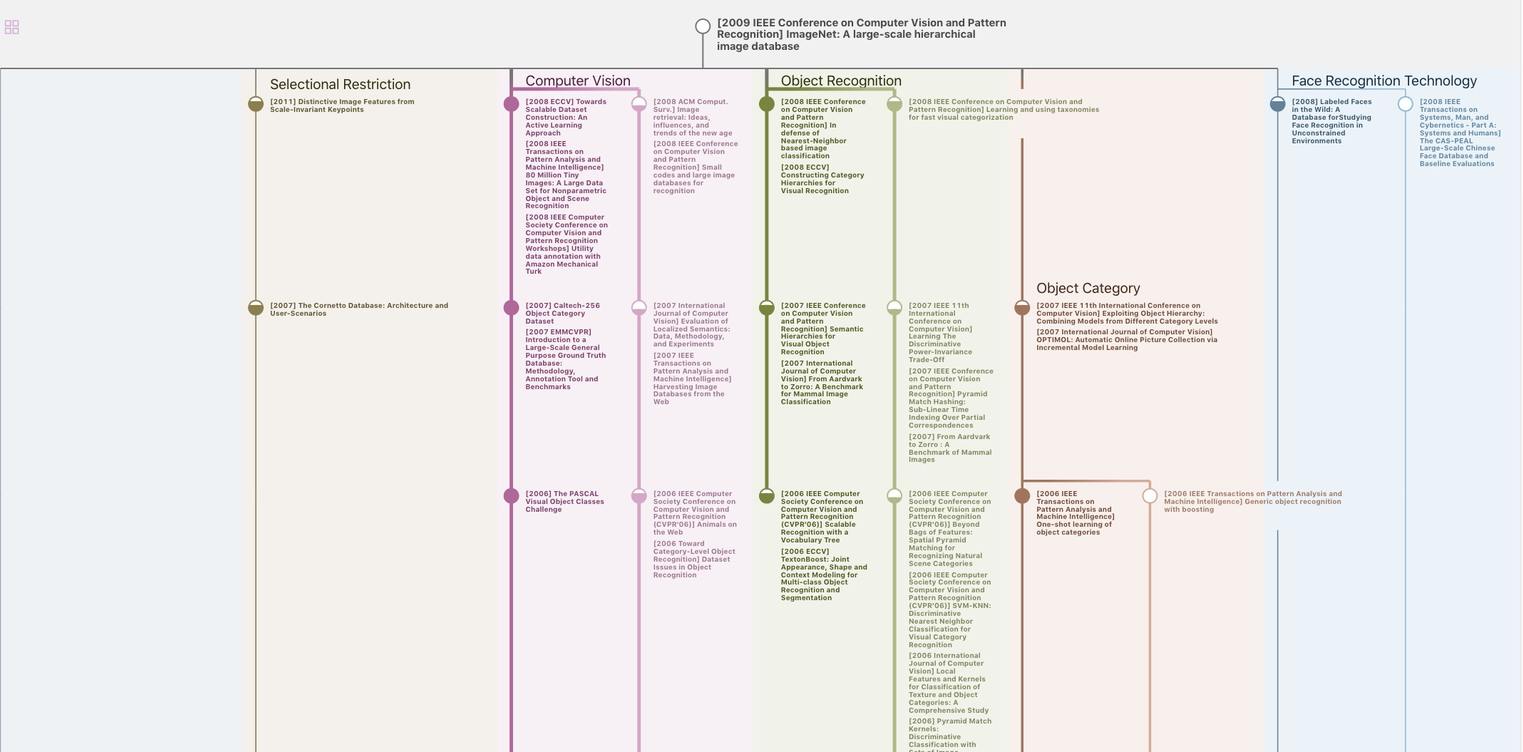
生成溯源树,研究论文发展脉络
Chat Paper
正在生成论文摘要