Transfer Reinforcement Learning for Autonomous Driving: From WiseMove to WiseSim
ACM Transactions on Modeling and Computer Simulation(2021)
摘要
AbstractReinforcement learning (RL) is an attractive way to implement high-level decision-making policies for autonomous driving, but learning directly from a real vehicle or a high-fidelity simulator is variously infeasible. We therefore consider the problem of transfer reinforcement learning and study how a policy learned in a simple environment using WiseMove can be transferred to our high-fidelity simulator, WiseMove. WiseMove is a framework to study safety and other aspects of RL for autonomous driving. WiseMove accurately reproduces the dynamics and software stack of our real vehicle. We find that the accurately modelled perception errors in WiseMove contribute the most to the transfer problem. These errors, when even naively modelled in WiseMove, provide an RL policy that performs better in WiseMove than a hand-crafted rule-based policy. Applying domain randomization to the environment in WiseMove yields an even better policy. The final RL policy reduces the failures due to perception errors from 10% to 2.75%. We also observe that the RL policy has significantly less reliance on velocity compared to the rule-based policy, having learned that its measurement is unreliable.
更多查看译文
关键词
Transfer reinforcement learning, deep reinforcement learning, policy distillation, autonomous driving
AI 理解论文
溯源树
样例
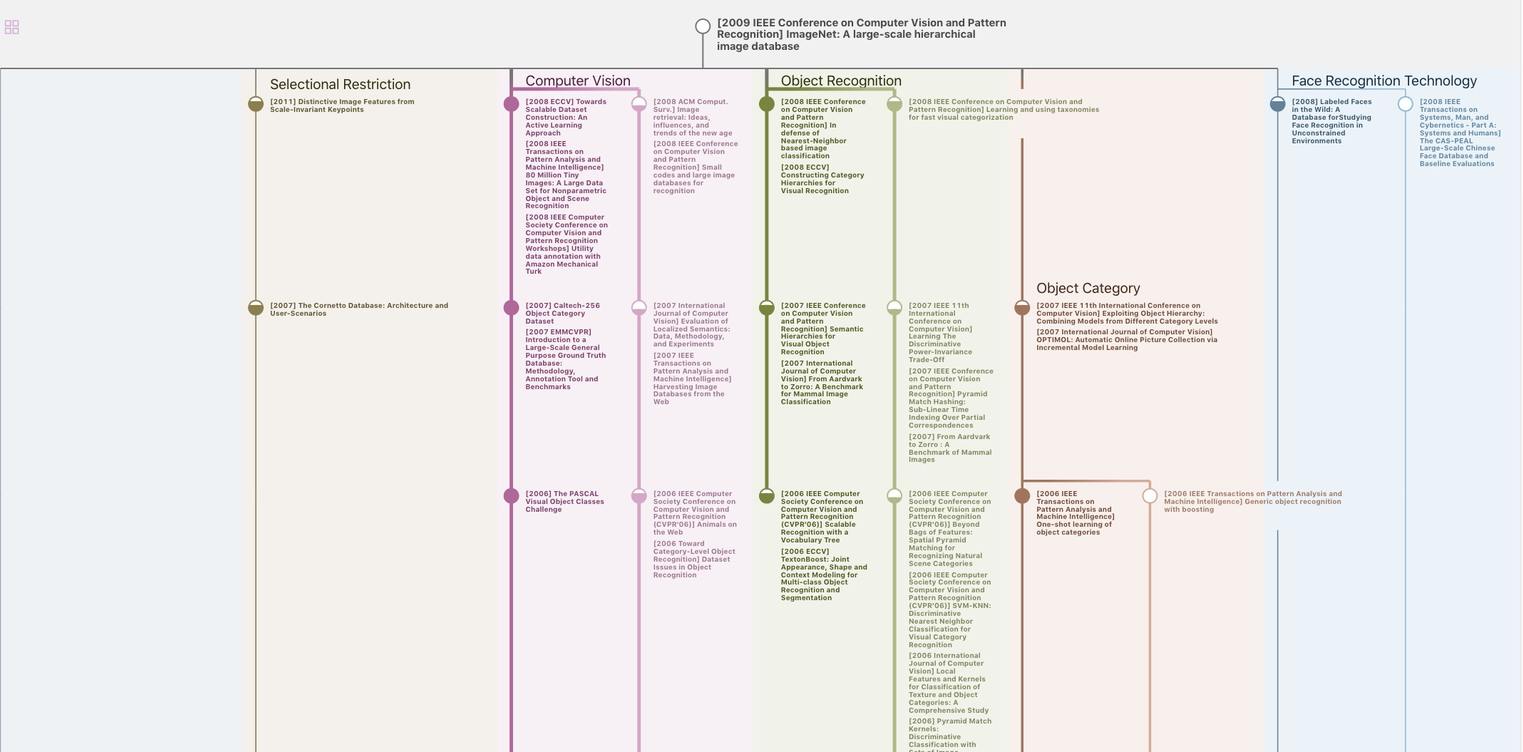
生成溯源树,研究论文发展脉络
Chat Paper
正在生成论文摘要