Short-term traffic prediction using physics-aware neural networks
Transportation Research Part C: Emerging Technologies(2022)
摘要
In this work, we propose an algorithm performing short-term predictions of the flow and speed of vehicles on a stretch of road, using past measurements of these quantities. This algorithm is based on a physics-aware recurrent neural network. A discretization of a macroscopic traffic flow model (using the so-called Traffic Reaction Model) is embedded in the architecture of the network and yields traffic state estimations and predictions for the flow and speed of vehicles, which are physically-constrained by the macroscopic traffic flow model and based on estimated and predicted space–time dependent traffic parameters. These parameters are themselves obtained using a succession of LSTM recurrent neural networks. The algorithm is tested on raw flow measurements obtained from loop detectors.
更多查看译文
关键词
Traffic reaction model,Macroscopic traffic flow model,Traffic prediction,Recurrent neural networks,LSTM
AI 理解论文
溯源树
样例
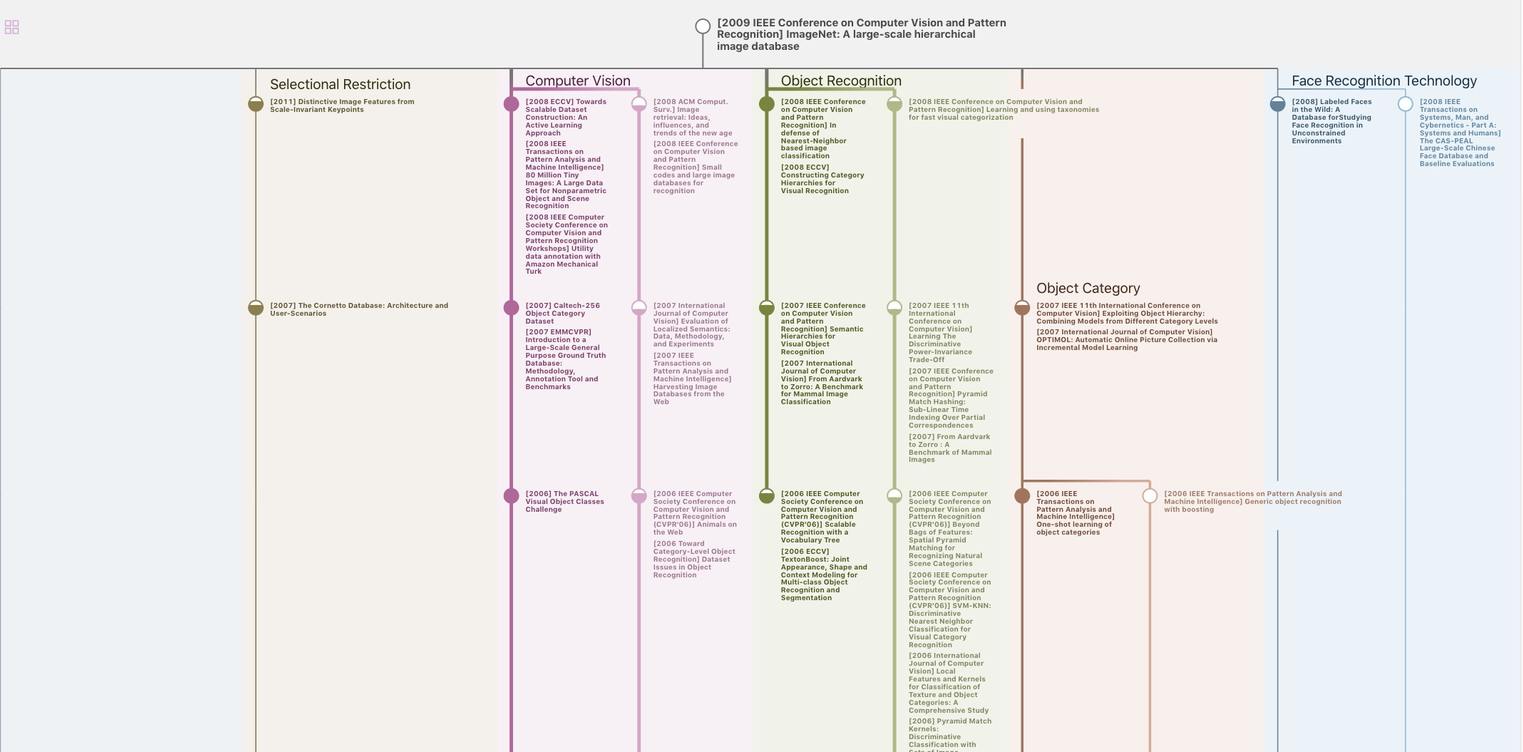
生成溯源树,研究论文发展脉络
Chat Paper
正在生成论文摘要