Combining Rules and Embeddings via Neuro-Symbolic AI for Knowledge Base Completion
arxiv(2021)
摘要
Recent interest in Knowledge Base Completion (KBC) has led to a plethora of approaches based on reinforcement learning, inductive logic programming and graph embeddings. In particular, rule-based KBC has led to interpretable rules while being comparable in performance with graph embeddings. Even within rule-based KBC, there exist different approaches that lead to rules of varying quality and previous work has not always been precise in highlighting these differences. Another issue that plagues most rule-based KBC is the non-uniformity of relation paths: some relation sequences occur in very few paths while others appear very frequently. In this paper, we show that not all rule-based KBC models are the same and propose two distinct approaches that learn in one case: 1) a mixture of relations and the other 2) a mixture of paths. When implemented on top of neuro-symbolic AI, which learns rules by extending Boolean logic to real-valued logic, the latter model leads to superior KBC accuracy outperforming state-of-the-art rule-based KBC by 2-10% in terms of mean reciprocal rank. Furthermore, to address the non-uniformity of relation paths, we combine rule-based KBC with graph embeddings thus improving our results even further and achieving the best of both worlds.
更多查看译文
关键词
knowledge base completion,ai,embeddings,neuro-symbolic
AI 理解论文
溯源树
样例
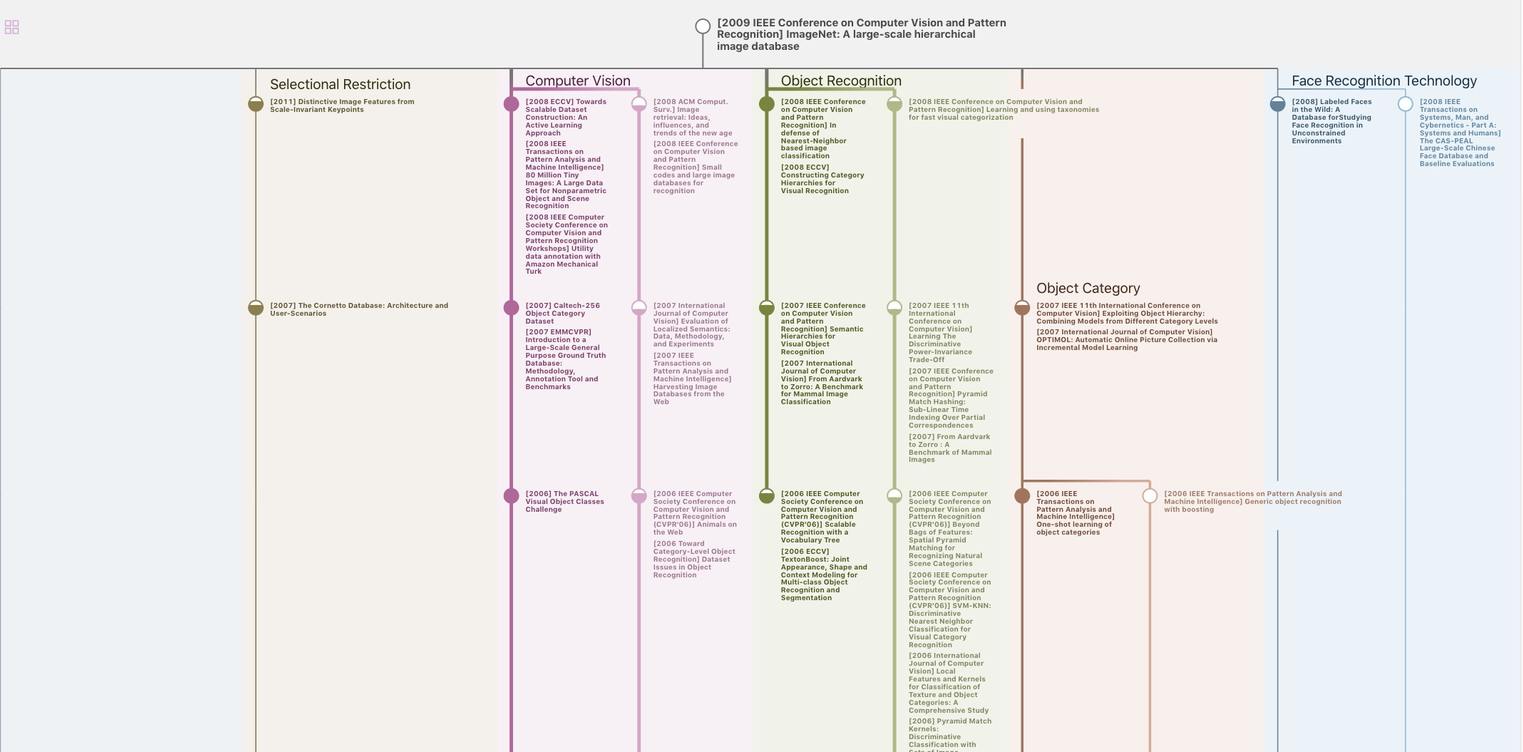
生成溯源树,研究论文发展脉络
Chat Paper
正在生成论文摘要