Accelerated Stochastic Gradient for Nonnegative Tensor Completion and Parallel Implementation
29TH EUROPEAN SIGNAL PROCESSING CONFERENCE (EUSIPCO 2021)(2021)
摘要
We consider the problem of nonnegative tensor completion. We adopt the alternating optimization framework and solve each nonnegative matrix completion problem via a stochastic variation of the accelerated gradient algorithm. We experimentally test the effectiveness and the efficiency of our algorithm using both real-world and synthetic data. We develop a shared-memory implementation of our algorithm using the multi-threaded API OpenMP, which attains significant speedup. We believe that our approach is a very competitive candidate for the solution of very large nonnegative tensor completion problems.
更多查看译文
关键词
tensors, stochastic gradient, nonnegative tensor completion, optimal first-order optimization algorithms, parallel algorithms, OpenMP
AI 理解论文
溯源树
样例
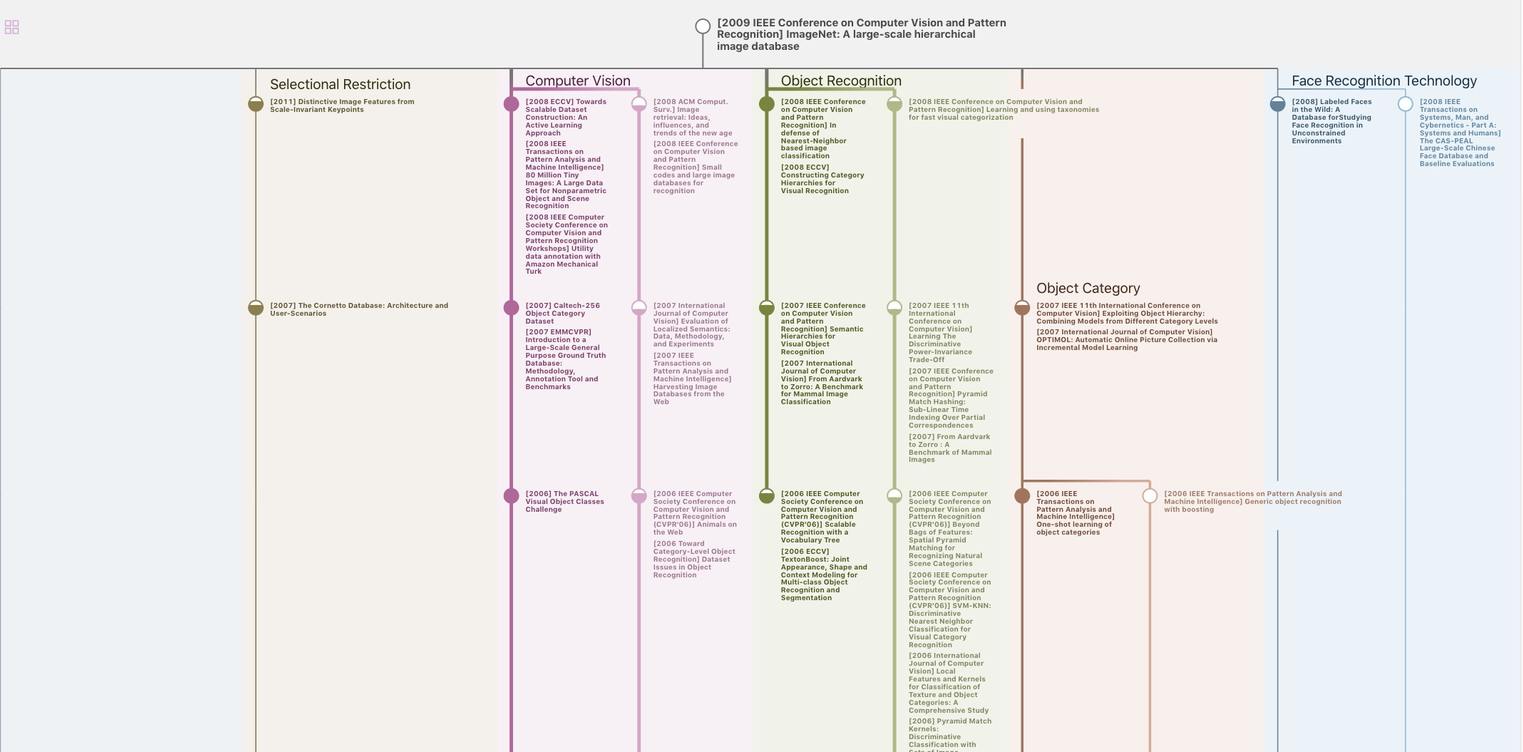
生成溯源树,研究论文发展脉络
Chat Paper
正在生成论文摘要