Interpreting machine learning functions as physical observables
arxiv(2021)
摘要
We propose to interpret machine learning functions as physical observables, opening up the possibility to apply "standard" statistical-mechanical methods to outputs from neural networks. This includes histogram reweighting and finite-size scaling, to analyse phase transitions quantitatively. In addition we incorporate predictive functions as conjugate variables coupled to an external field within the Hamiltonian of a system, allowing to induce order-disorder phase transitions in a novel manner. A noteworthy feature of this approach is that no knowledge of the symmetries in the Hamiltonian is required.
更多查看译文
关键词
machine learning functions,physical observables
AI 理解论文
溯源树
样例
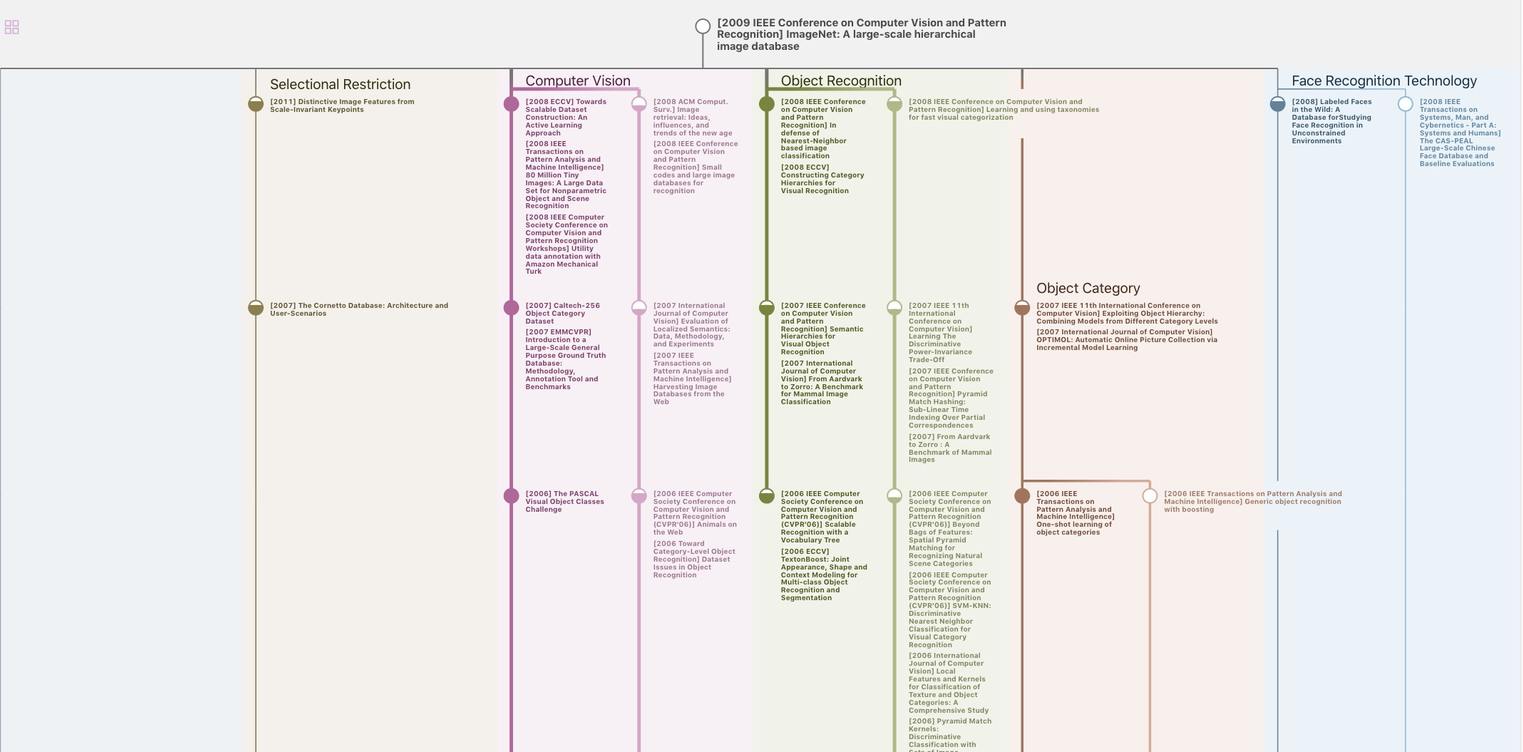
生成溯源树,研究论文发展脉络
Chat Paper
正在生成论文摘要