Unsupervised Learning of Joint Embeddings for Node Representation and Community Detection
MACHINE LEARNING AND KNOWLEDGE DISCOVERY IN DATABASES, ECML PKDD 2021: RESEARCH TRACK, PT II(2021)
摘要
In graph analysis community detection and node representation learning are two highly correlated tasks. In this work, we propose an efficient generative model called J-ENC for learning Joint Embedding for Node representation and Community detection. J-ENC learns a community-aware node representation, i.e., learning of the node embeddings are constrained in such a way that connected nodes are not only "closer" to each other but also share similar community assignments. This joint learning framework leverages community-aware node embeddings for better performance on these tasks: node classification, overlapping community detection and non-overlapping community detection. We demonstrate on several graph datasets that J-ENC effectively outperforms many competitive baselines on these tasks. Furthermore, we show that J-ENC not only has quite robust performance with varying hyper-parameters but also is computationally efficient than its competitors.
更多查看译文
关键词
community detection,joint embeddings,node representation,unsupervised learning
AI 理解论文
溯源树
样例
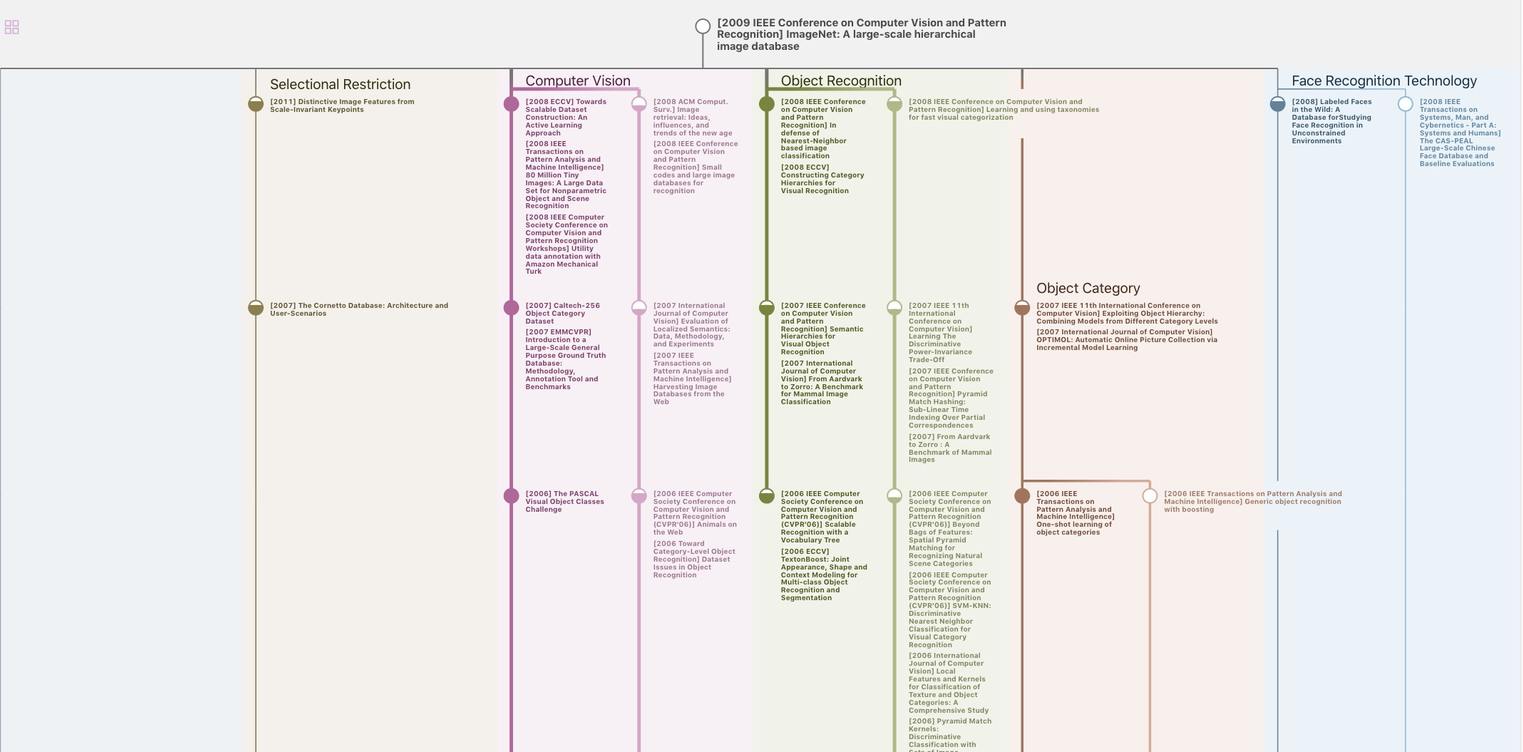
生成溯源树,研究论文发展脉络
Chat Paper
正在生成论文摘要