Gaussian Process Encoders: VAEs with Reliable Latent-Space Uncertainty
MACHINE LEARNING AND KNOWLEDGE DISCOVERY IN DATABASES, ECML PKDD 2021: RESEARCH TRACK, PT II(2021)
摘要
Variational autoencoders are a versatile class of deep latent variable models. They learn expressive latent representations of high dimensional data. However, the latent variance is not a reliable estimate of how uncertain the model is about a given input point. We address this issue by introducing a sparse Gaussian process encoder. The Gaussian process leads to more reliable uncertainty estimates in the latent space. We investigate the implications of replacing the neural network encoder with a Gaussian process in light of recent research. We then demonstrate how the Gaussian Process encoder generates reliable uncertainty estimates while maintaining good likelihood estimates on a range of anomaly detection problems. Finally, we investigate the sensitivity to noise in the training data and show how an appropriate choice of Gaussian process kernel can lead to automatic relevance determination.
更多查看译文
关键词
Variational Autoencoder, Uncertainty estimation, Anomaly detection, Gaussian process
AI 理解论文
溯源树
样例
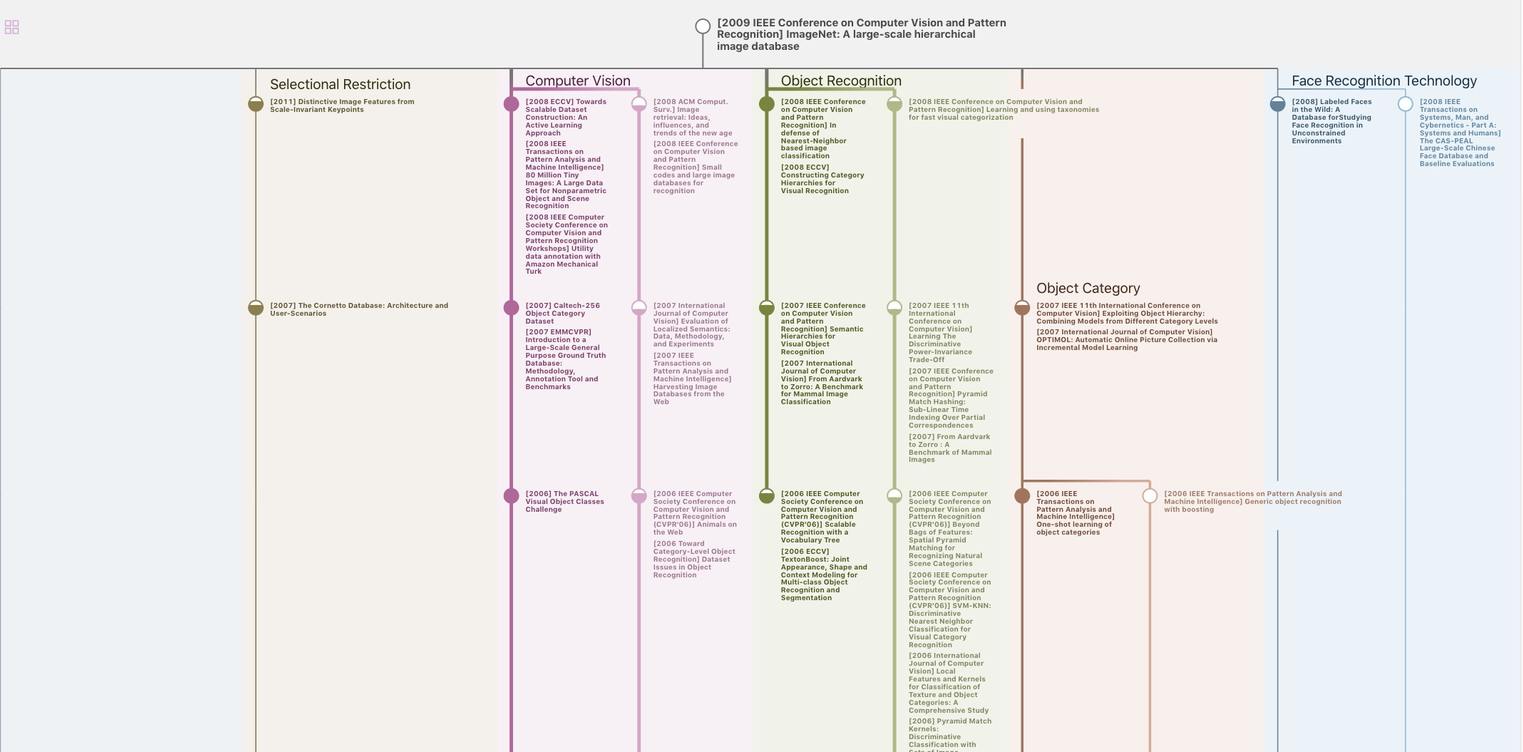
生成溯源树,研究论文发展脉络
Chat Paper
正在生成论文摘要