Checking Robustness of Representations Learned by Deep Neural Networks
MACHINE LEARNING AND KNOWLEDGE DISCOVERY IN DATABASES, ECML PKDD 2021: APPLIED DATA SCIENCE TRACK, PT V(2021)
摘要
Recent works have shown the vulnerability of deep neural networks to adversarial or out-of-distribution examples. This weakness may come from the fact that training deep models often leads to extracting spurious correlations between image classes and some characteristics of images used for training. As demonstrated, popular, ready-to-use models like the ResNet or the EfficientNet may rely on the non-obvious and counterintuitive features. Detection of these weaknesses is often difficult as classification accuracy is excellent and does not indicate that the model is non-robust. To address this problem, we propose a new method and a measure called robustness score. The method allows indicating which classes are recognized by the deep model using non-robust representations, i.e. representations based on spurious correlations. Since the root of this problem lies in the quality of the training data, our method allows us to analyze the training dataset in terms of the existence of these non-obvious spurious correlations. This knowledge can be used to attack the model by finding adversarial images. Consequently, our method can expose threats to the model's reliability, which should be addressed to increase the certainty of classification decisions. The method was verified using the ImageNet and Pascal VOC datasets, revealing many flaws that affect the final quality of deep models trained on these datasets.
更多查看译文
关键词
Deep neural networks, Robust representations, Spurious correlations, Explainable AI
AI 理解论文
溯源树
样例
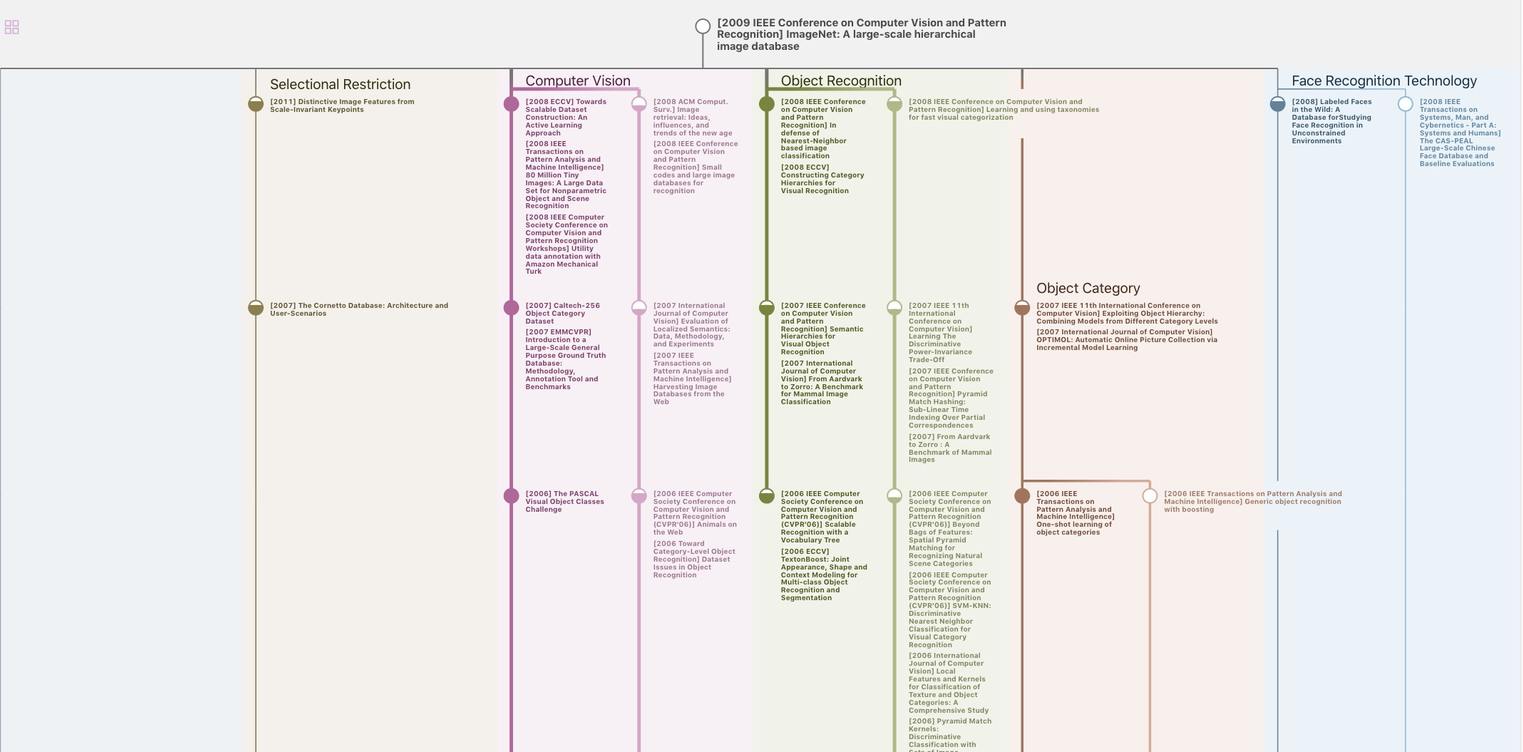
生成溯源树,研究论文发展脉络
Chat Paper
正在生成论文摘要