Comparing Traditional and Neural Approaches for Detecting Health-Related Misinformation.
CLEF(2021)
摘要
Detecting health-related misinformation is a research challenge that has recently received increasing attention. Helping people to find credible and accurate health information on the Web remains an open research issue as has been highlighted during the COVID-19 pandemic. However, in such scenarios, it is often critical to detect misinformation quickly [ 34 ], which implies working with little data, at least at the beginning of the spread of such information. In this work, we present a comparison between different automatic approaches of identifying misinformation, and we compare how they behave for different tasks and with limited training data. We experiment with traditional algorithms, such as SVMs or KNNs, as well as newer BERT-based models [ 5 ]. Our experiments utilise the CLEF 2018 Consumer Health Search task dataset [ 16 ] to perform experiments on detecting untrustworthy contents and information that is difficult to read. Our results suggest that traditional models are still a strong baseline for these challenging tasks. In the absence of substantive training data, classical approaches tend to outperform BERT-based models.
更多查看译文
关键词
Health-related content, Misinformation, Language, Neural approaches
AI 理解论文
溯源树
样例
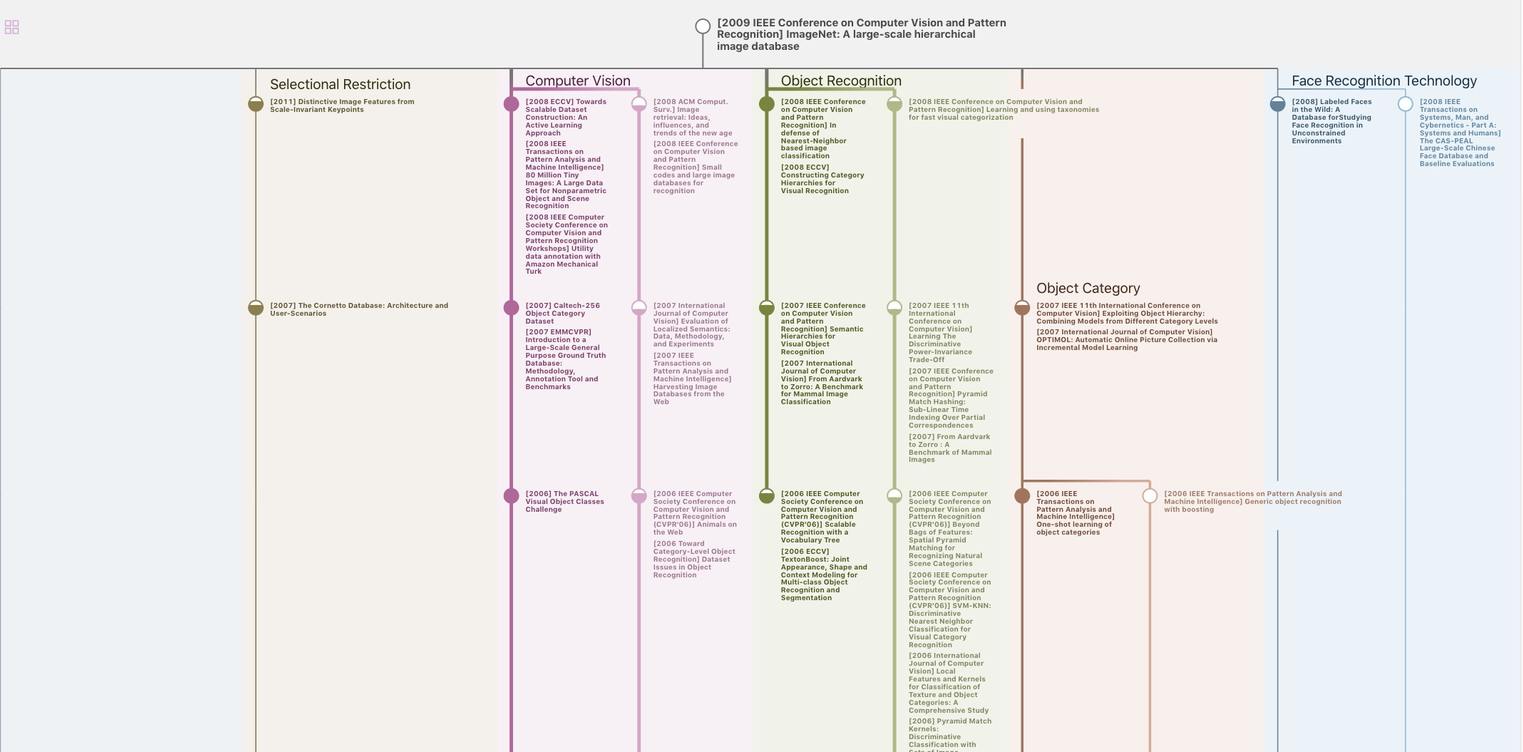
生成溯源树,研究论文发展脉络
Chat Paper
正在生成论文摘要