An Automatic Computer-Aided Diagnosis System Based On The Multimodal Fusion Of Breast Cancer (Mf-Cad)
BIOMEDICAL SIGNAL PROCESSING AND CONTROL(2021)
摘要
The risk of death incurred by breast cancer is rising exponentially, especially among women. The early breast cancer diagnosis before it metastasizes helps medical staff controlling this disease, which decreases the risk of death. This made early breast cancer detection a crucial problem. Different imaging modalities offer complementary information concerning the same lesion helps to increase the performance of thcy fusing several modalities. This paper proposes a computerized features classification of breast cancer lesions through both the Dynamic Contrast-Enhanced Magnetic Resonance Imaging (DCE-MRI) and Digital Mammographic images (MGs). This study aims to investigate a Multimodal Fusion-based Computer-Aided Diagnosis (CAD) system, called MFCAD, based on multivariate analysis of different modalities, for breast cancer mass detection. In this paper, firstly a new local feature descriptor is proposed in feature extraction, namely, the Gradient Local Information Pattern (GLIP), where we consider the gradient magnitude and orientation as well as the local differences as local binary features for DCE-MRI (or MGs) modality. Secondly, the fusion scheme is conducted using the Canonical Correlation Analysis (CCA) to highlight the intrinsic relation between these modalities. Finally, for comparative purposes, several selected machine learning classifiers (K-Nearest Neighbors, Support Vector Machine, Random forests, Artificial Neural Networks and Radial Basis Function Neural Network (RBFNN)) are used to distinguish between mass and No-mass breast images.Evaluation experiments of the diagnostic performances of our MF-CAD system are conducted over private datasets that contain both MG and DCE-MRI images acquired from 286 patients, which are "Breast DCE-MRI", "Breast-MG" and "Breast Multimodal" datasets. Experimental results of the proposed MF-CAD system achieved an Area Under the ROC Curve (AUC) value of 99.10% using RBFNN classifier, while for each single modality alone, the best AUC values of 97.20% and 93.50% are obtained respectively for MG and DCE-MRI modalities using random forest classifier. A comparative study with recent existing approaches shows the competitive performances of the proposed approach.
更多查看译文
关键词
Breast cancer, Multimodal fusion, Computer-aided diagnosis, Digital mammographic images, Dynamic contrast-enhanced magnetic reso-nance imaging, Gradient local information pattern descriptor, Canonical correlation analysis
AI 理解论文
溯源树
样例
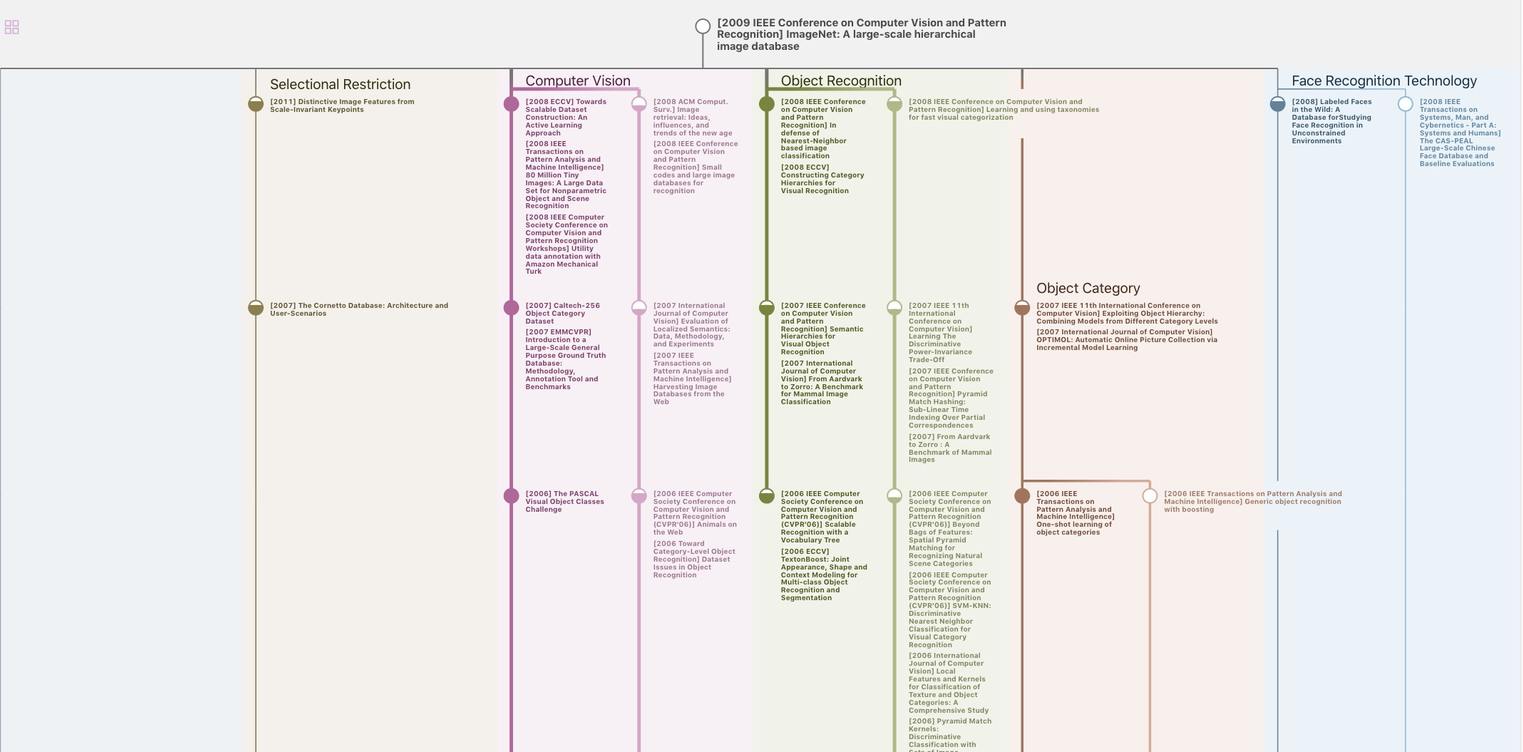
生成溯源树,研究论文发展脉络
Chat Paper
正在生成论文摘要