Improvement Of Automatic Ischemic Stroke Lesion Segmentation In Ct Perfusion Maps Using A Learned Deep Neural Network
COMPUTERS IN BIOLOGY AND MEDICINE(2021)
摘要
Acute ischemic stroke is one of the leading causes of death and long-term disability worldwide. It occurs when a blood clot blocks an artery that supplies blood to the brain tissue. Segmentation of acute ischemic stroke lesions plays a vital role to improve diagnosis, outcome assessment, and treatment planning. The current standard approach of ischemic stroke lesion segmentation is simply thresholding the Computed Tomography Perfusion (CTP) maps, i.e., quantitative feature maps created by summarizing CTP time sequence scans. However, this approach is not precise enough (its Dice similarity score is only around 50%) to be used in practice. Numerous machine learning-based techniques have recently been proposed to improve the accuracy of ischemic stroke lesion segmentation. Although they have achieved remarkable results, they still need to be improved before they can be used in actual practice. This paper presents a novel deep learning-based technique, MutiRes U-Net, for the segmentation of ischemic stroke lesions in CTP maps. MultiRes U-Net is a modified version of the original U-Net that is re-designed to be robust to segment the objects in different scales and unusual appearances. Additionally, in this paper, we propose to enrich the input CTP maps by using their contra-lateral and corresponding Tmax images. We evaluated the proposed method using the ISLES challenge 2018 dataset. As compared to the state-ofthe-art methods, the results show an improvement in segmentation task accuracy. The dice similarity score (DSC) was 68%, the Jaccard score was 57.13%, and the mean absolute volume error was 22.62(ml).
更多查看译文
关键词
Ischemic stroke lesion segmentation, Computed tomography perfusion (CTP), Enriched CTP maps, MultiRes U-Net
AI 理解论文
溯源树
样例
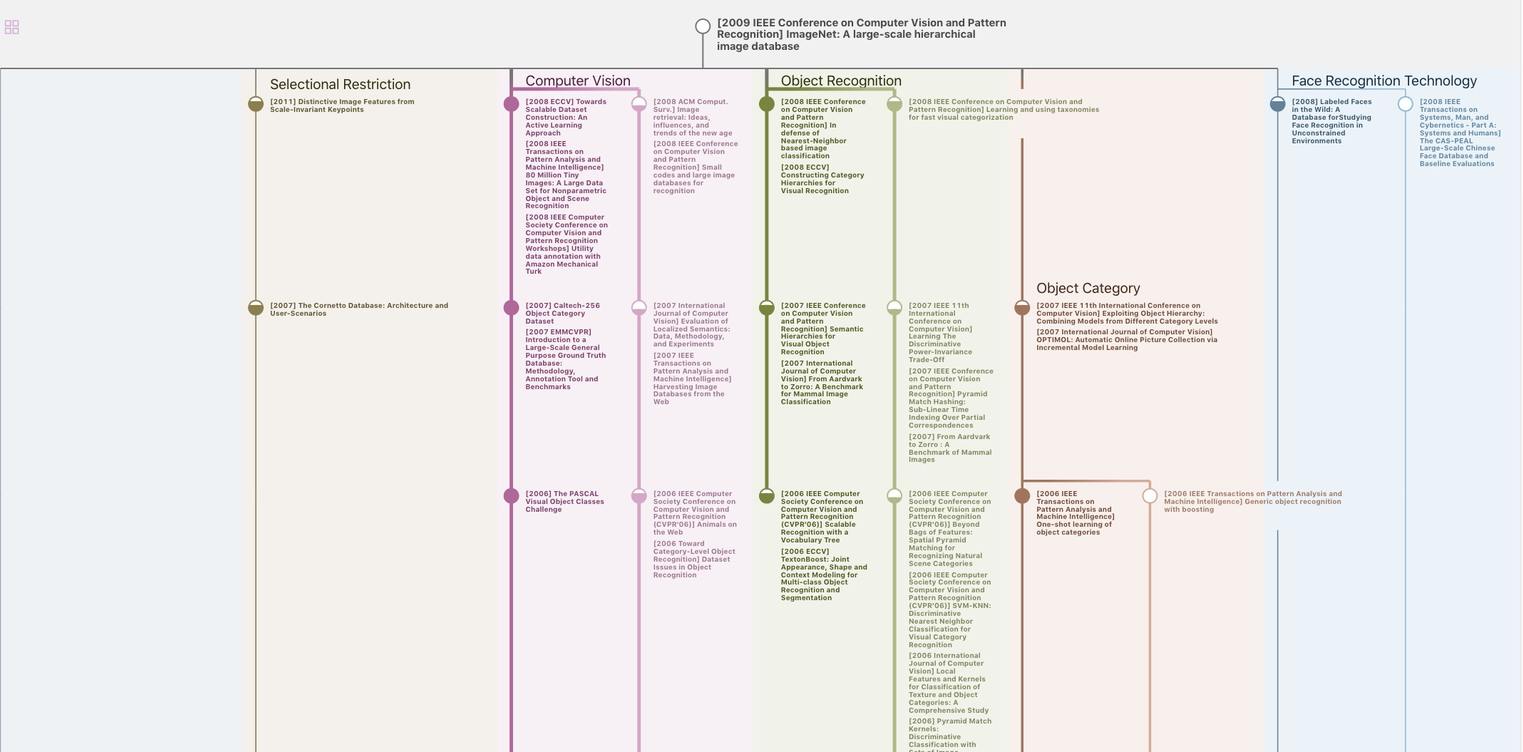
生成溯源树,研究论文发展脉络
Chat Paper
正在生成论文摘要