A Verifiable Federated Learning Scheme Based on Secure Multi-party Computation
WIRELESS ALGORITHMS, SYSTEMS, AND APPLICATIONS, WASA 2021, PT II(2021)
摘要
Federated learning ensures that the quality of the model is uncompromised while the resulting global model is consistent with the model trained by directly collecting user data. However, the risk of inferring data considered in federated learning. Furthermore, the inference to the learning outcome considered in a federated learning environment must satisfy that data cannot be inferred from any outcome except the owner of the data. In this paper, we propose a new federated learning scheme based on secure multi-party computation (SMC) and differential privacy. The scheme prevents inference during the learning process as well as inference of the output. Meanwhile, the scheme protects the user's local data during the learning process to ensure the correctness of the results after users' midway exits through the process.
更多查看译文
关键词
Federated learning, Secure multi-party computation, Differential privacy, Verification
AI 理解论文
溯源树
样例
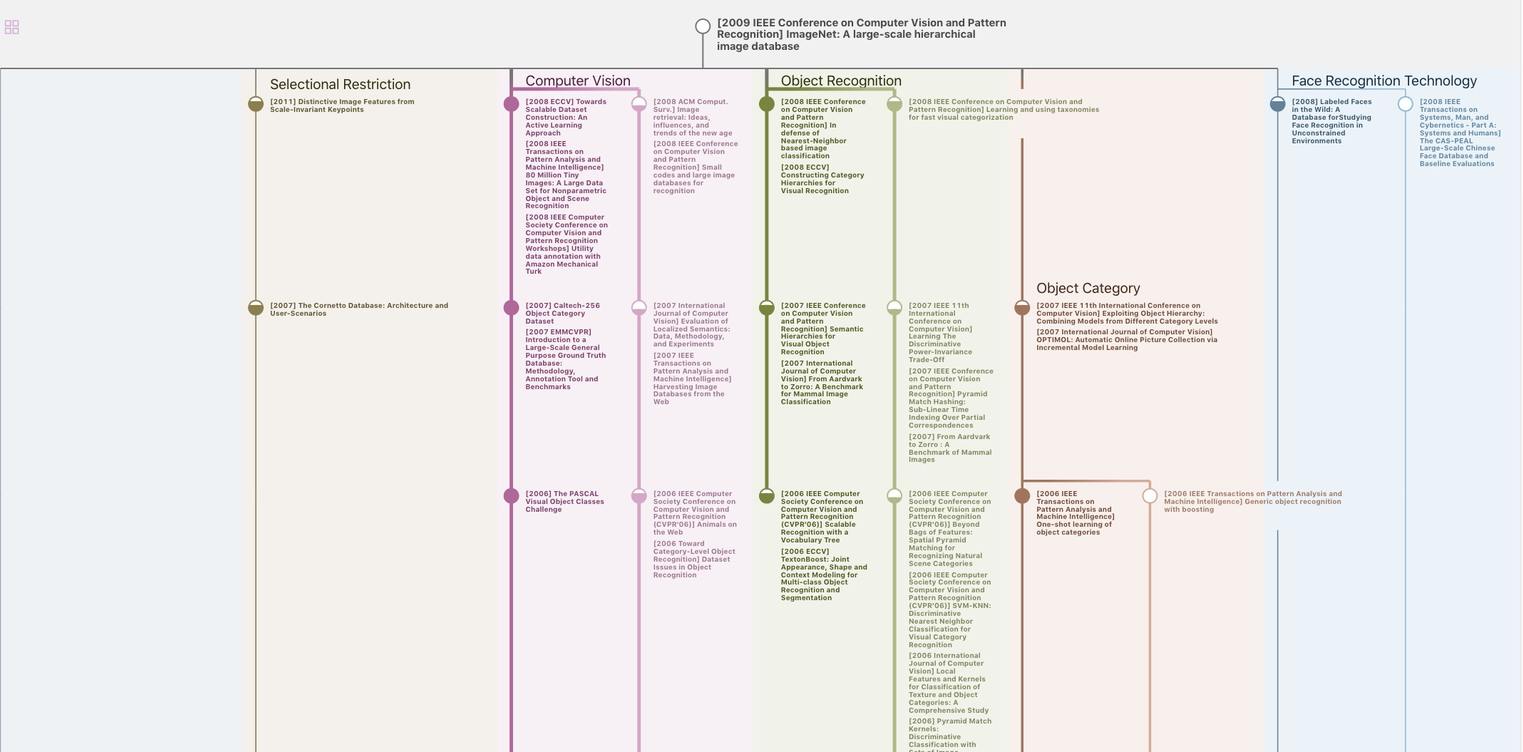
生成溯源树,研究论文发展脉络
Chat Paper
正在生成论文摘要