ParkLSTM: Periodic Parking Behavior Prediction Based on LSTM with Multi-source Data for Contract Parking Spaces
WIRELESS ALGORITHMS, SYSTEMS, AND APPLICATIONS, WASA 2021, PT II(2021)
摘要
With the rapid development of urbanization and the swift rising of the number of vehicles in cities, the process of finding a suitable parking space not only wastes a lot of time but also indirectly aggravates the problem of traffic congestion. To assist the decision-making and alleviate the pain of parking, researchers propose a variety of methods to improve the parking efficiency of existing parking lots. Different from existing studies, we address the parking issue from an incremental rather than a stock perspective. In this paper, we propose a LSTM-based prediction model to make full use of contract parking spaces, which are characterized by the periodic departure time and complementary to the idle space during the peak period of the city. In addition, we utilize multi-source data as the input to improve the prediction performance. We evaluate our model on real-world parking data involved with nearly 14 million parking records in Wuhan. The experimental results show that the average accuracy of the ParkLSTM prediction reaches 91.091%, which is 11.19%-19.70% higher than other parking behavior prediction models.
更多查看译文
关键词
Intelligent transportation, Contract parking spaces, Multi-source data, Periodic, LSTM
AI 理解论文
溯源树
样例
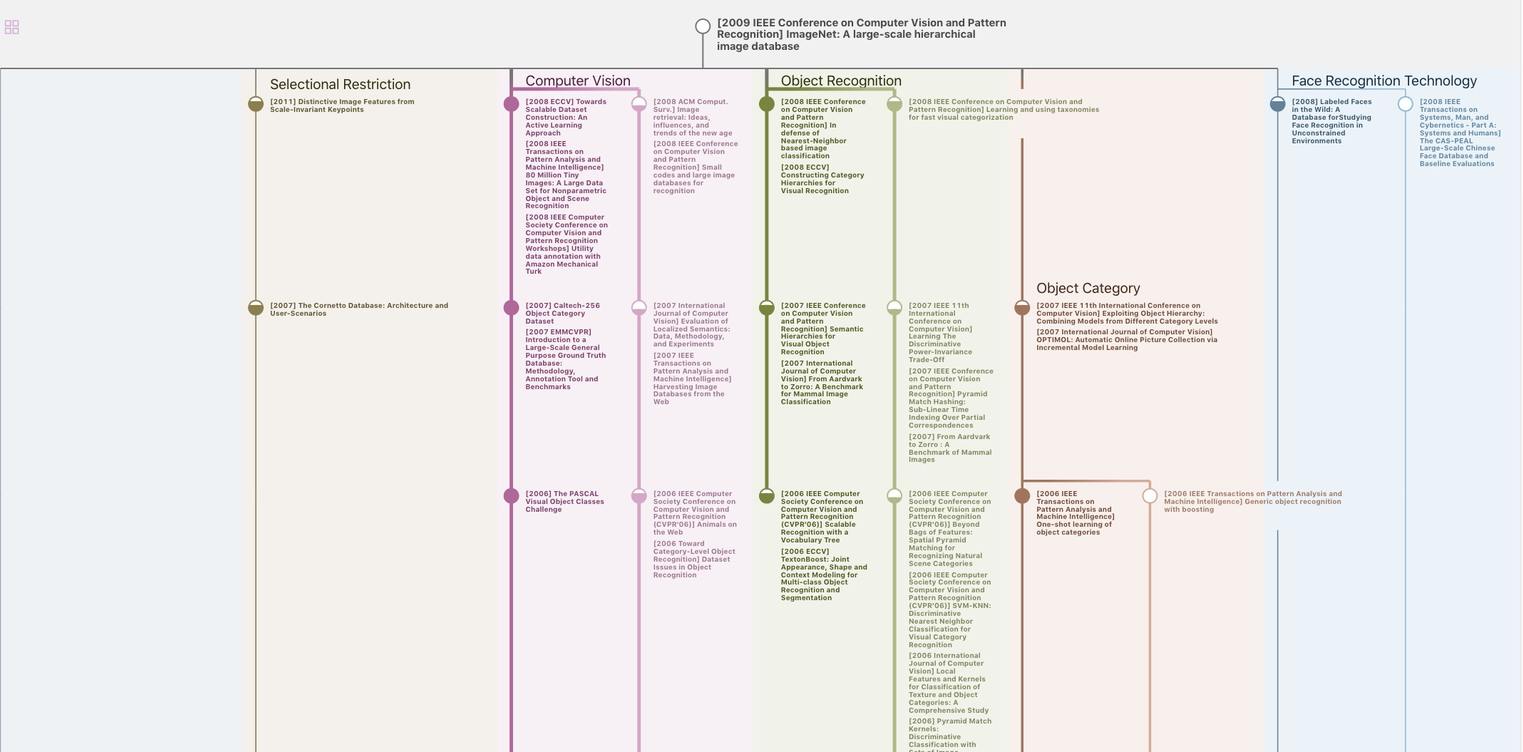
生成溯源树,研究论文发展脉络
Chat Paper
正在生成论文摘要