Cognition-Enhanced Machine Learning for Better Predictions with Limited Data
TOPICS IN COGNITIVE SCIENCE(2022)
摘要
The fields of machine learning (ML) and cognitive science have developed complementary approaches to computationally modeling human behavior. ML's primary concern is maximizing prediction accuracy; cognitive science's primary concern is explaining the underlying mechanisms. Cross-talk between these disciplines is limited, likely because the tasks and goals usually differ. The domain of e-learning and knowledge acquisition constitutes a fruitful intersection for the two fields' methodologies to be integrated because accurately tracking learning and forgetting over time and predicting future performance based on learning histories are central to developing effective, personalized learning tools. Here, we show how a state-of-the-art ML model can be enhanced by incorporating insights from a cognitive model of human memory. This was done by exploiting the predictive performance equation's (PPE) narrow but highly specialized domain knowledge with regard to the temporal dynamics of learning and forgetting. Specifically, the PPE was used to engineer timing-related input features for a gradient-boosted decision trees (GBDT) model. The resulting PPE-enhanced GBDT outperformed the default GBDT, especially under conditions in which limited data were available for training. Results suggest that integrating cognitive and ML models could be particularly productive if the available data are too high-dimensional to be explained by a cognitive model but not sufficiently large to effectively train a modern ML algorithm. Here, the cognitive model's insights pertaining to only one aspect of the data were enough to jump-start the ML model's ability to make predictions-a finding that holds promise for future explorations.
更多查看译文
关键词
Cognitive model, Machine learning, Prediction, Memory, Learning, Gradient boosting
AI 理解论文
溯源树
样例
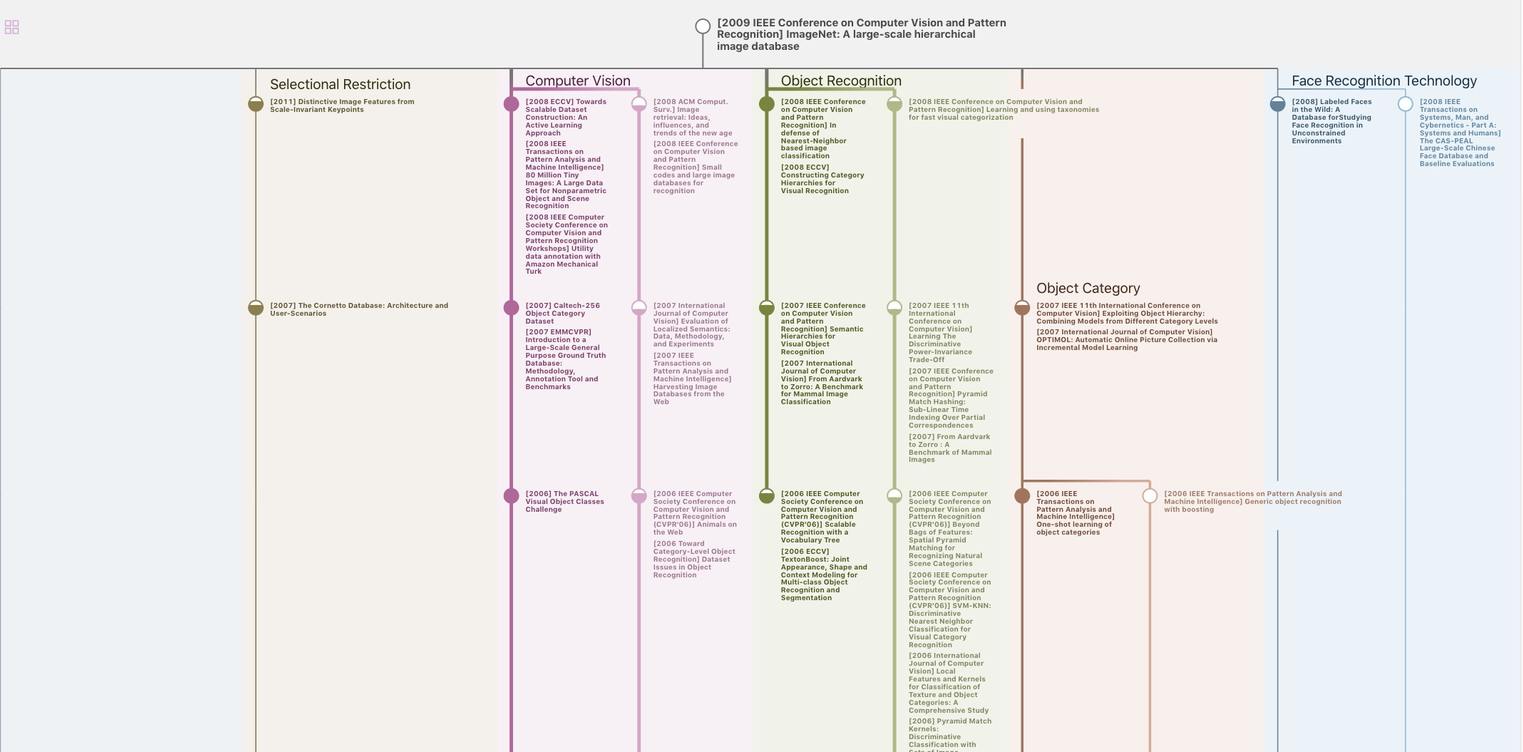
生成溯源树,研究论文发展脉络
Chat Paper
正在生成论文摘要