Evaluating the Impact of Semantic Segmentation and Pose Estimation on Dense Semantic SLAM
2021 IEEE/RSJ INTERNATIONAL CONFERENCE ON INTELLIGENT ROBOTS AND SYSTEMS (IROS)(2021)
摘要
Recent Semantic SLAM methods combine classical geometry-based estimation with deep learning-based object detection or semantic segmentation. In this paper we evaluate the quality of semantic maps generated by state-of-the-art class- and instance-aware dense semantic SLAM algorithms whose codes are publicly available and explore the impacts both semantic segmentation and pose estimation have on the quality of semantic maps. We obtain these results by providing algorithms with ground-truth pose and/or semantic segmentation data available from simulated environments. We establish that semantic segmentation is the largest source of error through our experiments, dropping mAP and OMQ performance by up to 74.3% and 71.3% respectively.
更多查看译文
关键词
semantic segmentation,pose estimation,dense Semantic,Recent Semantic SLAM methods,classical geometry-based estimation,deep learning-based object detection,semantic maps
AI 理解论文
溯源树
样例
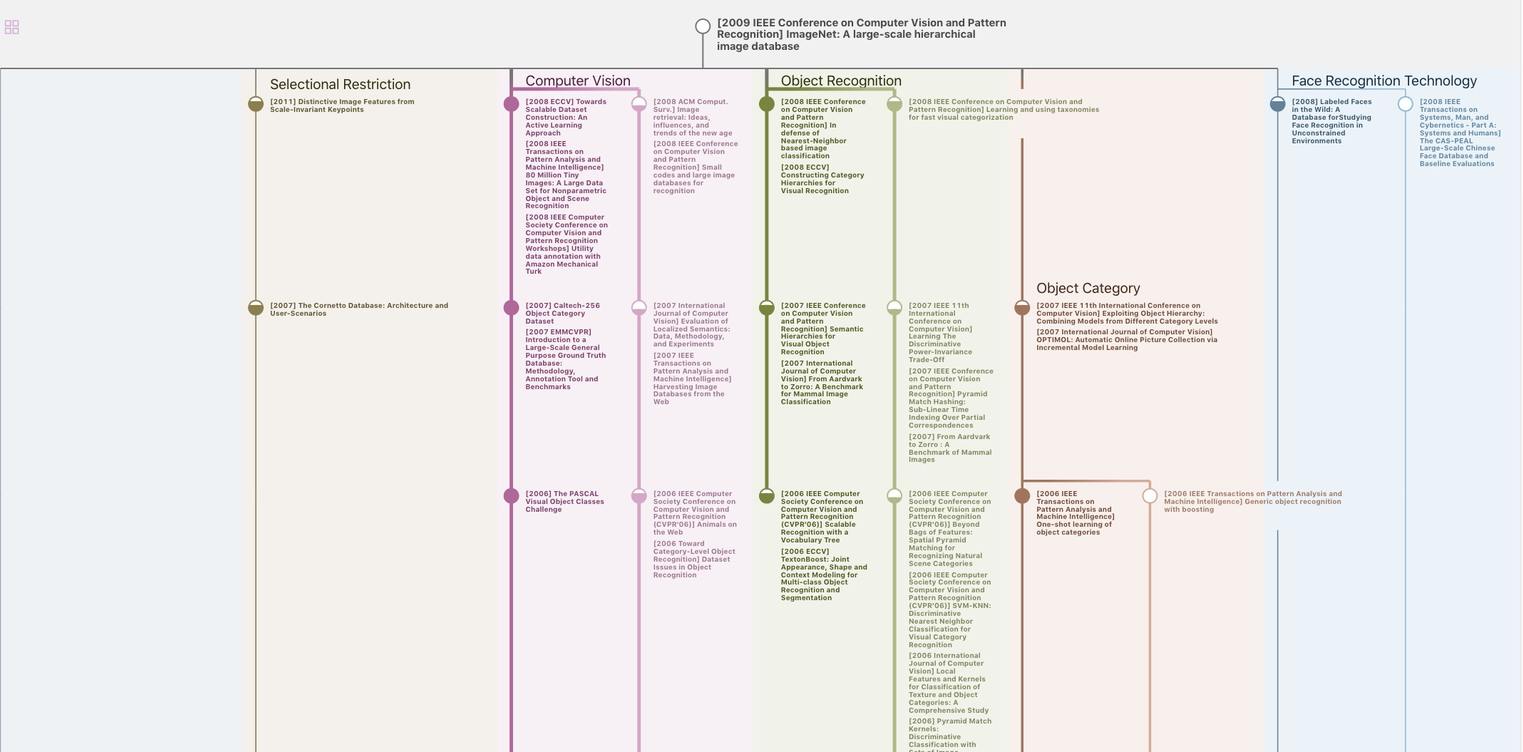
生成溯源树,研究论文发展脉络
Chat Paper
正在生成论文摘要