CONTAINER: Few-Shot Named Entity Recognition via Contrastive Learning
PROCEEDINGS OF THE 60TH ANNUAL MEETING OF THE ASSOCIATION FOR COMPUTATIONAL LINGUISTICS (ACL 2022), VOL 1: (LONG PAPERS)(2022)
摘要
Named Entity Recognition (NER) in Few-Shot setting is imperative for entity tagging in low resource domains. Existing approaches only learn class-specific semantic features and intermediate representations from source domains. This affects generalizability to unseen target domains, resulting in suboptimal performances. To this end, we present CONTAINER, a novel contrastive learning technique that optimizes the inter-token distribution distance for Few-Shot NER. Instead of optimizing class-specific attributes, CONTAINER optimizes a generalized objective of differentiating between token categories based on their Gaussian-distributed embeddings. This effectively alleviates overfitting issues originating from training domains. Our experiments in several traditional test domains (OntoNotes, CoNLL'03, WNUT '17, GUM) and a new large scale Few-Shot NER dataset (Few-NERD) demonstrate that, on average, CONTAINER outperforms previous methods by 3%-13% absolute F1 points while showing consistent performance trends, even in challenging scenarios where previous approaches could not achieve appreciable performance. The source code of CONTAINER will be available at: https://github.com/psunlpgroup/CONTaiNER.
更多查看译文
关键词
learning,few-shot
AI 理解论文
溯源树
样例
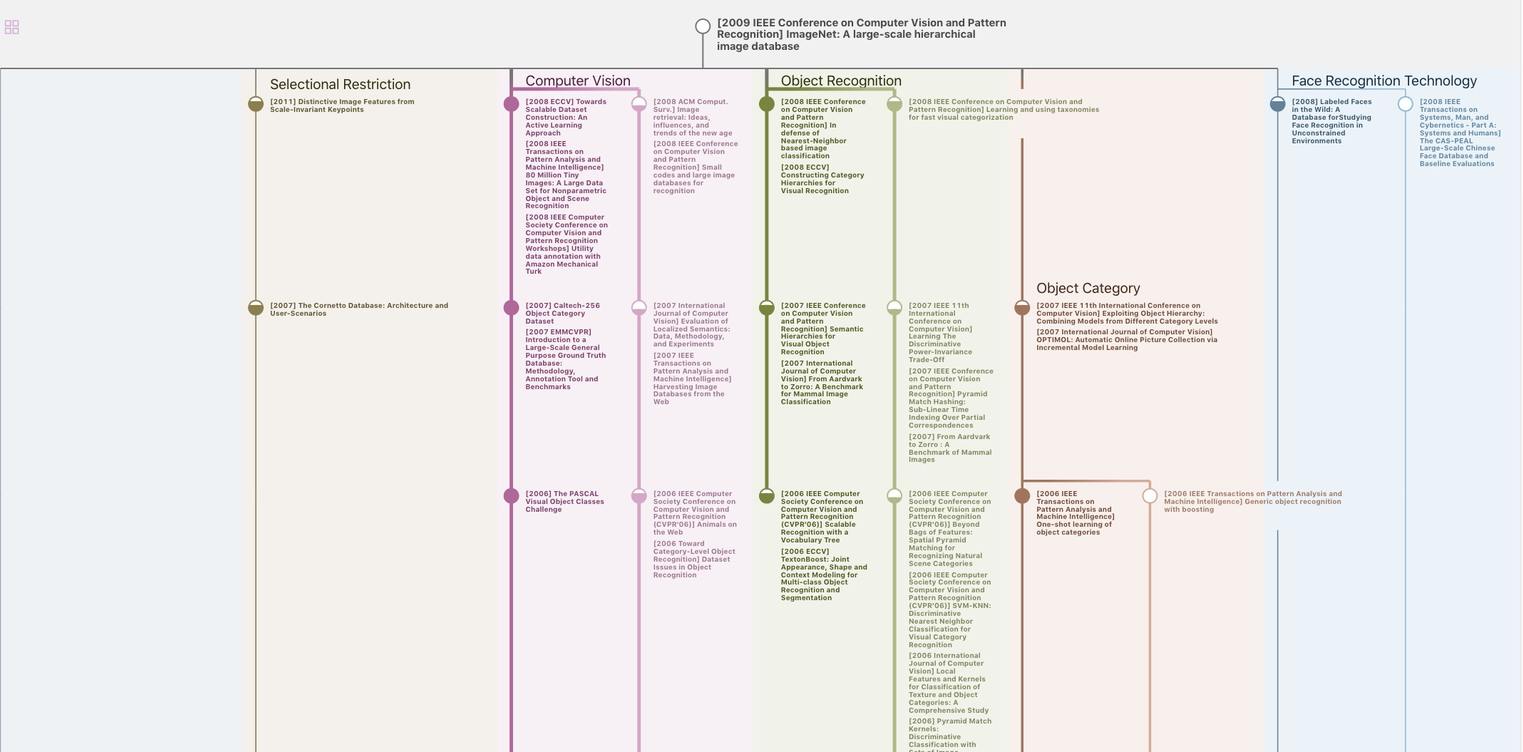
生成溯源树,研究论文发展脉络
Chat Paper
正在生成论文摘要