A Scoring Model Assisted by Frequency for Multi-Document Summarization
ARTIFICIAL NEURAL NETWORKS AND MACHINE LEARNING, ICANN 2021, PT V(2021)
摘要
While position information plays a significant role in sentence scoring of single document summarization, the repetition of content among different documents greatly impacts the salience scores of sentences in multi-document summarization. Introducing frequencies information can help identify important sentences which are generally ignored when only considering position information before. Therefore, in this paper, we propose a scoring model, SAFA (Self-Attention with Frequency Graph) which combines position information with frequency to identify the salience of sentences. The SAFA model constructs a frequency graph at the multi-document level based on the repetition of content of sentences, and assigns initial score values to each sentence based on the graph. The model then uses the position-aware gold scores to train a self-attention mechanism, obtaining the sentence significance at its single document level. The score of each sentence is updated by combing position and frequency information together. We train and test the SAFA model on the large-scale multi-document dataset Multi-News. The extensive experimental results show that the model incorporating frequency information in sentence scoring outperforms the other state-of-the-art extractive models.
更多查看译文
关键词
Multiple document summarization, Position information, Frequency, Graph
AI 理解论文
溯源树
样例
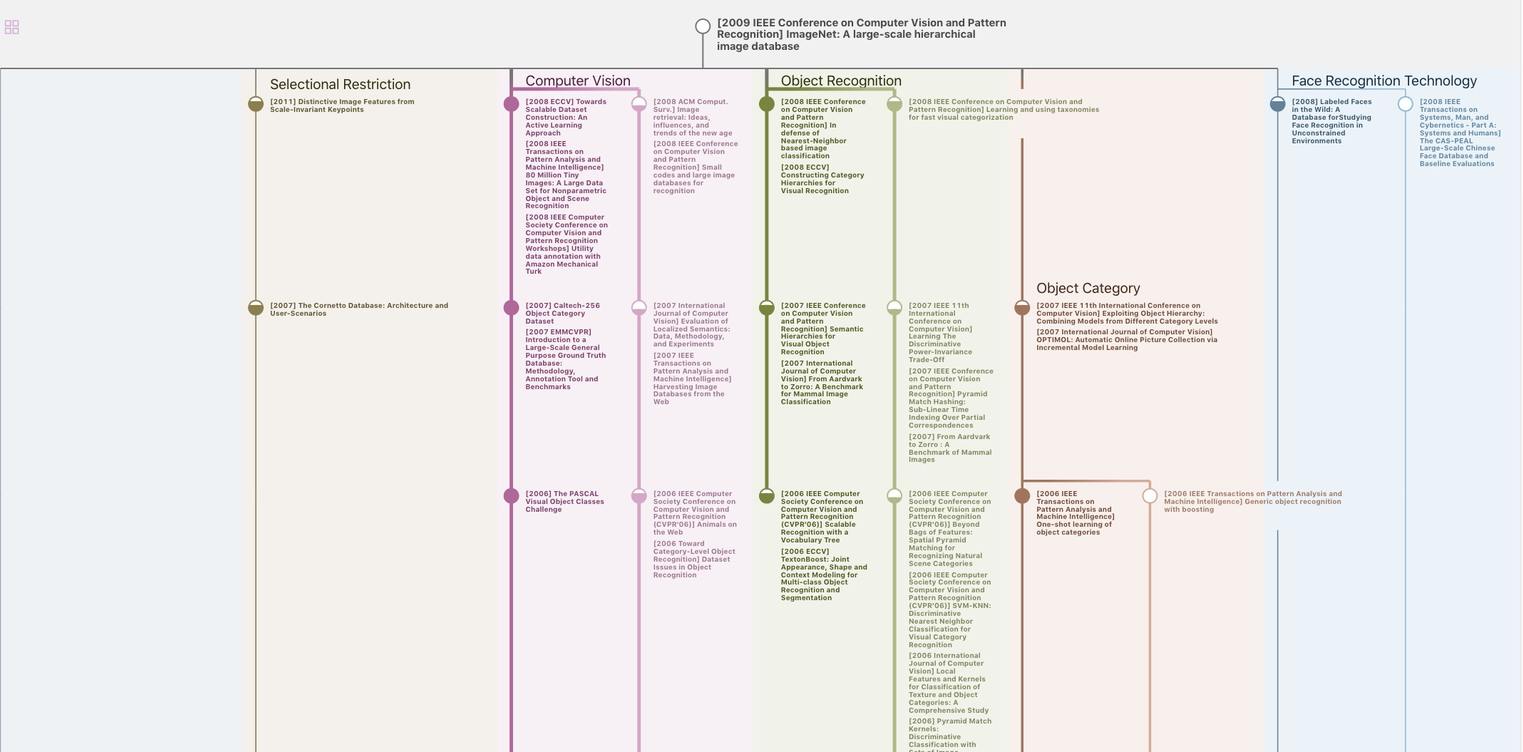
生成溯源树,研究论文发展脉络
Chat Paper
正在生成论文摘要