A Strategy for Referential Problem in Low-Resource Neural Machine Translation
ARTIFICIAL NEURAL NETWORKS AND MACHINE LEARNING, ICANN 2021, PT V(2021)
摘要
This paper aims to solve a series of referential problems in sequence decoding, which caused by corpus sparsity in low-resource Neural Machine Translation (NMT), including pronoun missing, reference error, gender bias. It is difficult to find the essential reason of these problems because they are only shown in the prediction results and all aspects of the model training. Different from the usual solutions based on complex mathematical rule setting and adding artificial features, we expect to turn the problems in the predictions into noise as much as possible. On this basis, we further use adversarial training to make the model find the balance between the noise and the golden samples, instead of exploring the reason of the problem during the complex training. In this paper, a noise-based preprocessing operation and a slight modification of the adversarial training can help the model to better generalize a series of referential problems in low-resource NMT tasks. Experiments show that the evaluation of BLEU score and the accuracy of pronouns in sequence on Korean-Chinese, Mongolian-Chinese and Arabic-Chinese task have been significantly improved.
更多查看译文
关键词
Low-Resource Neural Machine Translation, Referential problem, Generative Adversarial Network
AI 理解论文
溯源树
样例
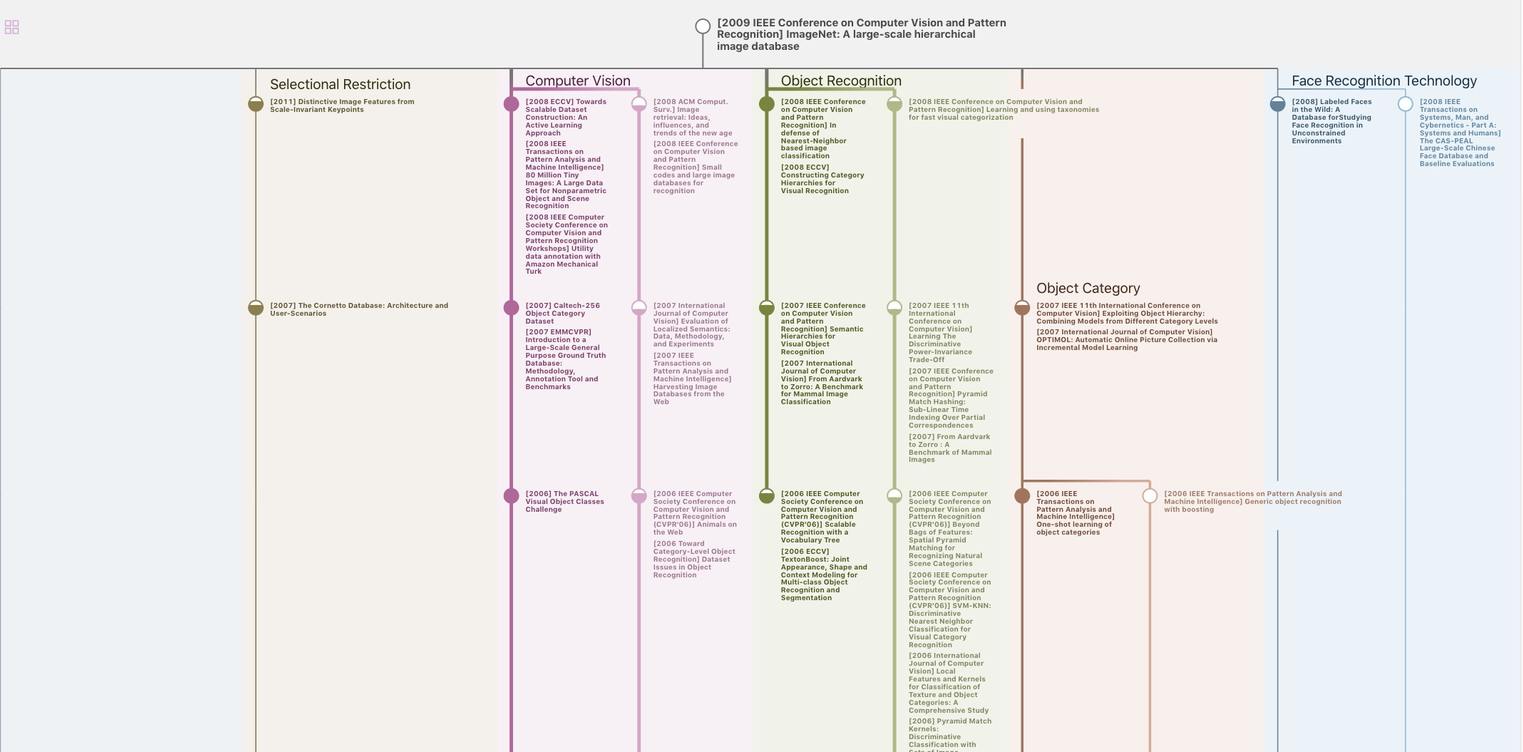
生成溯源树,研究论文发展脉络
Chat Paper
正在生成论文摘要