Self-supervised Multi-view Clustering for Unsupervised Image Segmentation
ARTIFICIAL NEURAL NETWORKS AND MACHINE LEARNING, ICANN 2021, PT V(2021)
摘要
At present, the main idea of CNN-based unsupervised image segmentation is clustering a single image in the framework of CNNs. However, the single image clustering is very difficult to obtain enough supervision information for network learning. For solving this problem, we propose a Self-supervised Multi-view Clustering (SMC) structure for unsupervised image segmentation to mine additional supervised information. Based on the observation that the predicted pixel-level labels and the input images have the same spatial features, the multi-view images acquired by data augmentation are clustered to obtain the multi-view results and the proposed SMC uses the differences among these results to learn self-supervised information. Moreover, a Hybrid Self-supervised (HS) loss is proposed to make full use of the self-supervised information for further improving the prediction accuracy and the convergence speed. Extensive experiments in BSD500 and PASCAL VOC 2012 datasets demonstrate the superiority of our proposed approach.
更多查看译文
关键词
Unsupervised image segmentation, Convolutional neural network, Self-supervised Multi-view Clustering
AI 理解论文
溯源树
样例
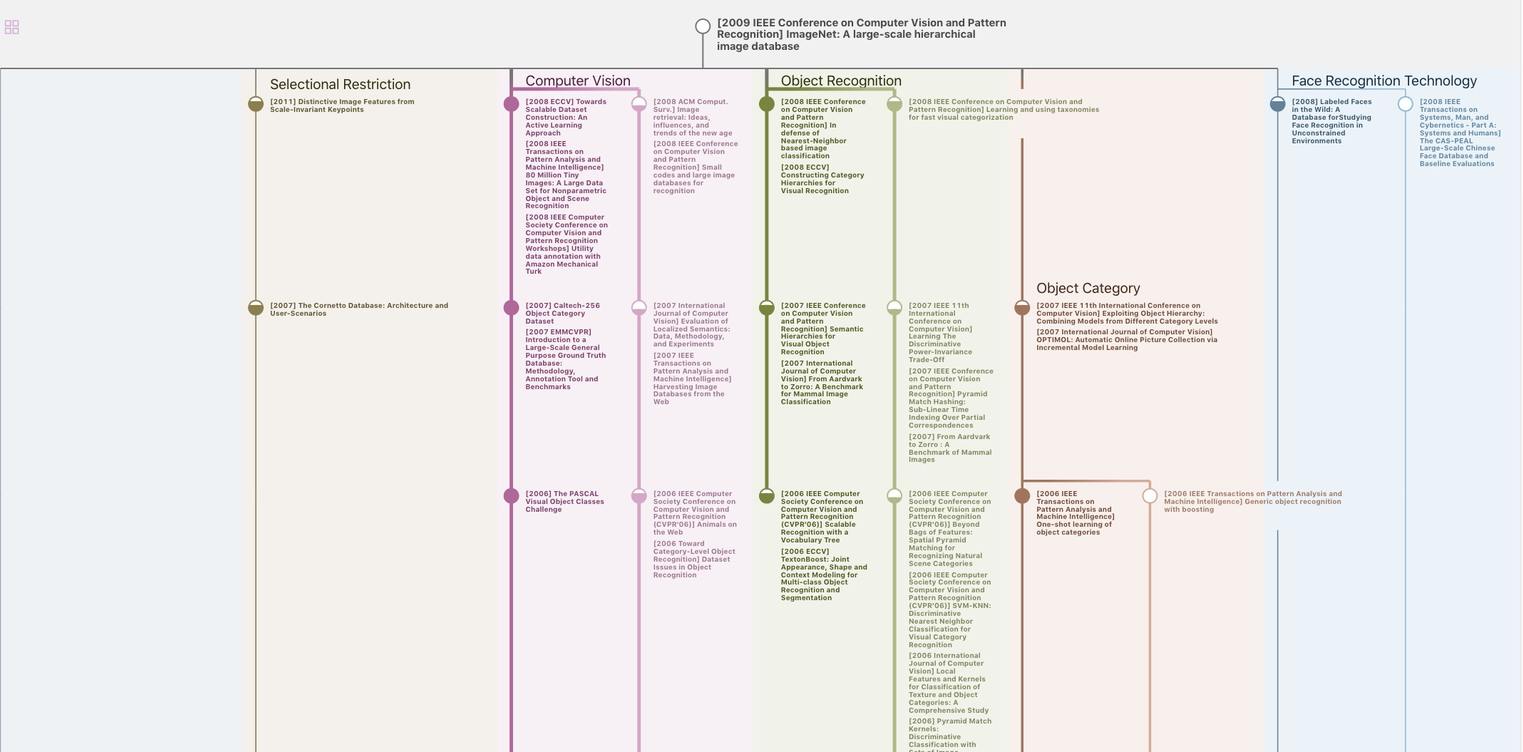
生成溯源树,研究论文发展脉络
Chat Paper
正在生成论文摘要