One-Shot Meta-learning for Radar-Based Gesture Sequences Recognition
ARTIFICIAL NEURAL NETWORKS AND MACHINE LEARNING - ICANN 2021, PT II(2021)
摘要
Radar-based gesture recognition constitutes an intuitive way for enhancing human-computer interaction (HCI). However, training algorithms for HCI capable of adapting to gesture recognition often require a large dataset with many task examples. In this work, we propose for the first time on radar sensed hand-poses, the use of optimization-based meta-techniques applied on a convolutional neural network (CNN) to distinguish 16 gesture sequences with only one sample per class (shot) in 2-ways, 4-ways and 5-ways experiments. We make use of a frequency-modulated continuous-wave (FMCW) 60 GHz radar to capture the sequences of four basic hand gestures, which are processed and stacked in the form of temporal projections of the radar range information (Range-Time Map - RTM). The experimental results demonstrate how the use of optimization-based meta-techniques leads to an accuracy greater than 94% in a 5-ways 1-shot classification problem, even on sequences containing a type of basic gesture never observed in the training phase. Additionally, thanks to the generalization capabilities of the proposed approach, the required training time on new sequences is reduced by a factor of 8,000 in comparison to a typical deep CNN.
更多查看译文
关键词
Gesture recognition, Meta learning, Millimeter wave radar
AI 理解论文
溯源树
样例
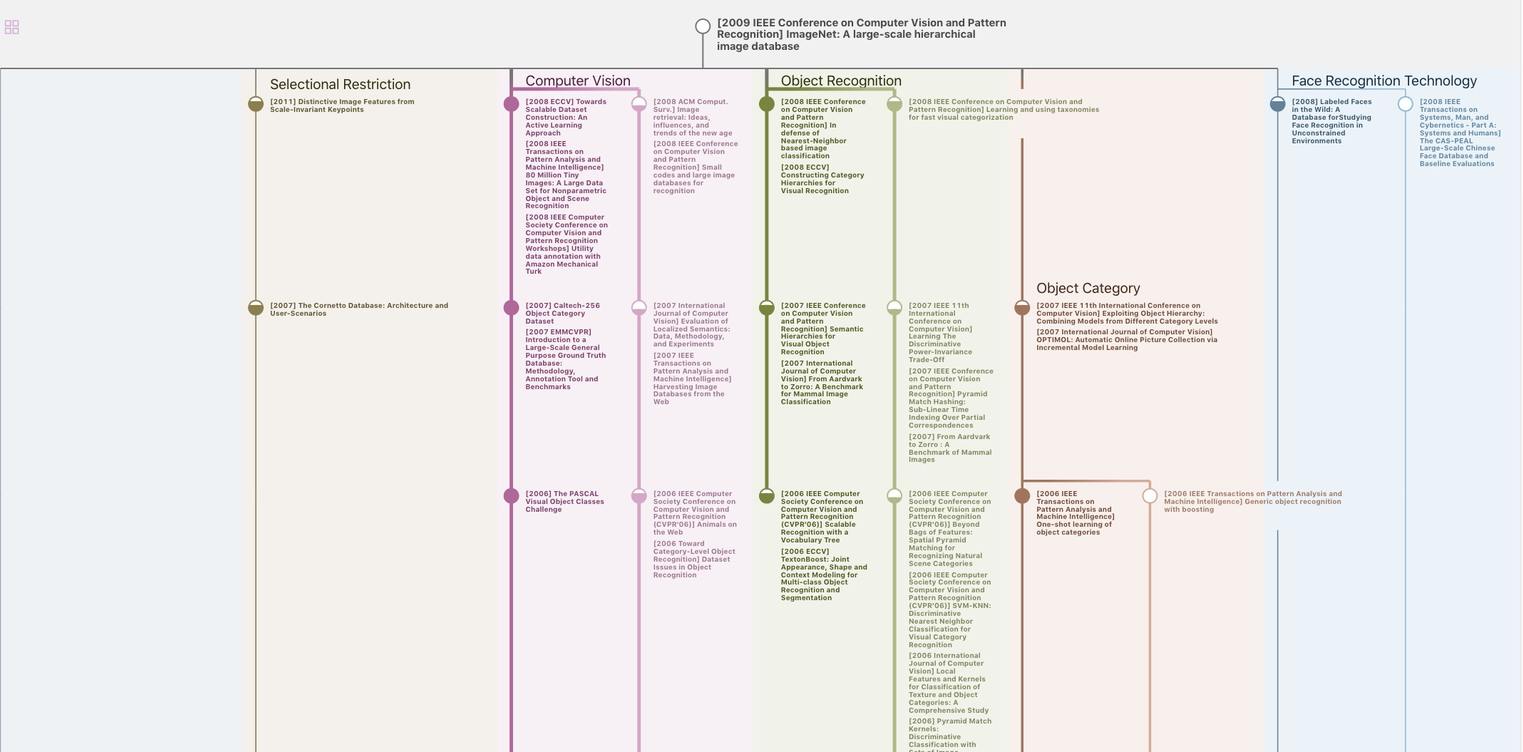
生成溯源树,研究论文发展脉络
Chat Paper
正在生成论文摘要