KCNet: Kernel-Based Canonicalization Network for Entities in Recruitment Domain
ARTIFICIAL NEURAL NETWORKS AND MACHINE LEARNING - ICANN 2021, PT II(2021)
摘要
Online recruitment platforms have abundant user-generated content in the form of job postings, candidate, and company profiles. This content when ingested into Knowledge bases causes redundant, ambiguous, and noisy entities. These multiple (non-standardized) representation of the entities deteriorates the performance of downstream tasks such as job recommender systems, search systems, and question answering. Therefore, making it imperative to canonicalize the entities to improve the performance of such tasks. Recent research discusses either statistical similarity measures or deep learning methods like word-embedding or siamese network-based representations for canonicalization. In this paper, we propose a Kernel-based Canonicalization Network (KCNet) that outperforms all the known statistical and deep learning methods. We also show that the use of side information such as industry type, url of websites, etc. further enhances the performance of the proposed method. Our experiments on 351,600 entities (companies, institutes, skills, and designations) from a popular online recruitment platform demonstrate that the proposed method improves the overall F1-score by 23% compared to the previous baselines, which results in coherent clusters of unique entities.
更多查看译文
关键词
Entity canonicalization, Recruitment domain, Entity normalization
AI 理解论文
溯源树
样例
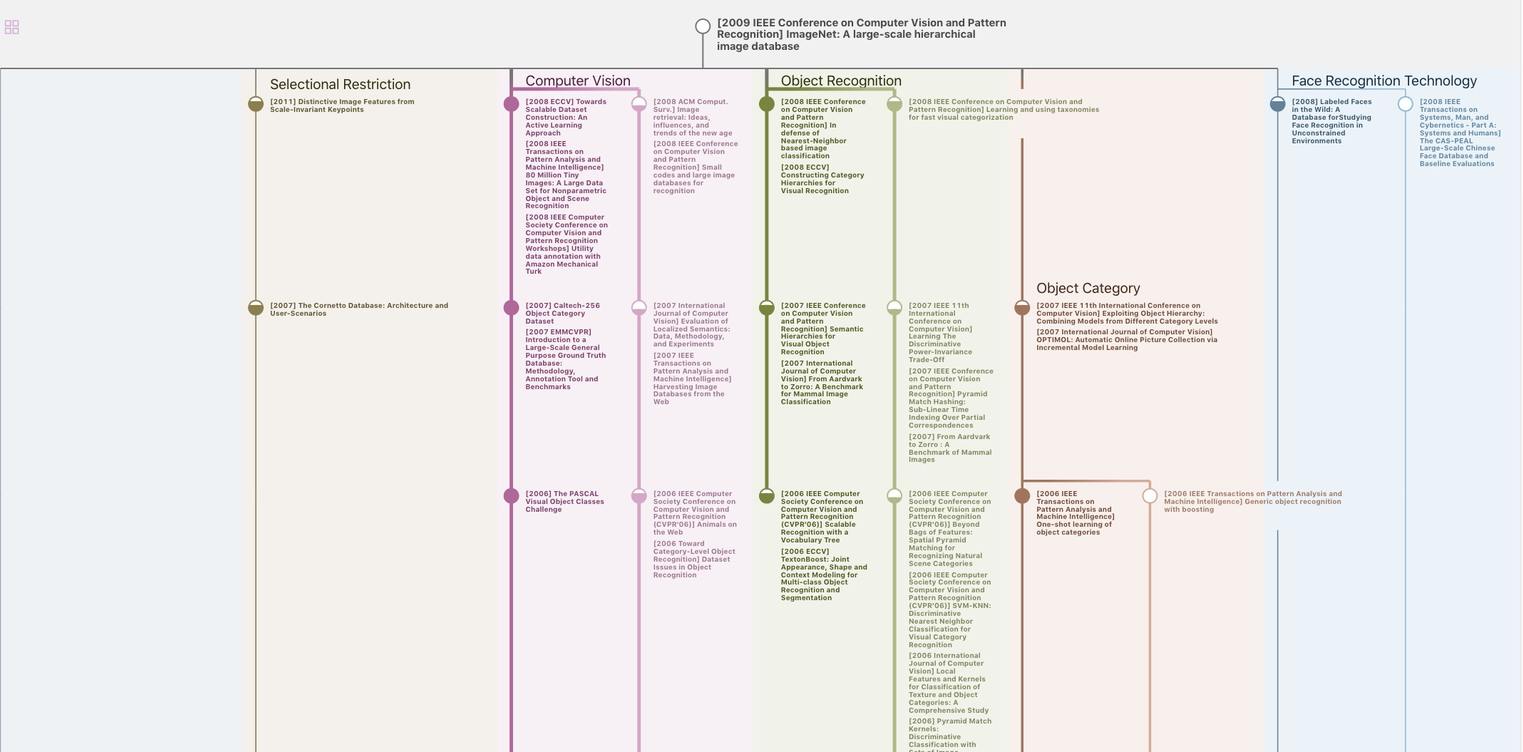
生成溯源树,研究论文发展脉络
Chat Paper
正在生成论文摘要