Attention-Based Multi-view Feature Fusion for Cross-Domain Recommendation
ARTIFICIAL NEURAL NETWORKS AND MACHINE LEARNING - ICANN 2021, PT I(2021)
摘要
Cross-domain recommendation can effectively alleviate the data sparsity problem in recommender systems. Existing methods for cross-domain recommendation can be roughly divided into two categories: specific-feature-based methods and sharing-feature-based methods. Specific-feature-based methods focus on learning users' and items' domain-specific features in each domain and transferring the same or similar features across domains for recommendation. Sharing-feature-based methods concentrate on obtaining latent transferable features cross domains and utilizing them for recommendation. However, both methods have defects: 1) specific-feature-based methods fail to capture latent transferable features cross domains, which greatly reduces the availability of source domain information; 2) sharing-feature-based methods ignore users' and items' domain-specific features so that the recommended items of different domains have high similarity. Since user preferences are distinct in different domains, the recommended items may be unsuitable for users. To overcome the above problems, we propose an Attention-based Multi-View Feature fusion model (AMVF). To improve the availability of source domain information and recommend suitable items, AMVF learns latent transferable features and domain-specific features simultaneously. Since various features make distinct contributions to recommendation, seamlessly fusing different features is a challenge. Therefore, an attention-based feature fusion algorithm is designed to learn different importance of various features. To demonstrate the effectiveness of AMVF, extensive experiments are conducted on two pairs cross-domain datasets. The results empirically verify the superior performance of AMVF.
更多查看译文
关键词
Cross-domain recommendation, Domain-specific feature, Latent transferable feature, Attention-based feature fusion
AI 理解论文
溯源树
样例
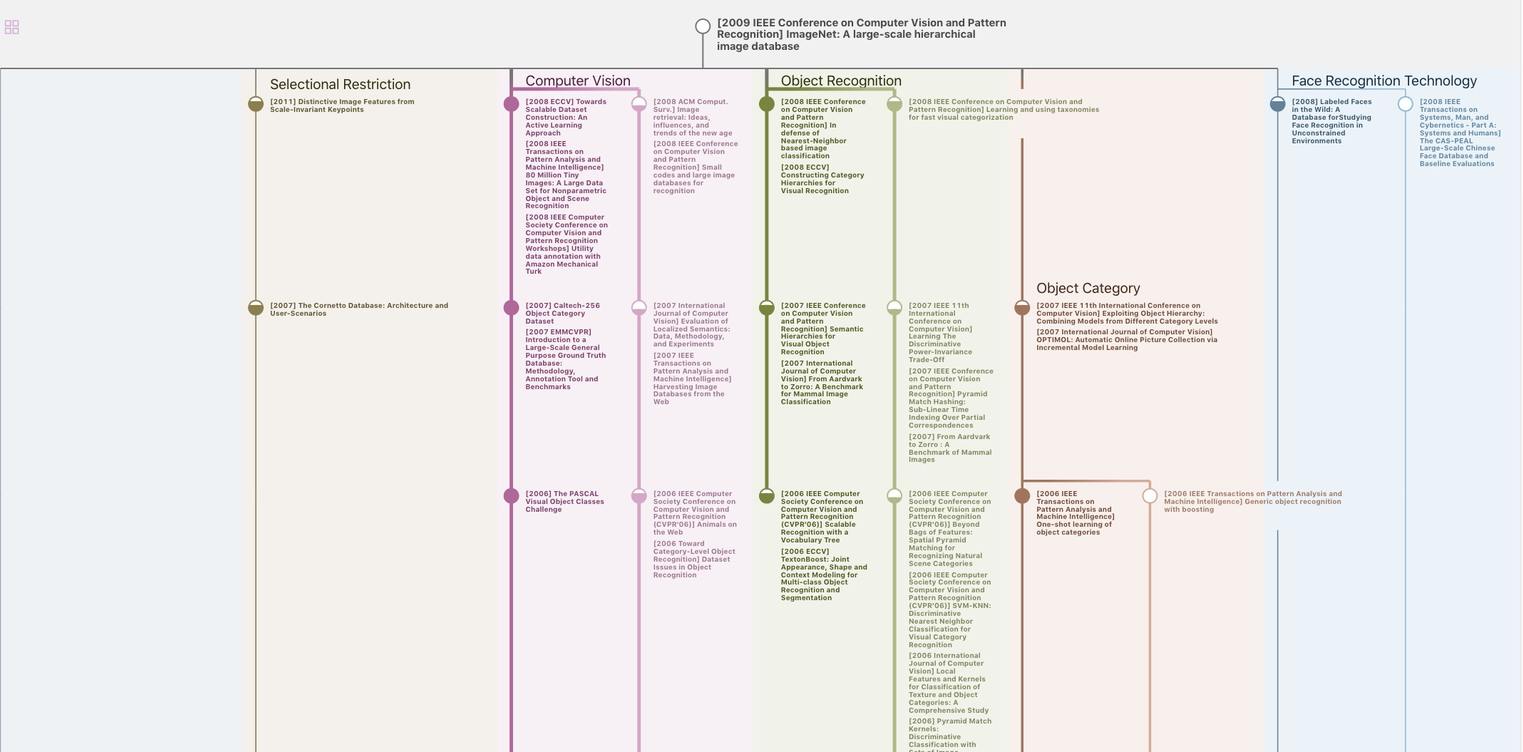
生成溯源树,研究论文发展脉络
Chat Paper
正在生成论文摘要