Independent Deep Deterministic Policy Gradient Reinforcement Learning in Cooperative Multiagent Pursuit Games
ARTIFICIAL NEURAL NETWORKS AND MACHINE LEARNING - ICANN 2021, PT IV(2021)
摘要
In this paper, we study a fully decentralized multi-agent pursuit problem in a non-communication environment. Fully decentralized (decentralized training and decentralized execution) has stronger robustness and scalability compared with centralized training and decentralized execution (CTDE), which is the current popular multi-agent reinforcement learning method. Both centralized training and communication mechanism require a large amount of information exchange between agents, which are strong assumptions that are difficult to meet in reality. However, traditional fully decentralized multi-agent reinforcement learning methods (e.g., IQL) are difficult to converge stably due to the dynamic changes of other agents' strategies. Therefore, we extend actor-critic to actor-critic-N framework, and propose Potential-Field-Guided Deep Deterministic Policy Gradient (PGDDPG) method on this basis. The agent uses the unified artificial potential field to guide the agent's strategy updating, which reduces the uncertainty of multi-agent's decision making in the complex and dynamic changing environment. Thus, PGDDPG which we proposed can converge fast and stably. Finally, through the pursuit experiments in MPE and CARLA, we prove that our method achieves higher success rate and more stable performance than DDPG and MADDPG.
更多查看译文
关键词
Reinforcement learning, Actor-critic, Potential field, Planning and learning, Predator-prey
AI 理解论文
溯源树
样例
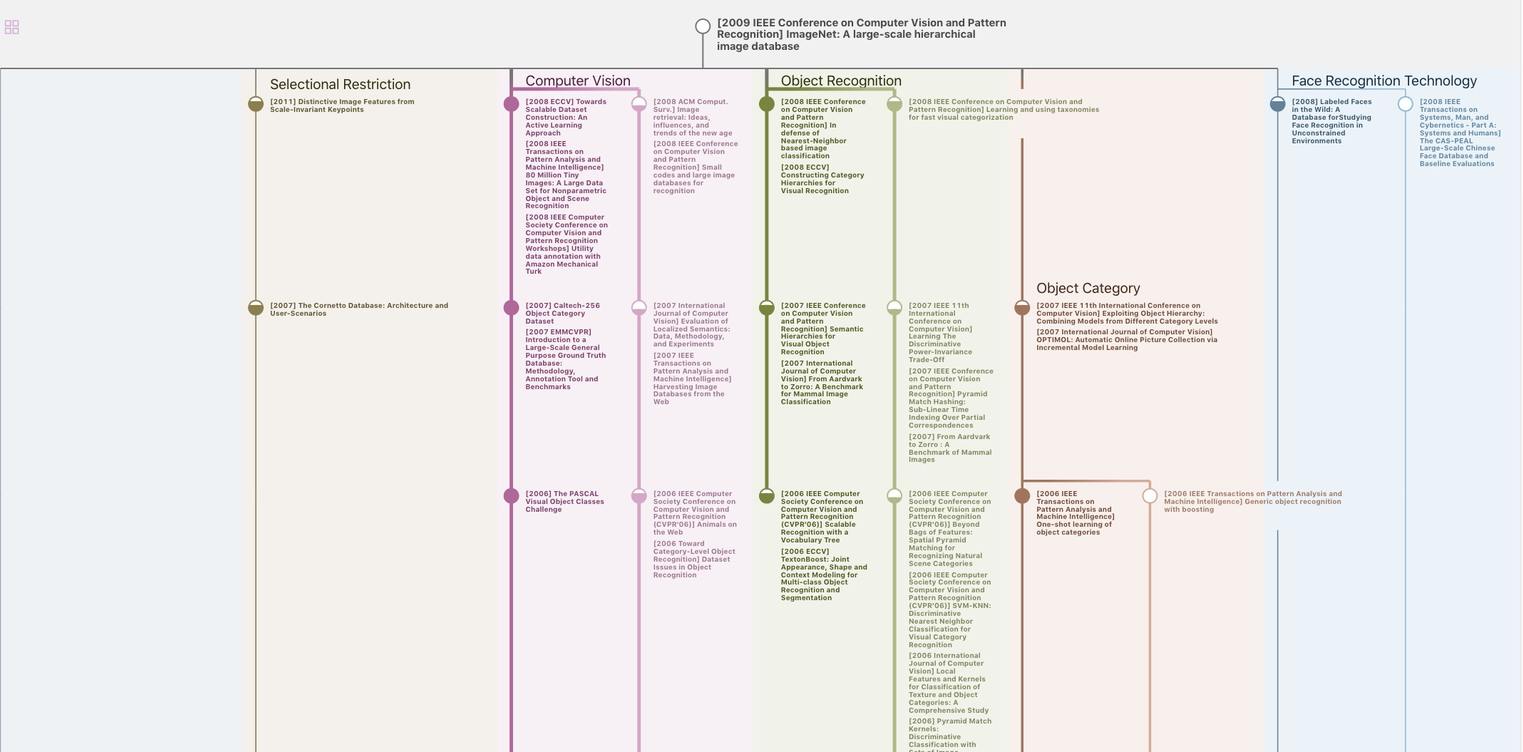
生成溯源树,研究论文发展脉络
Chat Paper
正在生成论文摘要